Structural Adversarial Objectives for Self-Supervised Representation Learning
ICLR 2023(2023)
摘要
Within the framework of generative adversarial networks (GANs), we propose objectives that task the discriminator with additional structural modeling responsibilities. In combination with an efficient smoothness regularizer imposed on the network, these objectives guide the discriminator to learn to extract informative representations, while maintaining a generator capable of sampling from the domain. Specifically, we influence the features produced by the discriminator at two levels of granularity. At coarse scale, we impose a Gaussian assumption encouraging smoothness and diversified representation, while at finer scale, we group features forming local clusters. Experiments demonstrate that augmenting GANs with these self-supervised objectives suffices to produce discriminators which, evaluated in terms of representation learning, compete with networks trained by state-of-the-art contrastive approaches. Furthermore, operating within the GAN framework frees our system from the reliance on data augmentation schemes that is prevalent across purely contrastive representation learning methods.
更多查看译文
关键词
learning,representation
AI 理解论文
溯源树
样例
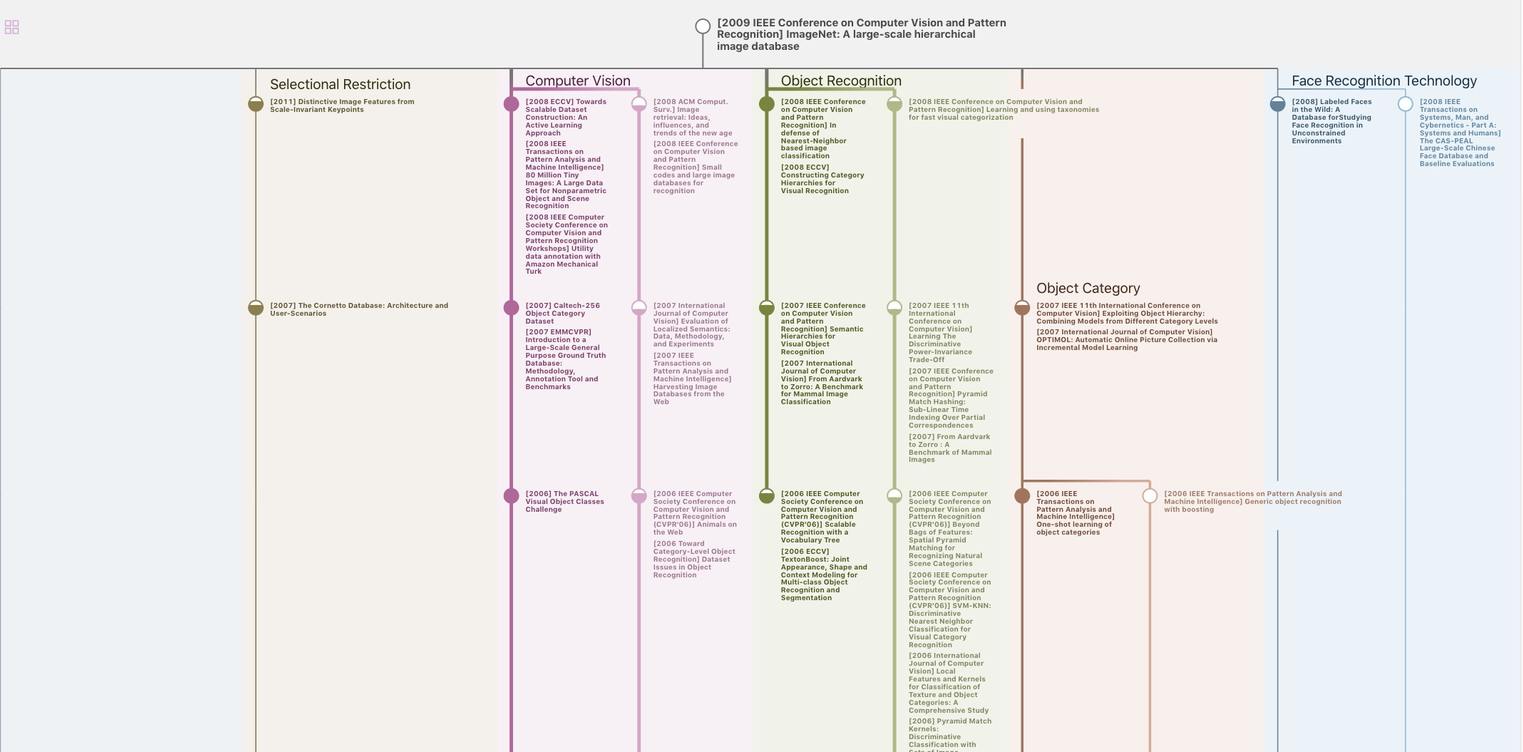
生成溯源树,研究论文发展脉络
Chat Paper
正在生成论文摘要