NOTELA: A Generalizable Method for Source Free Domain Adaptation
ICLR 2023(2023)
摘要
Source-free domain adaptation (SFDA) is a compelling problem as it allows to leverage any off-the-shelf model without requiring access to its original training set and adapts it using only unlabelled data. While several SFDA approaches have recently been proposed, their evaluation focuses on a narrow set of distribution shifts for vision tasks, and their generalizability outside of that scope has not yet been investigated. We put those recent approaches to the test by evaluating them on a new set of challenging---due to extreme covariate and label shift---and naturally-occurring distribution shifts in the audio domain. We study the task of adapting a bird species classifier trained on focalized recordings of bird songs to datasets of passive recordings for various geographical locations. Interestingly, we find that some recent SFDA methods underperform doing no adaptation at all. Drawing inspiration from those findings and insights, we propose a new method that improves on noisy student approaches by adjusting the teacher's pseudo-labels through Laplacian regularization. Our approach enjoys increased stability and significantly better performance on several of our proposed distribution shifts. We then look back at SFDA benchmarks in the vision domain and find that our approach is competitive with the state-of-the-art there as well.
更多查看译文
关键词
source-free domain adaptation,robustness to distribution shifts,bioacoustics
AI 理解论文
溯源树
样例
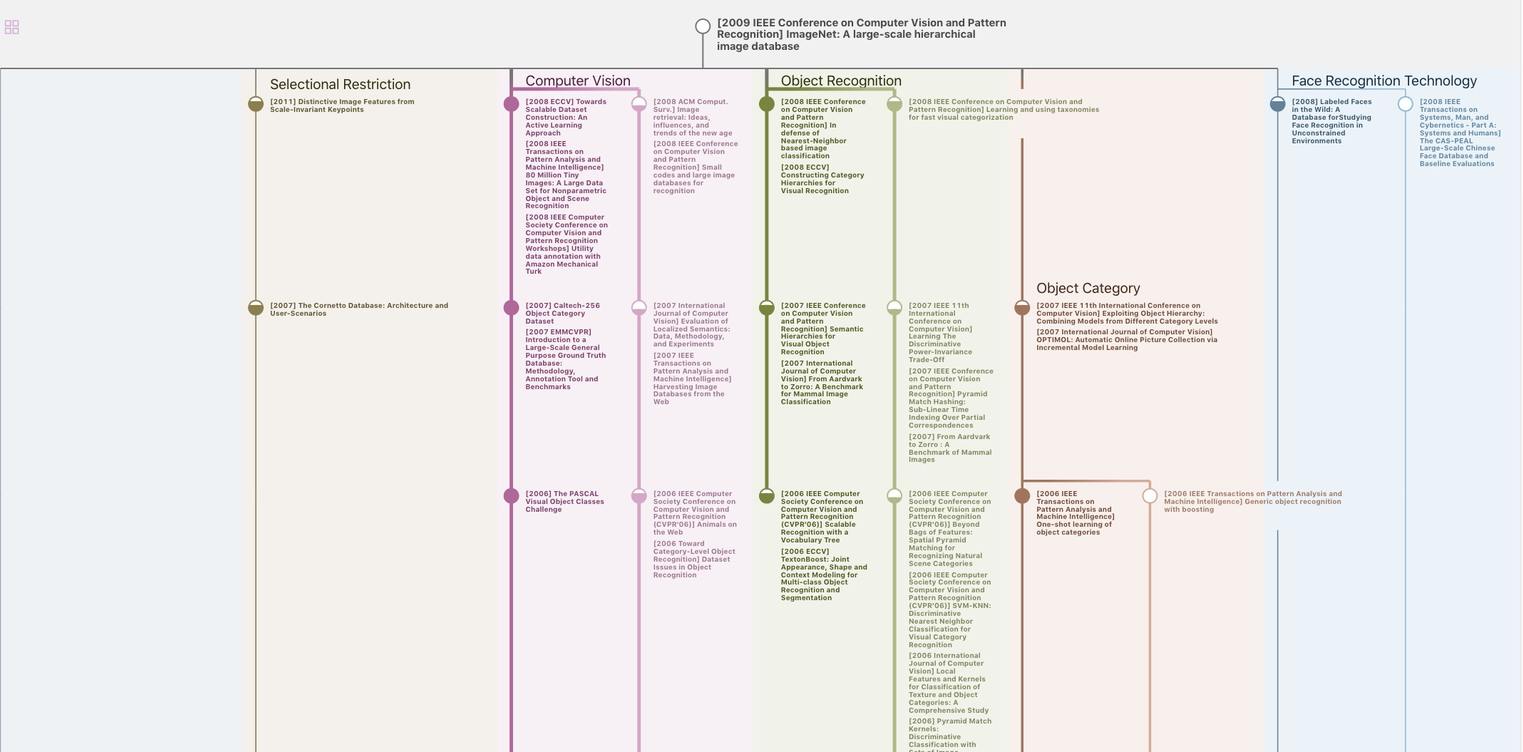
生成溯源树,研究论文发展脉络
Chat Paper
正在生成论文摘要