Learning from Labeled Images and Unlabeled Videos for Video Segmentation
ICLR 2023(2023)
摘要
Performance on video object segmentation still lags behind that of image segmentation due to a paucity of labeled videos. Annotations are time-consuming and laborious to collect, and may not be feasibly obtained in certain situations. However there is a growing amount of freely available unlabeled video data which has spurred interest in unsupervised video representation learning. In this work we focus on the setting in which there is no/little access to labeled videos for video object segmentation. To this end we leverage large-scale image segmentation datasets and adversarial learning to train 2D/3D networks for video object segmentation. We first motivate the treatment of images and videos as two separate domains by analyzing the performance gap of an image segmentation network trained on images and applied to videos. Through studies using several image and video segmentation datasets, we show how an adversarial loss placed at various locations within the network can make feature representations invariant to these domains and improve the performance when the network has access to only labeled images and unlabeled videos. To prevent the loss of discriminative semantic class information we apply our adversarial loss within clusters of features and show this boosts our method's performance within Transformer-based models.
更多查看译文
关键词
Video,Segmentation,Representation
AI 理解论文
溯源树
样例
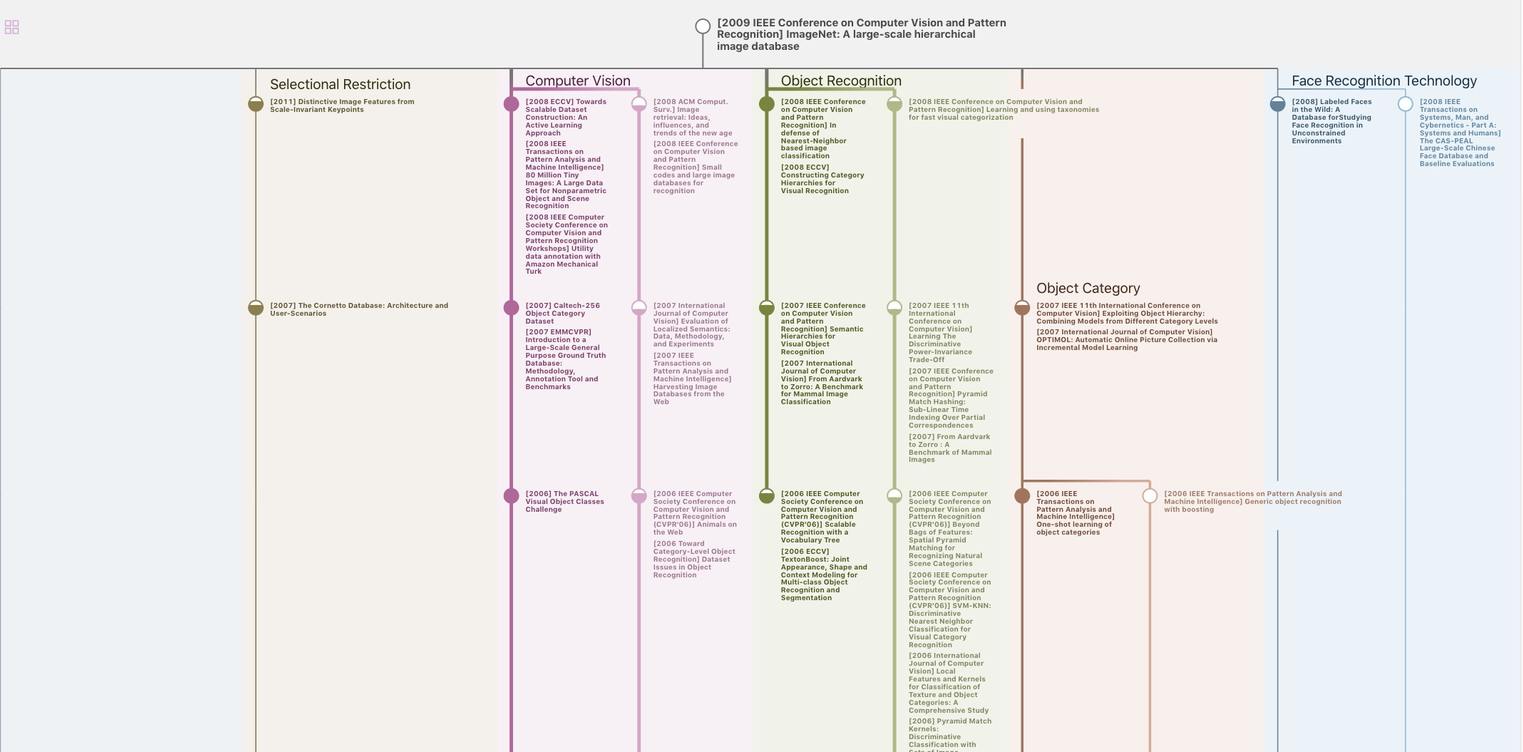
生成溯源树,研究论文发展脉络
Chat Paper
正在生成论文摘要