Multi-relational Affinity Propagation.
ASONAM(2022)
Abstract
There is a growing need for clustering algorithms which can operate in complex settings where there are multiple entity types with potential dependencies captured in different kinds of links. In this work, we present a novel approach for multi-relational clustering based on both the similarity of the entities' features, along with the multi-relational structure of the network among the entities. Our approach extends the affinity propagation clustering algorithm to multi-relational domains and encodes a variety of relational constraints to capture the dependencies across different node types in the underlying network. In contrast to the original formulation of affinity propagation that relies on enforcing hard constraints on the output clusters, we model the relational dependencies as soft constraints, allowing control over how they influence the final clustering of the nodes. This formulation allows us to balance between the homogeneity of the entities within the resulting clusters and their connections to clusters of nodes of the same and differing types. This in turn facilitates the exploration of the middle ground between feature-based similarity clustering, community detection, and block modeling in multi-relational networks. We present results on clustering a sample from Digg.com, a richly structured online social news website. We show that our proposed algorithm outperforms other clustering approaches on a variety of evaluation measures. We also analyze the impact of different parameter settings on the clustering output, in terms of both the homogeneity and the connectedness of the resulting clusters.
MoreTranslated text
AI Read Science
Must-Reading Tree
Example
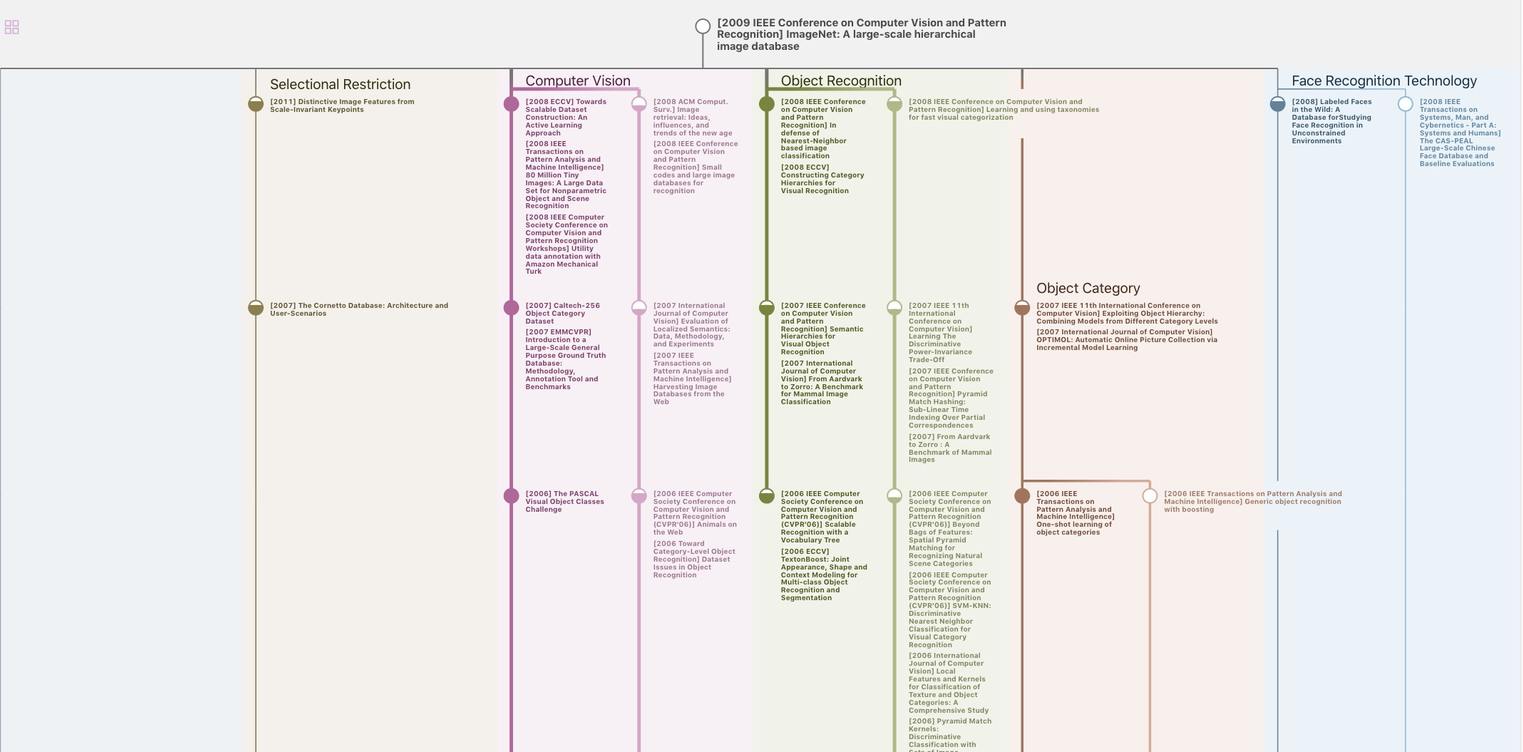
Generate MRT to find the research sequence of this paper
Chat Paper
Summary is being generated by the instructions you defined