DECN: Evolution Inspired Deep Convolution Network for Black-box Optimization
ICLR 2023(2023)
摘要
We design a deep evolutionary convolution network (DECN) for continuous black-box optimization to force the random population to move near the optimal solution, which is the goal of population-based optimization, such as evolutionary algorithm and evolutionary strategy. DECN is composed of two modules: convolution-based reasoning module (CRM) and selection module (SM), to move from hand-designed searching strategies to learned searching strategies in population-based optimization. CRM produces a population that is closer to the optimal solution based on the convolution operators, and SM removes poor solutions. We also design a proper loss function to support the training of DECN. The experimental results on unconstrained continuous optimization problems show that DECN can learn searching strategies and surpass population-based baselines. Moreover, DECN obtains good performance when transferred to optimization problems unseen during the training stage. In addition, DECN is friendly to the acceleration with Graphics Processing Units (GPUs) and runs 102 times faster than unaccelerated EA when evolving 32 populations, each containing 6400 individuals.
更多查看译文
关键词
Learning to Optimize,Black-box Optimization
AI 理解论文
溯源树
样例
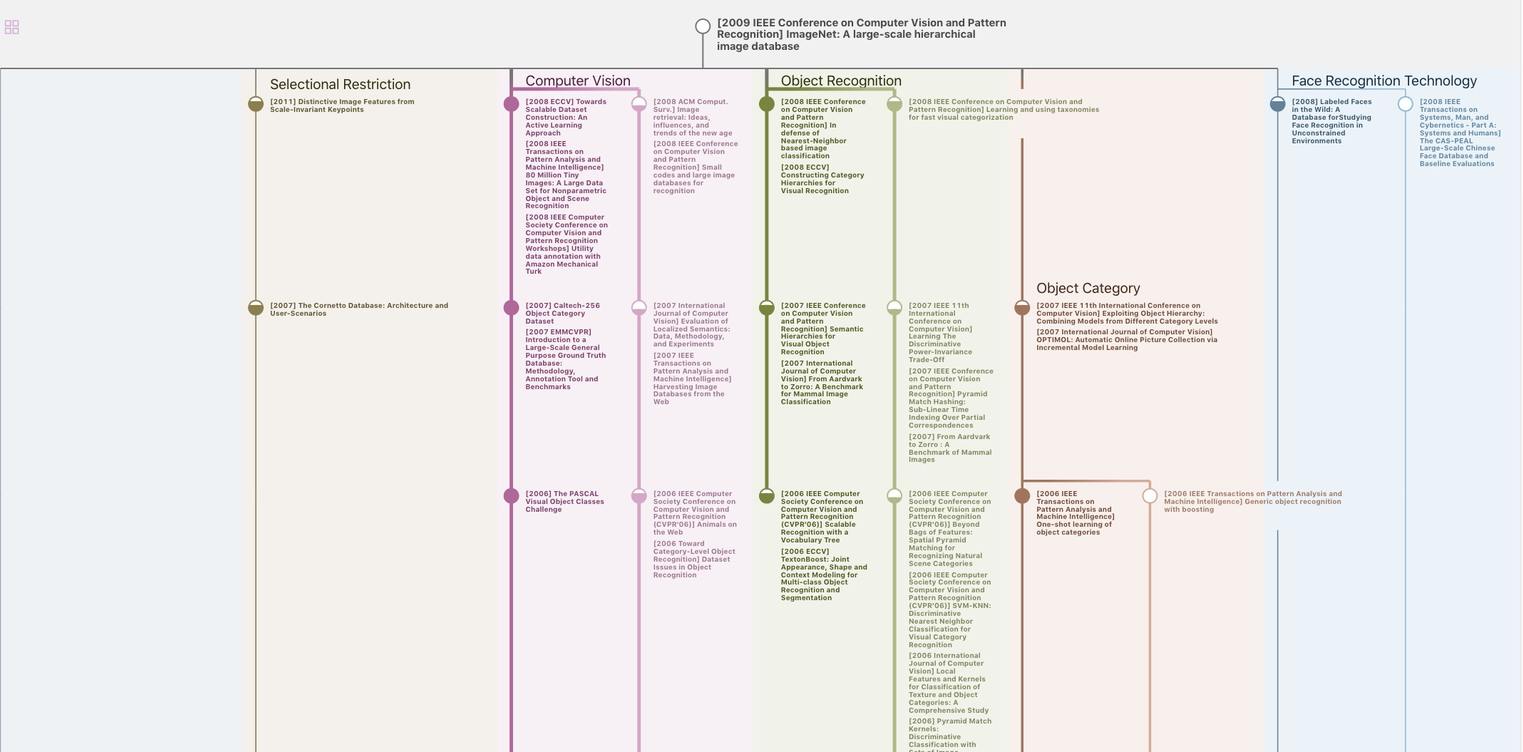
生成溯源树,研究论文发展脉络
Chat Paper
正在生成论文摘要