Wasserstein Fair Autoencoders
ICLR 2023(2023)
摘要
Autoencoders, or nonlinear factor models parameterized by neural networks, have become an indispensable tool for generative modeling and representation learning in high dimensions. Imposing structural constraints such as conditional independence on the latent variables (representation, or factors) in order to capture invariance or fairness with autoencoders has been attempted through adding ad hoc penalties to the loss function mostly in the variational autoencoder (VAE) context, often based on heuristic arguments. In this paper, we demonstrate that Wasserstein autoencoders (WAEs) are highly flexible in embracing structural constraints. Well-known extensions of VAEs for this purpose are gracefully handled within the framework of the seminal result by Tolstikhin et al. (2018). In particular, given a conditional independence structure of the generative model (decoder), corresponding encoder structure and penalties are induced from the functional constraints that define the WAE. This property of WAEs opens up a principled way of penalizing autoencoders to impose structural constraints. Utilizing this generative model structure, we present results on fair representation and conditional generation tasks, and compare them with other preceding methods.
更多查看译文
关键词
conditional generation,fair representation,disentanglement,wasserstein autoencoder
AI 理解论文
溯源树
样例
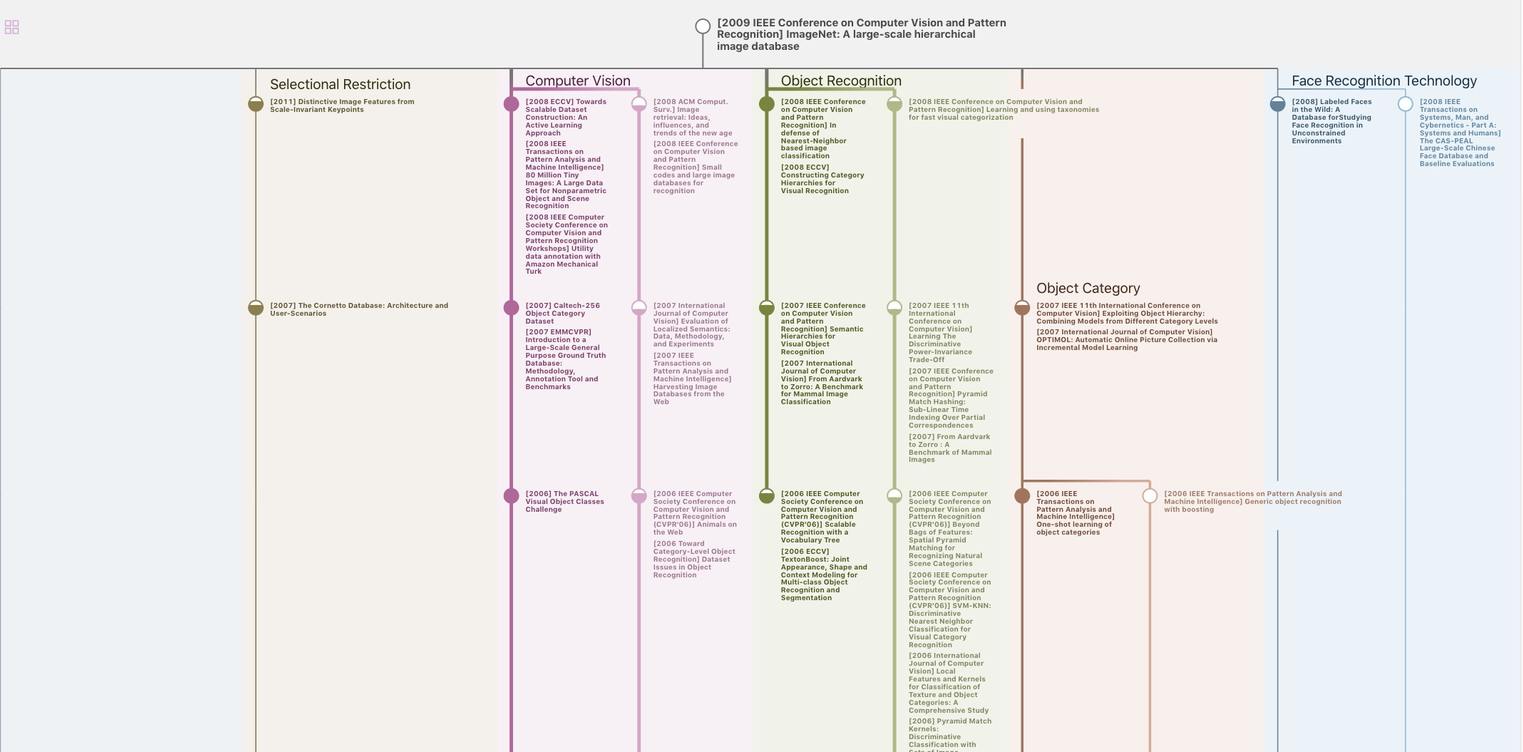
生成溯源树,研究论文发展脉络
Chat Paper
正在生成论文摘要