Predicting quantum chemical property with easy-to-obtain geometry via positional denoising
NeurIPS(2023)
摘要
As quantum chemical properties have a significant dependence on their geometries, graph neural networks (GNNs) using 3D geometric information have achieved high prediction accuracy in many tasks. However, they often require 3D geometries obtained from high-level quantum mechanical calculations, which are practically infeasible, limiting their applicability in real-world problems. To tackle this, we propose a method to accurately predict the properties with relatively easy-to-obtain geometries (e.g., optimized geometries from the molecular force field). In this method, the input geometry, regarded as the corrupted geometry of the correct one, gradually approaches the correct one as it passes through the stacked denoising layers. We investigated the performance of the proposed method using 3D message-passing architectures for two prediction tasks: molecular properties and chemical reaction property. The reduction of positional errors through the denoising process contributed to performance improvement by increasing the mutual information between the correct and corrupted geometries. Moreover, our analysis of the correlation between denoising power and predictive accuracy demonstrates the effectiveness of the denoising process.
更多查看译文
AI 理解论文
溯源树
样例
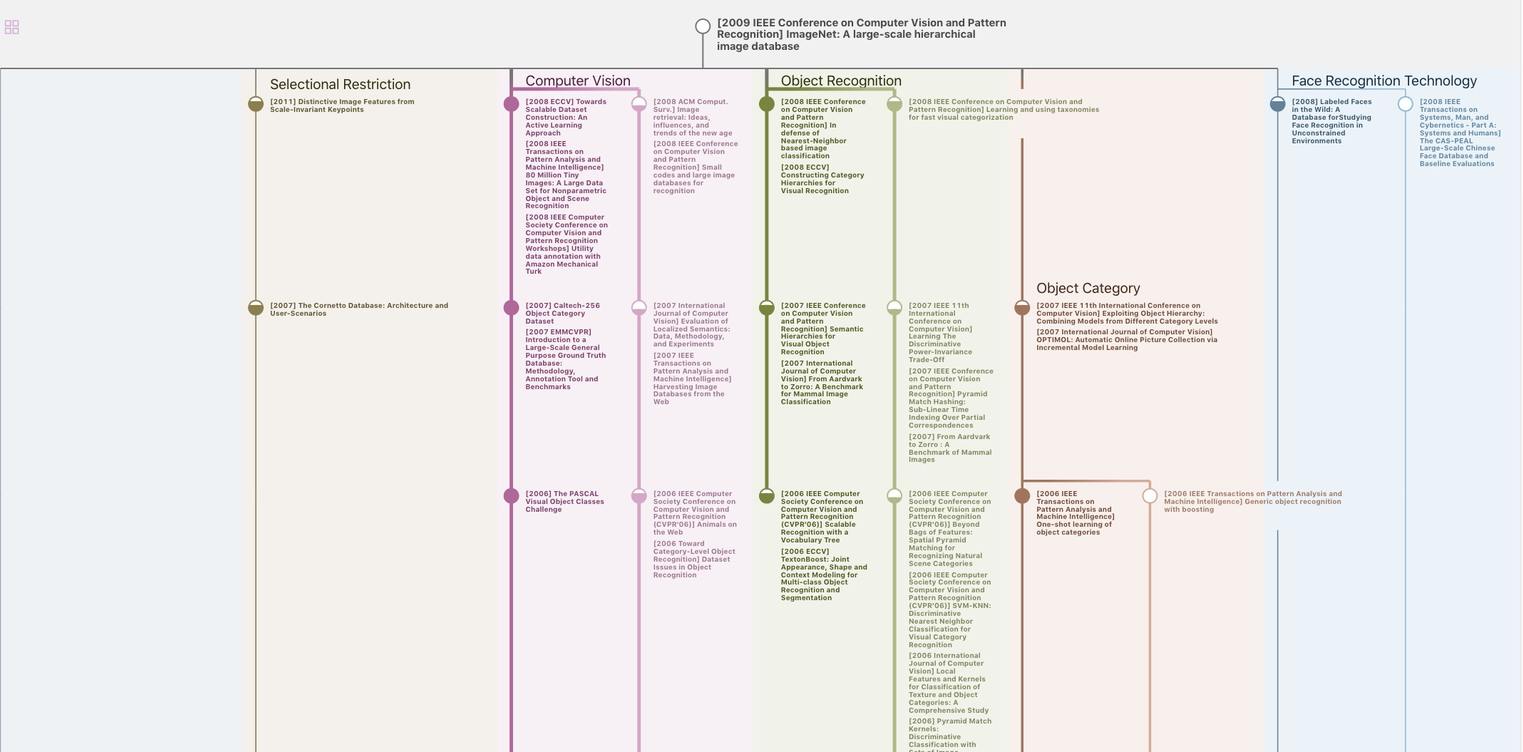
生成溯源树,研究论文发展脉络
Chat Paper
正在生成论文摘要