Cross-Window Self-Training via Context Variations from Sparsely-Labeled Time Series
ICLR 2023(2023)
摘要
A real-world time series is often sparsely labeled due to the expensive annotation cost. Recently, self-training methods have been applied to a dataset with few labels to infer the labels of unlabeled augmented instances. Accelerating this trend for time-series data, fully taking advantage of its sequential nature, we propose a novel data augmentation approach called context-additive augmentation, which allows a target instance to be augmented easily by adding preceding and succeeding instances to form an augmented instance. Unlike the existing augmentation techniques which may alter the target instance by directly perturbing its features, it preserves a target instance as is but still gives various augmented instances with varying contexts. Additionally, we propose a cross-window self-training framework based on the context-additive augmentation. The framework first augments target instances by applying context-varying windows over a given time series. Then, the framework derives reliability-based cross-window labels and uses them to maintain consistency among augmented instances across the windows. Extensive experiments using real datasets show that the framework outperforms the existing state-of-the-art self-training methods.
更多查看译文
关键词
semi-supervised learning,time series,pseudo labeling
AI 理解论文
溯源树
样例
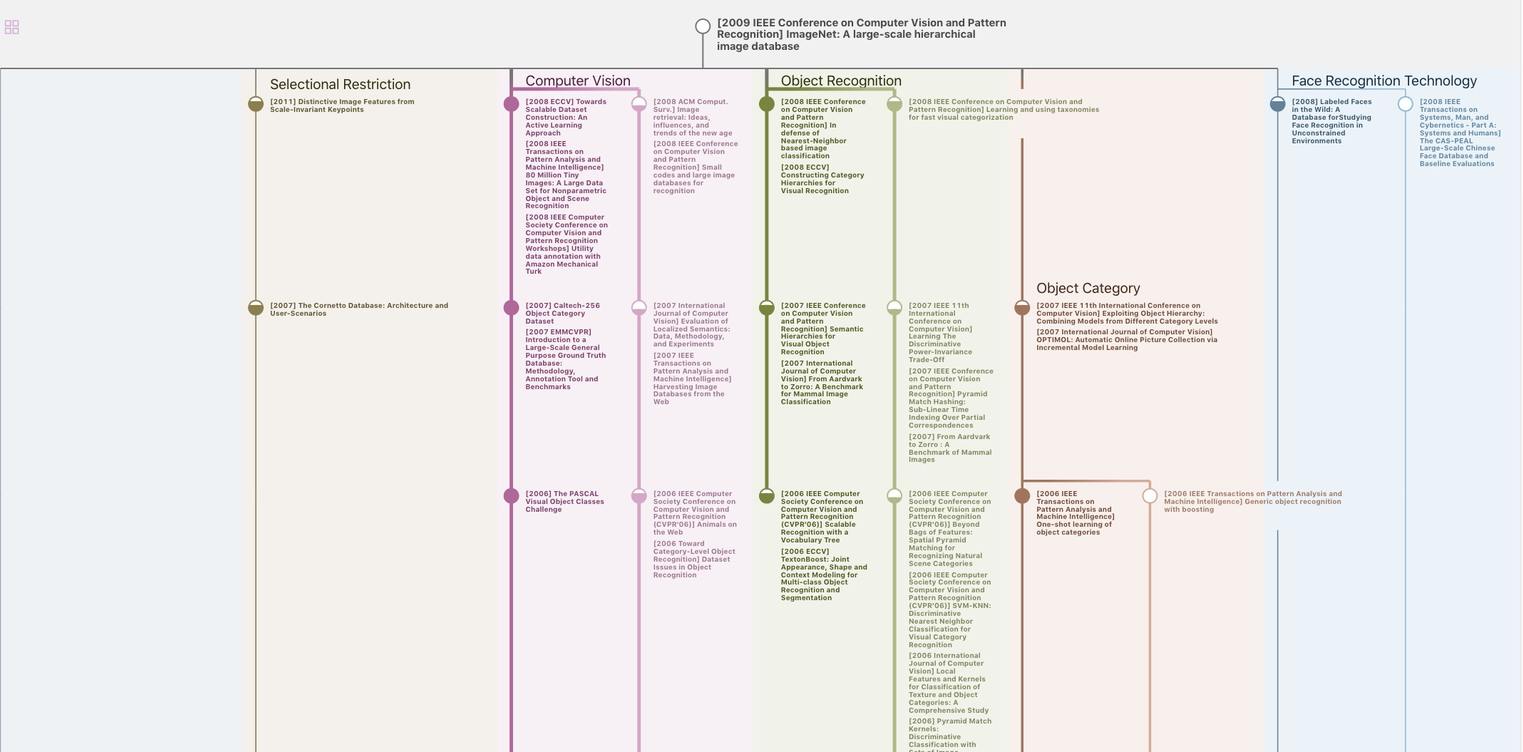
生成溯源树,研究论文发展脉络
Chat Paper
正在生成论文摘要