Disentangled Feature Swapping Augmentation for Weakly Supervised Semantic Segmentation
ICLR 2023(2023)
摘要
Weakly supervised semantic segmentation utilizes a localization map obtained from a classifier to generate a pseudo-mask. However, classifiers utilize background cues to predict class labels because of a biased dataset consisting of images, in which specific objects frequently co-occur with certain backgrounds. Consequently, the classifier confuses the background with the target objects, resulting in inaccurate localization maps. To this end, we propose DisEntangled FeaTure swapping augmentation(DEFT) to prevent the classifier from being biased by a misleading correlation. Our method first disentangles the foreground and background features. Then, we randomly swap the disentangled features within mini-batches via a two-way process. These features contain various contexts that do not appear in the biased dataset, but the class relevant representation is preserved. In addition, we introduce training schemes to obtain further performance gains. Experimental results showed that when our augmentation was used in various weakly supervised semantic segmentation methods trained on the Pascal VOC 2012 dataset, the performance of the localization maps and pseudo-mask as well as the segmentation results improved.
更多查看译文
关键词
Weakly Supervised Semantic Segmentation,Data Augmentation,Feature Disentanglement
AI 理解论文
溯源树
样例
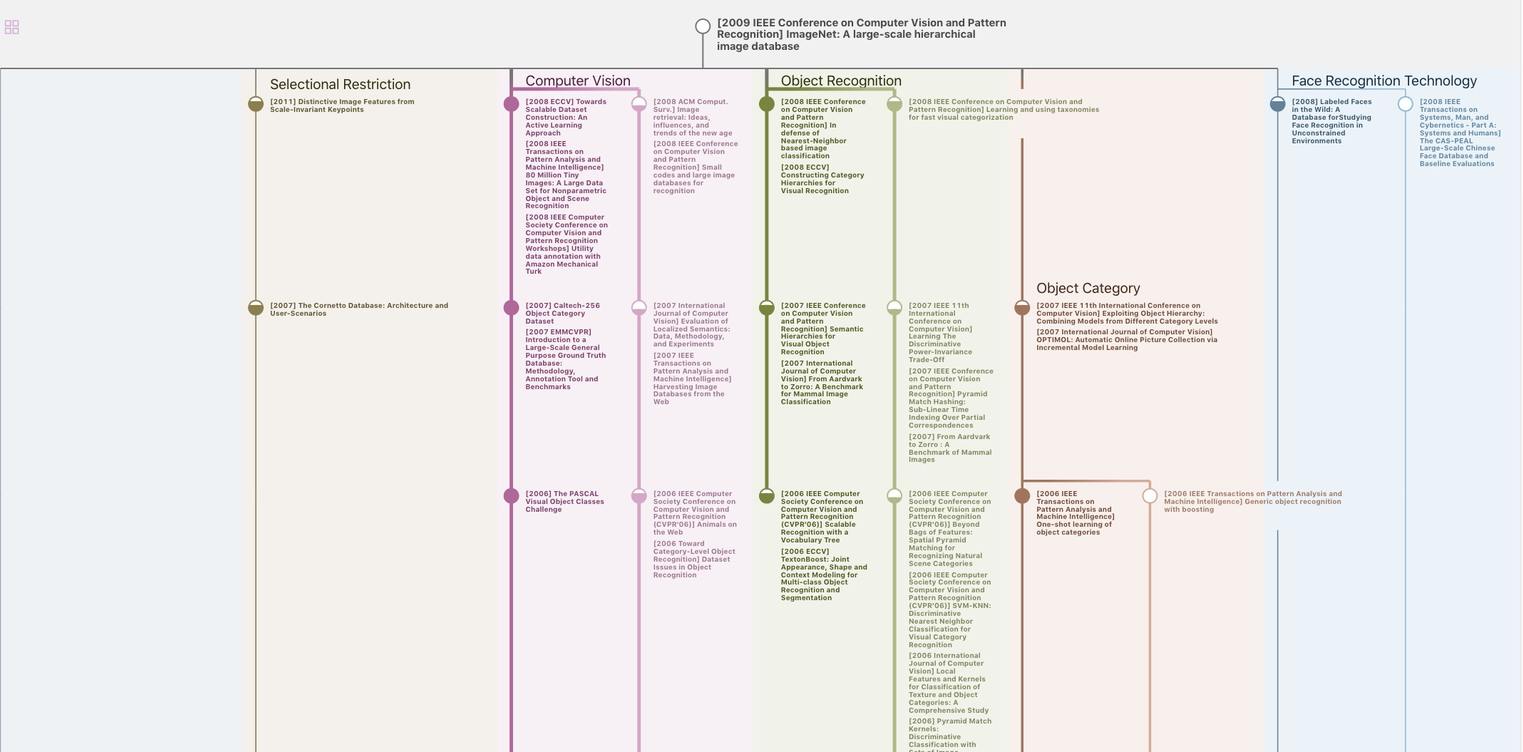
生成溯源树,研究论文发展脉络
Chat Paper
正在生成论文摘要