Backdoor or Feature? A New Perspective on Data Poisoning
ICLR 2023(2023)
摘要
In a backdoor attack, an adversary adds maliciously constructed ("backdoor") examples into a training set to make the resulting model
vulnerable to manipulation. Defending against such attacks---that is, finding and removing the backdoor examples---typically involves viewing these examples as outliers and using techniques from robust statistics to detect and remove them.
In this work, we present a new perspective on backdoor attacks. We argue that without structural information on the training data distribution, backdoor attacks are indistinguishable from naturally-occuring features in the data (and thus impossible to ``detect'' in a general sense). To circumvent this impossibility, we assume that a backdoor attack corresponds to the strongest feature in the training data. Under this assumption---which we make formal---we develop a new framework for detecting backdoor attacks. Our framework naturally gives rise to a corresponding algorithm whose efficacy we show both theoretically and experimentally.
更多查看译文
AI 理解论文
溯源树
样例
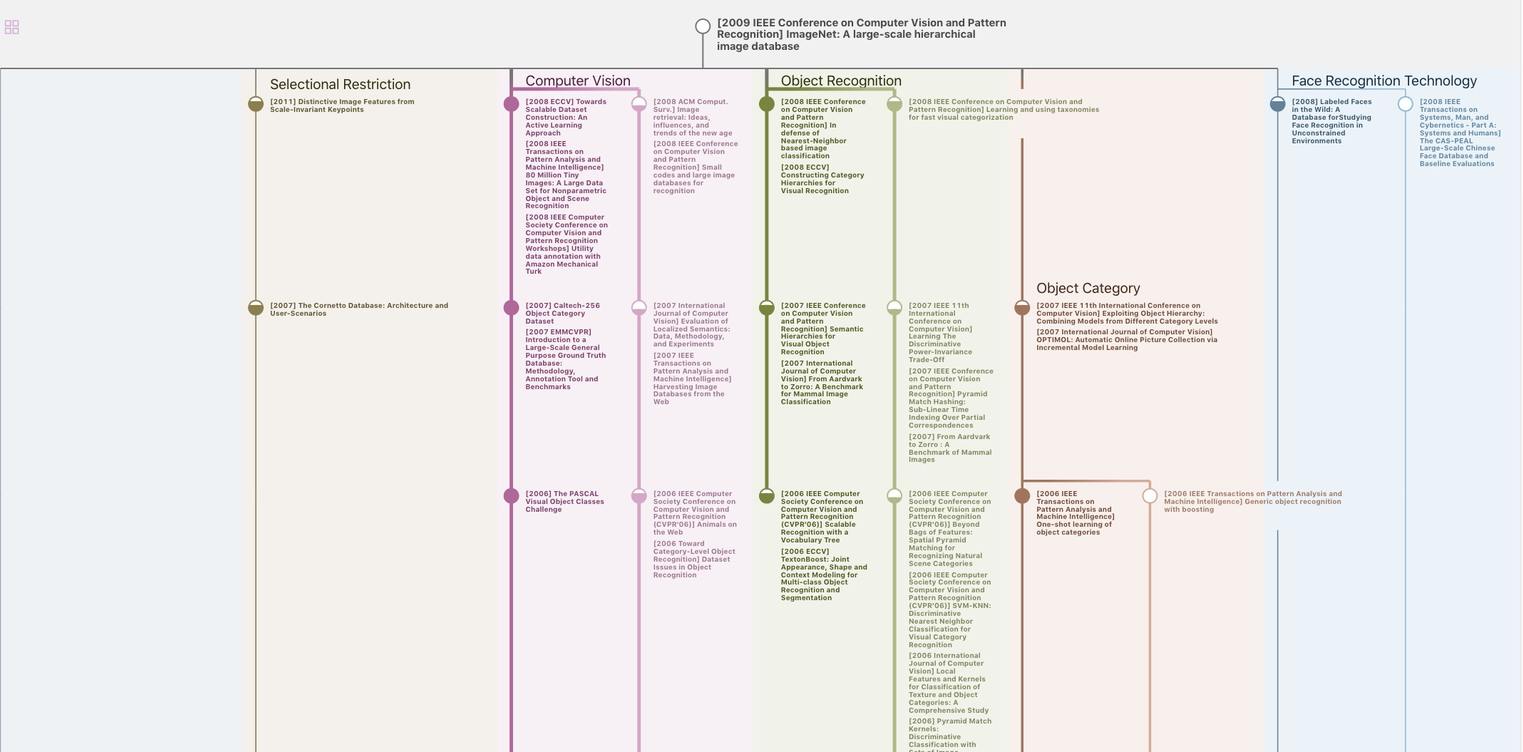
生成溯源树,研究论文发展脉络
Chat Paper
正在生成论文摘要