Multi Task Learning of Different Class Label Representations for Stronger Models
ICLR 2023(2023)
摘要
We find that the way in which class labels are represented can have a powerful effect on how well models trained on them learn. In classification, the standard way of representing class labels is as one-hot vectors. We present a new way of representing class labels called Binary Labels, where each class label is a large binary vector. We further introduce a new paradigm, multi task learning on different label representations. We train a network on two tasks. The main task is to classify images based on their one-hot label, and the auxiliary task is to classify images based on their Binary Label. We show that networks trained on both tasks have many advantages, including higher accuracy across a wide variety of datasets and architectures, both when trained from scratch and when using transfer learning. Networks trained on both tasks are also much more effective when training data is limited, and seem to do especially well on more challenging problems.
更多查看译文
关键词
Label Representation,Image Classification,Representation Learning,Multi-Task Learning
AI 理解论文
溯源树
样例
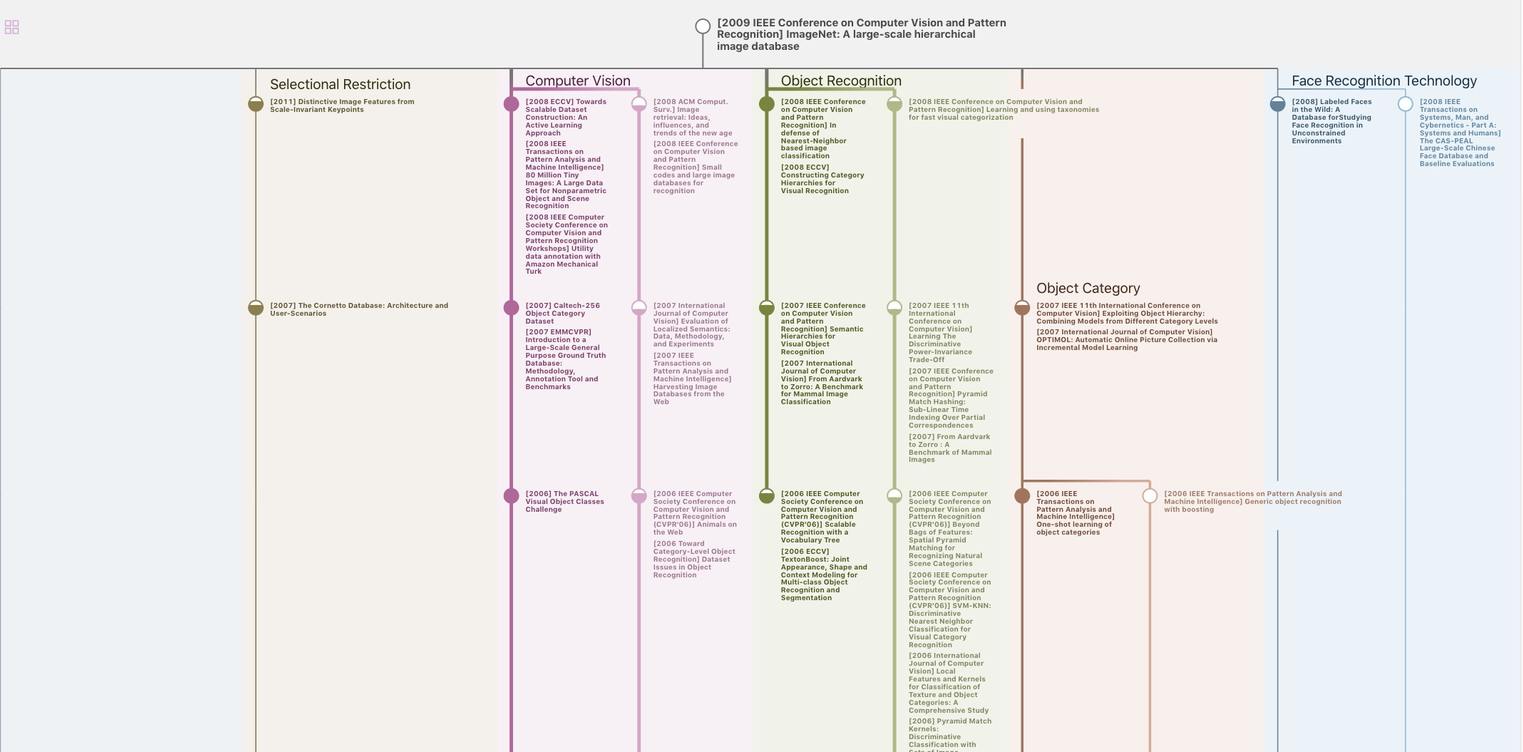
生成溯源树,研究论文发展脉络
Chat Paper
正在生成论文摘要