How Hard is Trojan Detection in DNNs? Fooling Detectors With Evasive Trojans
ICLR 2023(2023)
摘要
As AI systems become more capable and widely used, a growing concern is the possibility for trojan attacks in which adversaries inject deep neural networks with hidden functionality. Recently, methods for detecting trojans have proven surprisingly effective against existing attacks. However, there is comparatively little work on whether trojans themselves could be rendered hard to detect. To fill this gap, we develop a general method for making trojans more evasive based on several novel techniques and observations. Our method combines distribution-matching, specificity, and randomization to eliminate distinguishing features of trojaned networks. Importantly, our method can be applied to various existing trojan attacks and is detector-agnostic. In experiments, we find that our evasive trojans reduce the efficacy of a wide range of detectors across numerous evaluation settings while maintaining high attack success rates. Moreover, we find that evasive trojans are also harder to reverse-engineer, underscoring the importance of developing more robust monitoring mechanisms for neural networks and clarifying the offence-defense balance of trojan detection.
更多查看译文
关键词
trojan detection,neural trojans,trojans,hidden functionality,monitoring
AI 理解论文
溯源树
样例
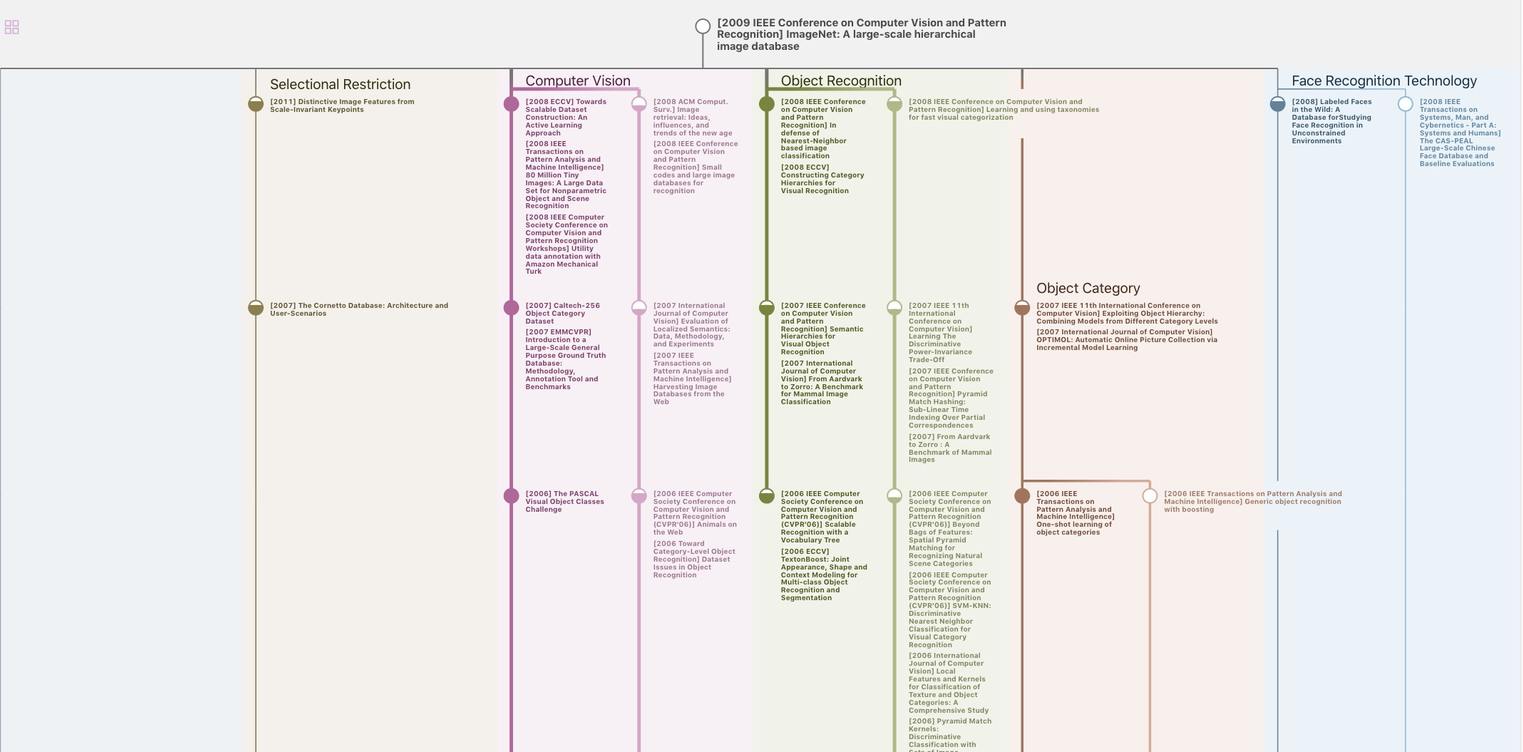
生成溯源树,研究论文发展脉络
Chat Paper
正在生成论文摘要