Pre-training Protein Structure Encoder via Siamese Diffusion Trajectory Prediction
ICLR 2023(2023)
Abstract
Due to the determining role of protein structures on diverse protein functions, pre-training representations of proteins on massive unlabeled protein structures has attracted rising research interests. Among recent efforts on this direction, mutual information (MI) maximization based methods have gained the superiority on various downstream benchmark tasks. The core of these methods is to design correlated views that share common information about a protein. Previous view designs focus on capturing structural motif co-occurrence on the same protein structure, while they cannot capture detailed atom/residue interactions. To address this limitation, we propose the Siamese Diffusion Trajectory Prediction (SiamDiff) method. SiamDiff builds a view as the trajectory that gradually approaches protein native structure from scratch, which facilitates the modeling of atom/residue interactions underlying the protein structural dynamics. Specifically, we employ the multimodal diffusion process as a faithful simulation of the structure-sequence co-diffusion trajectory, where rich patterns of protein structural changes are embedded. On such basis, we design a principled theoretical framework to maximize the MI between correlated multimodal diffusion trajectories. We study the effectiveness of SiamDiff on both residue-level and atom-level structures. On the EC and ATOM3D benchmarks, we extensively compare our method with previous protein structure pre-training approaches. The experimental results verify the consistently superior or competitive performance of SiamDiff on all benchmark tasks compared to existing baselines. The source code will be made public upon acceptance.
MoreTranslated text
Key words
Protein representation learning,diffusion models,self-supervised learning
AI Read Science
Must-Reading Tree
Example
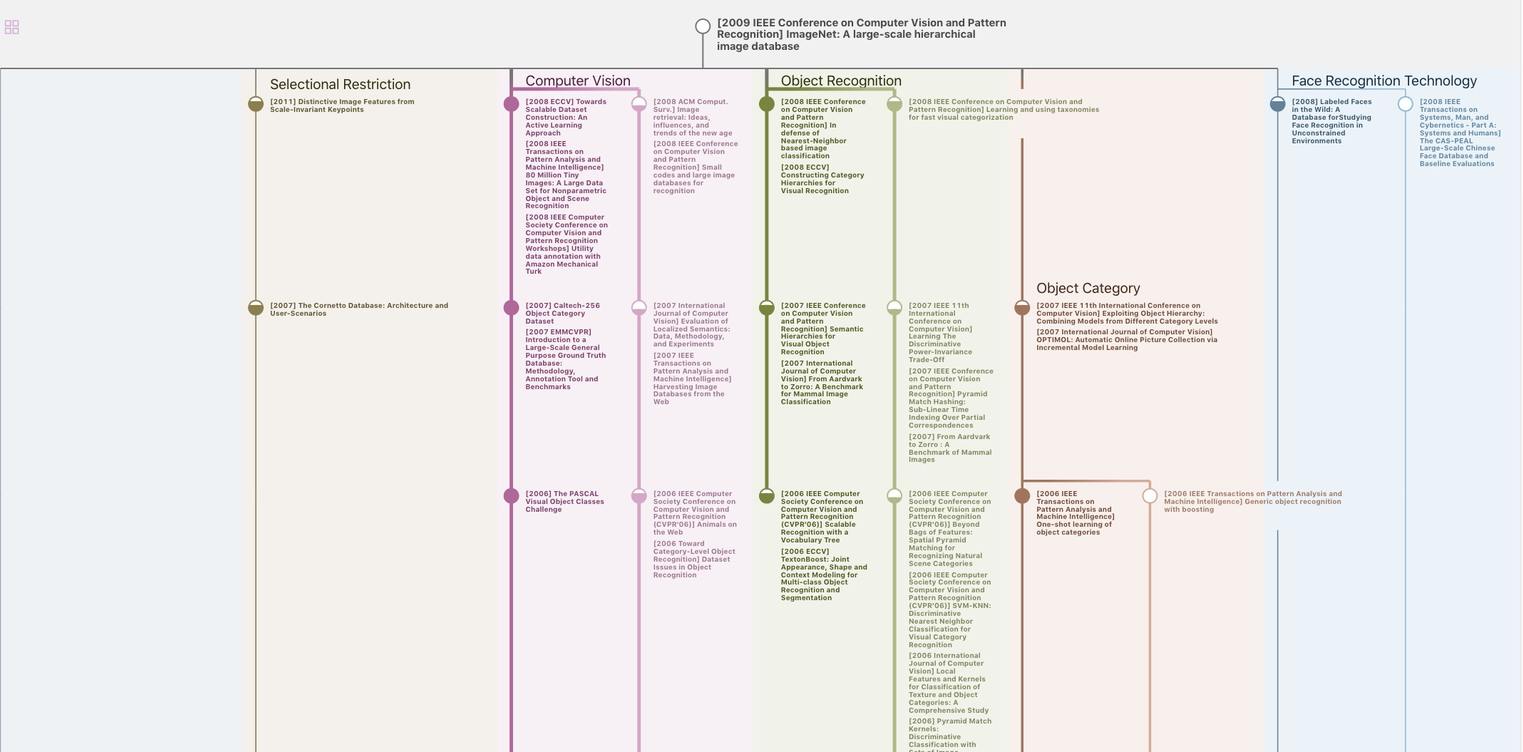
Generate MRT to find the research sequence of this paper
Chat Paper
Summary is being generated by the instructions you defined