Variational Learning ISTA
ICLR 2023(2023)
摘要
Compressed sensing combines the power of convex optimization techniques with a sparsity inducing prior on the signal space to solve an underdetermined system of equations. For many problems, the sparsifying dictionary is not directly given, nor its existence can be assumed. Besides, the sensing matrix can change across different scenarios. Addressing these issues requires solving a sparse representation learning problem, namely dictionary learning, taking into account the epistemic uncertainty on the learned dictionaries and, finally, jointly learning sparse representations and reconstructions under varying sensing matrix conditions.
We propose a variant of the LISTA architecture that incorporates the sensing matrix into the architecture. In particular, we propose to learn a distribution over dictionaries via a variational approach, dubbed \ac{VLISTA}, which approximates a posterior distribution over the dictionaries as part of an unfolded LISTA-based recovery network. Such a variational posterior distribution is updated after each iteration, and thereby adapts the dictionary according to the optimization dynamics. As a result, \ac{VLISTA} provides a probabilistic way to jointly learn the dictionary distribution and the reconstruction algorithm with varying sensing matrices. We provide theoretical and experimental support for our architecture and show that it learns calibrated uncertainties.
更多查看译文
关键词
compressed sensing,LISTA,variational models,inverse problems
AI 理解论文
溯源树
样例
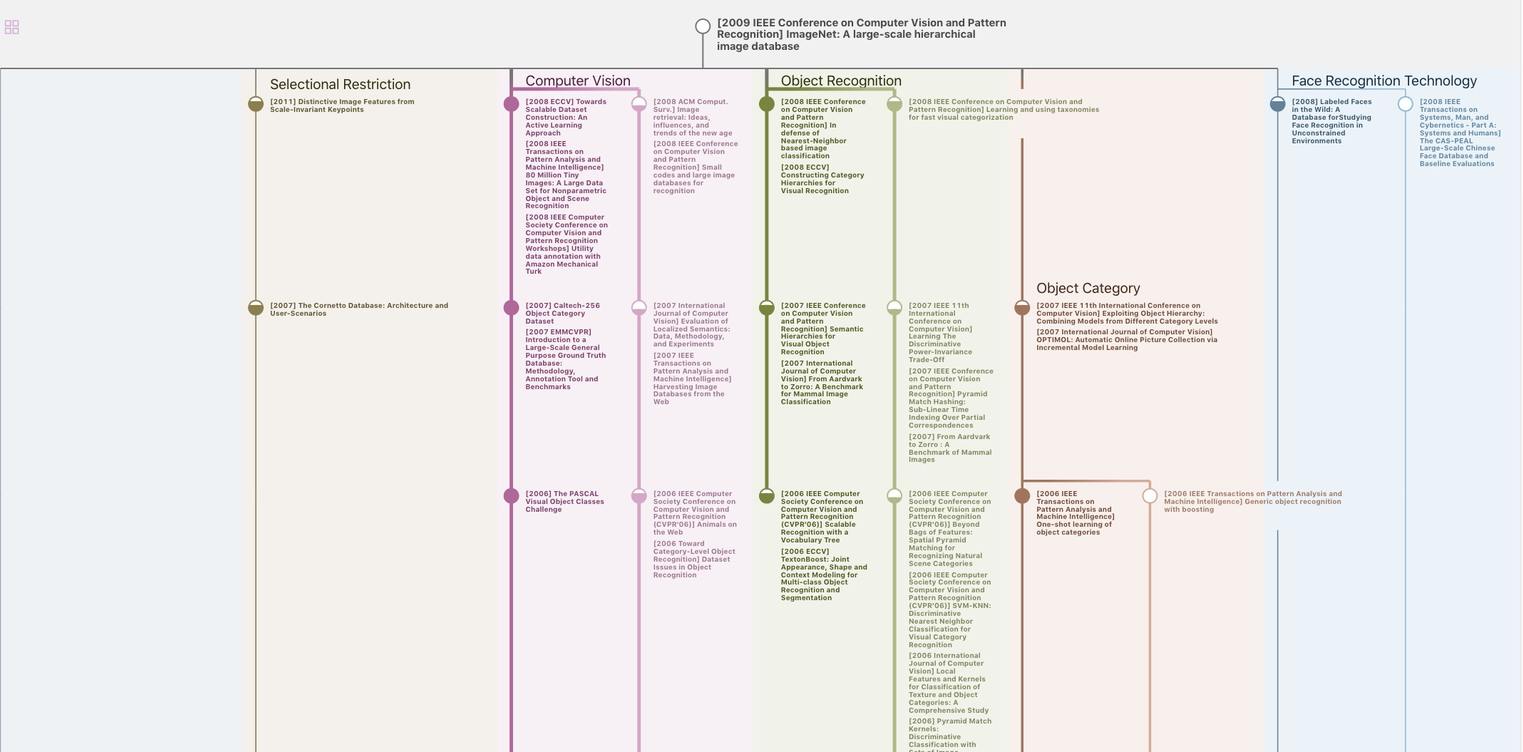
生成溯源树,研究论文发展脉络
Chat Paper
正在生成论文摘要