On the Importance of In-distribution Class Prior for Out-of-distribution Detection
ICLR 2023(2023)
摘要
Given a pre-trained in-distribution (ID) model, the task of inference-time out-of-distribution (OOD) detection methods aims to recognize upcoming OOD data in inference time. However, some representative methods share an unproven assumption that the probability that OOD data belong to every ID class should be the same, i.e., probabilities that OOD data belong to ID classes form a uniform distribution. In this paper, we theoretically and empirically show that this assumption makes these methods incapable of recognizing OOD data when the ID model is trained with class-imbalanced data. Fortunately, by analyzing the causal relations between ID/OOD classes and features, we identify several common scenarios where probabilities that OOD data belong to ID classes should be the ID-class-prior distribution. Based on the above finding, we propose two effective strategies to modify previous inference-time OOD detection methods: 1) if they explicitly use the uniform distribution, we can replace the uniform distribution with the ID-class-prior distribution; 2) otherwise, we can reweight their scores according to the similarity between the ID-class-prior distribution and the softmax outputs of the pre-trained model. Extensive experiments show that both strategies significantly improve the accuracy of recognizing OOD data when the ID model is pre-trained with imbalanced data. As a highlight, when evaluating on the iNaturalist dataset, our method can achieve ~36% increase on AUROC and ~61% decrease on FPR95, compared with the original Energy method, reflecting the importance of ID-class prior in the OOD detection, which lights up a new road to study this problem.
更多查看译文
AI 理解论文
溯源树
样例
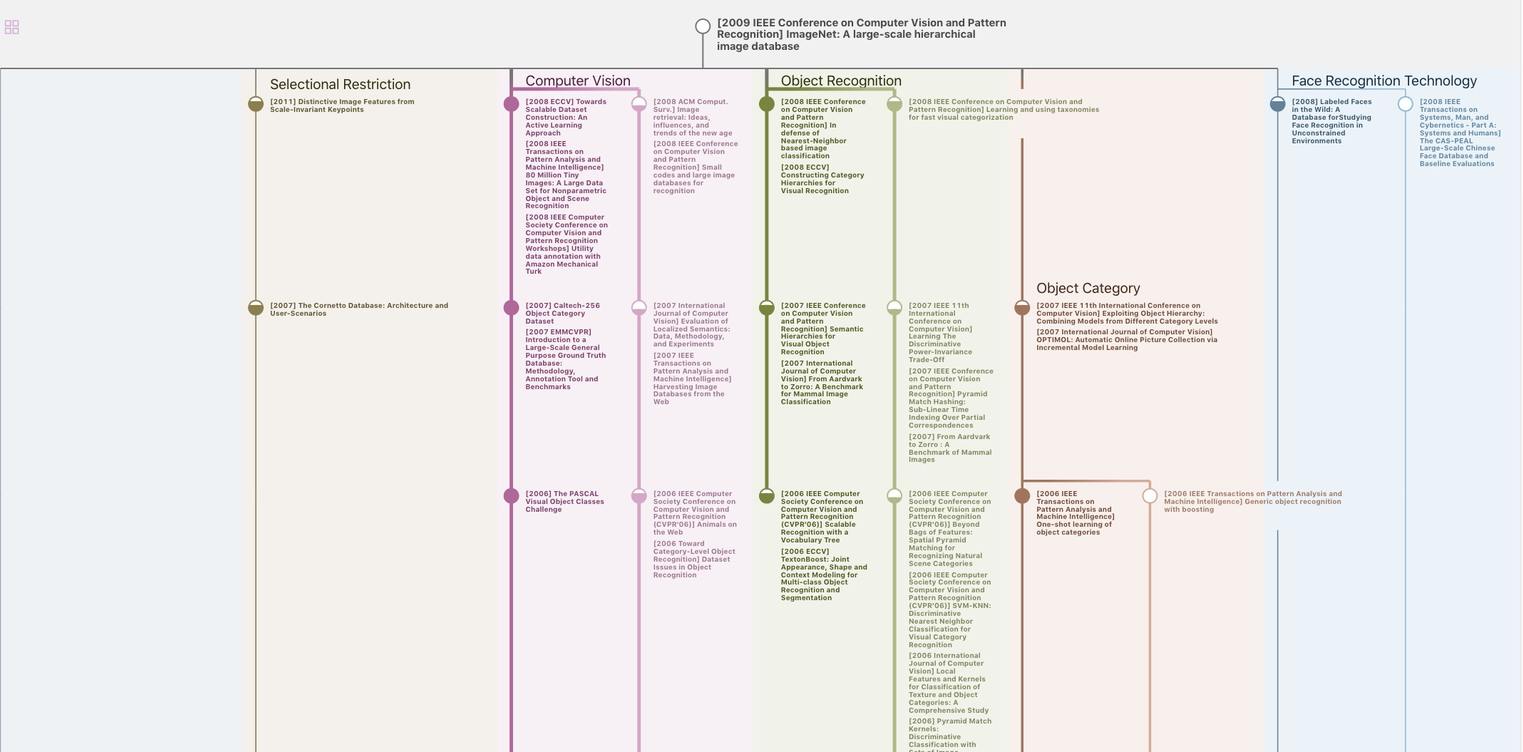
生成溯源树,研究论文发展脉络
Chat Paper
正在生成论文摘要