Multimodal medical image fusion based on visual saliency map and multichannel dynamic threshold neural P systems in sub-window variance filter domain
BIOMEDICAL SIGNAL PROCESSING AND CONTROL(2023)
摘要
As a powerful image enhancement technique, multimodal medical image fusion has been widely used for biomedical diagnosis and surgical navigation. However, the trade-off between efficiency and fusion quality for existing fusion images remains a great challenge. In this study, a robust and efficient medical image fusion method based on a sub-window variance filter (SVF) is proposed to overcome the above problems. First, the input images are decomposed two-layer using SVF, the base layer including rich energy and contour intensity information, and the detail layer containing integral detail features. Then, a contrast function is employed to improve the MRI base layer and a neighbor energy function to refine the detail layer. Next, a novel multichannel dynamic threshold neural P system is proposed to fuse the detail layer, which can provide a comprehensive consideration of the information in the detail layer and compensate well for the defect of the single-channel model. Moreover, a visual saliency map (VSM-based) is designed to fuse the base layer, preserving important energy information and improving image contrast. Finally, the fused result is reconstructed by the inverse SVF. Ten representative current multimodal medical image fusion methods are compared, and six typical quality evaluation metrics are combined to objectively evaluate the fused images. Tremendous experimental results indicate that the proposed model can effectively preserve higher contrast, more complete edge features, and better color than some state-of-the-art algorithms, both visual quality and quantitative evaluations on public datasets.
更多查看译文
关键词
Multimodal medical image fusion,Sub-window variance filter,Visual saliency map,Multichannel neural P system
AI 理解论文
溯源树
样例
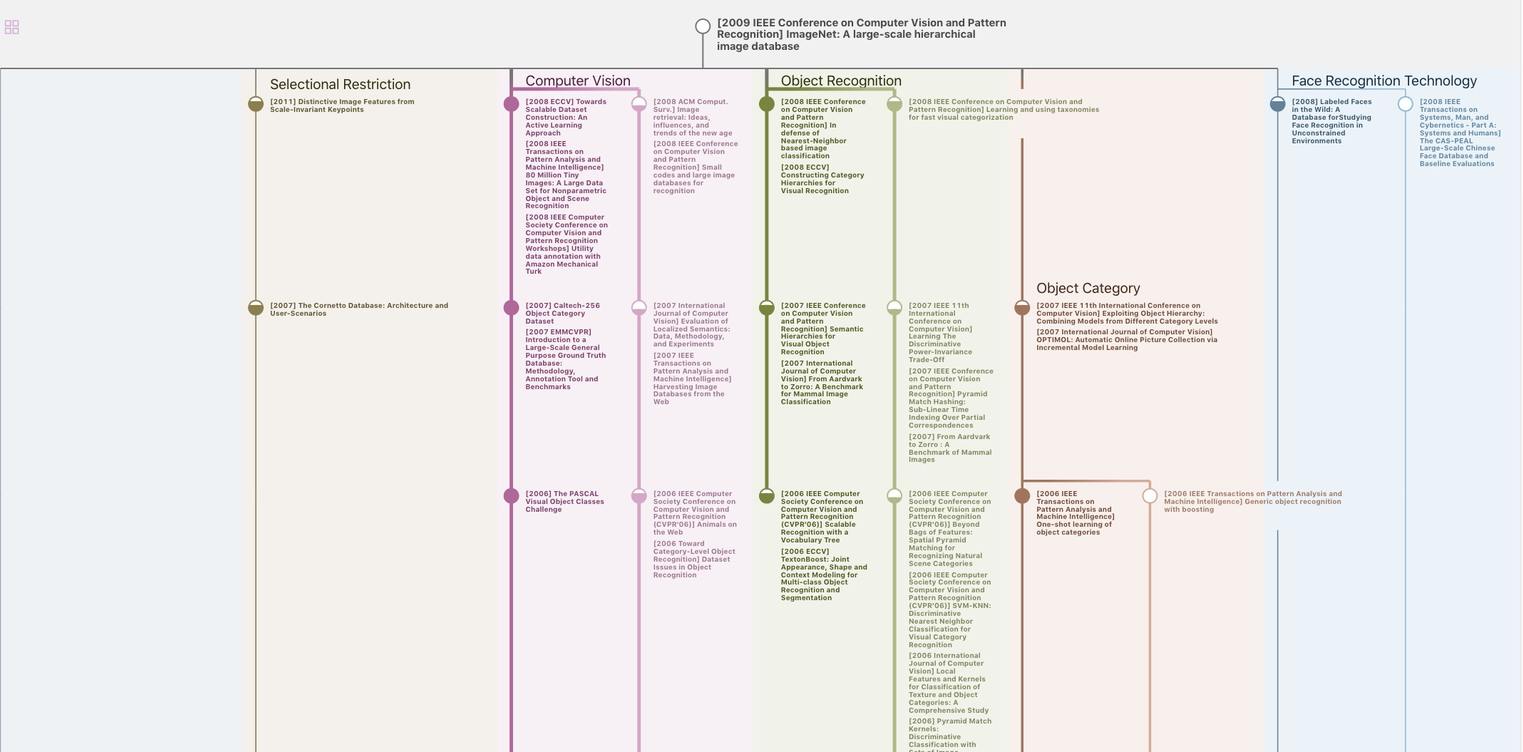
生成溯源树,研究论文发展脉络
Chat Paper
正在生成论文摘要