Enhanced-feature pyramid network for semantic segmentation.
ICAIIC(2023)
摘要
Semantic segmentation is a complicated topic when they require strictly the object boundary accuracy. For autonomous driving applications, they have to face a long range of objective sizes in the street scenes, so a single field of views is not suitable to extract input features. Feature pyramid network (FPN) is an effective method for computer vision tasks such as object detection and semantic segmentation. The architecture of this approach composes of a bottom-up pathway and a top-down pathway. Based on the structure, we can obtain rich spatial information from the largest layer and extract rich segmentation information from lower-scale features. The traditional FPN efficiently captures different objective sizes by using multiple receptive fields and then predicts the outputs from the concatenated features. The final feature combination is not optimistic when they burden the hardware with huge computation and reduce the semantic information. In this paper, we propose multiple predictions for semantic segmentation. Instead of combining four-feature scales together, the proposed method processes separately three lower scales as the contextual contributor and the largest features as the coarser-information branch. Each contextual feature is concatenated with the coarse branch to generate an individual prediction. By deploying this architecture, a single prediction effectively segments specific objective sizes. Finally, score maps are fused together in order to gather the prominent weights from the different predictions. A series of experiments is implemented to validate the efficiency on various open data sets. We have achieved good results 76.4% mIoU at 52 FPS on Cityscapes and 43.6% mIoU on Mapillary Vistas.
更多查看译文
关键词
Semantic segmentation, feature pyramid network, multiscale prediction, real-time application
AI 理解论文
溯源树
样例
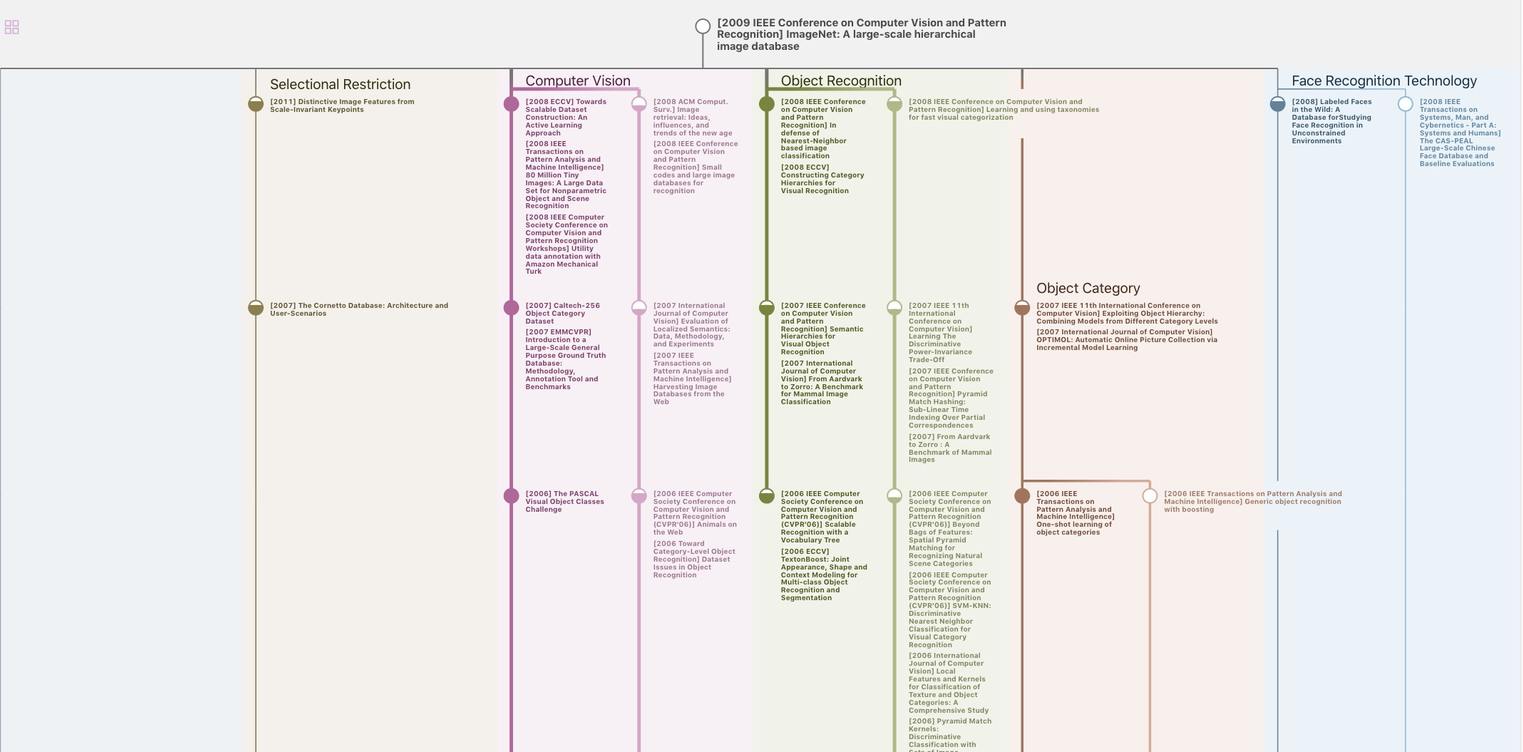
生成溯源树,研究论文发展脉络
Chat Paper
正在生成论文摘要