Distributional Signals for Node Classification in Graph Neural Networks
CoRR(2023)
Abstract
In graph neural networks (GNNs), both node features and labels are examples of graph signals, a key notion in graph signal processing (GSP). While it is common in GSP to impose signal smoothness constraints in learning and estimation tasks, it is unclear how this can be done for discrete node labels. We bridge this gap by introducing the concept of distributional graph signals. In our framework, we work with the distributions of node labels instead of their values and propose notions of smoothness and non-uniformity of such distributional graph signals. We then propose a general regularization method for GNNs that allows us to encode distributional smoothness and non-uniformity of the model output in semi-supervised node classification tasks. Numerical experiments demonstrate that our method can significantly improve the performance of most base GNN models in different problem settings.
MoreTranslated text
Key words
Graph neural networks,graph signal processing,regularization,smoothness,node classification
AI Read Science
Must-Reading Tree
Example
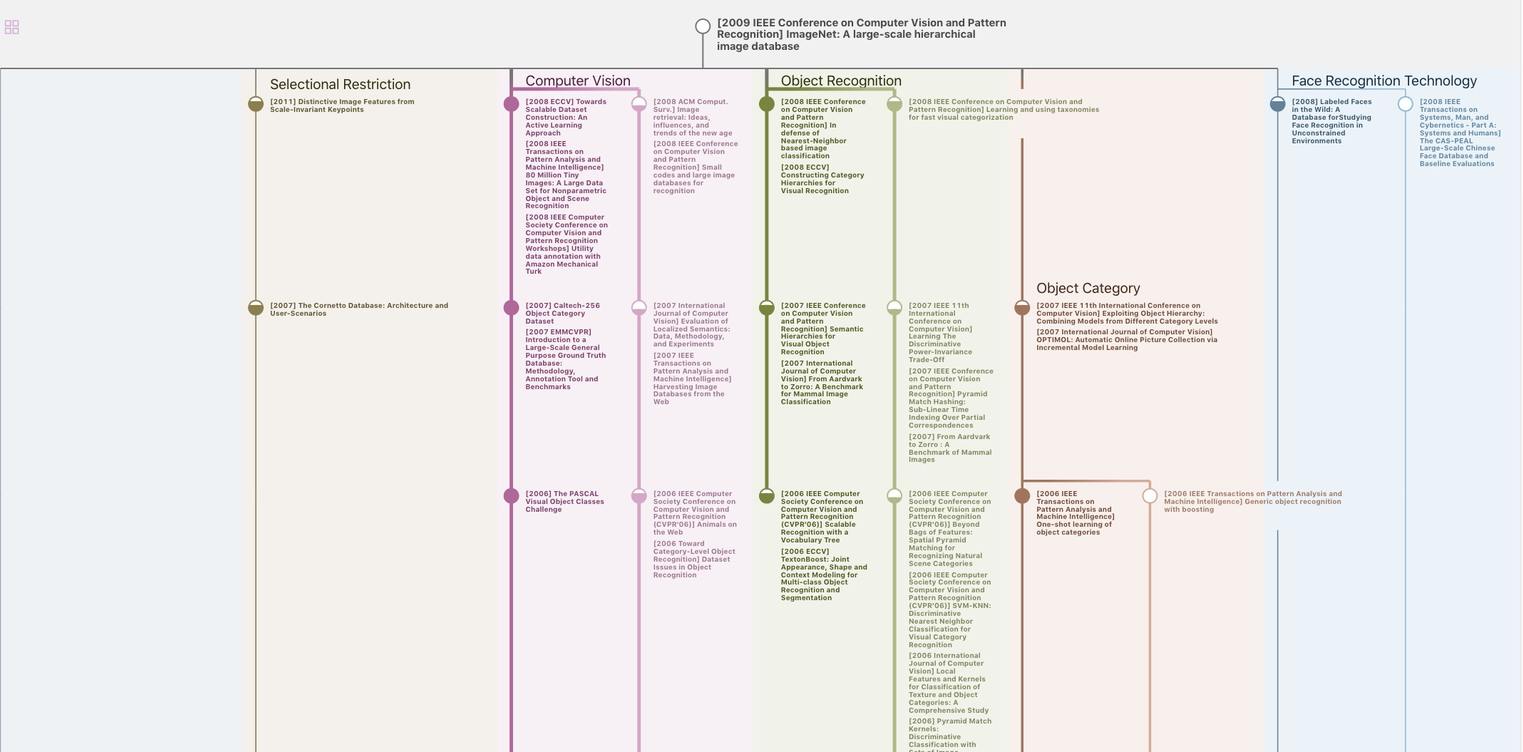
Generate MRT to find the research sequence of this paper
Chat Paper
Summary is being generated by the instructions you defined