CAE-Net: Cross-Modal Attention Enhancement Network for RGB-T Salient Object Detection
ELECTRONICS(2023)
摘要
RGB salient object detection (SOD) performs poorly in low-contrast and complex background scenes. Fortunately, the thermal infrared image can capture the heat distribution of scenes as complementary information to the RGB image, so the RGB-T SOD has recently attracted more and more attention. Many researchers have committed to accelerating the development of RGB-T SOD, but some problems still remain to be solved. For example, the defective sample and interfering information contained in the RGB or thermal image hinder the model from learning proper saliency features, meanwhile the low-level features with noisy information result in incomplete salient objects or false positive detection. To solve these problems, we design a cross-modal attention enhancement network (CAE-Net). First, we concretely design a cross-modal fusion (CMF) module to fuse cross-modal features, where the cross-attention unit (CAU) is employed to enhance the two modal features, and channel attention is used to dynamically weigh and fuse the two modal features. Then, we design the joint-modality decoder (JMD) to fuse cross-level features, where the low-level features are purified by higher level features, and multi-scale features are sufficiently integrated. Besides, we add two single-modality decoder (SMD) branches to preserve more modality-specific information. Finally, we employ a multi-stream fusion (MSF) module to fuse three decoders' features. Comprehensive experiments are conducted on three RGB-T datasets, and the results show that our CAE-Net is comparable to the other methods.
更多查看译文
关键词
salient object detection,multi-stream fusion,cross-attention unit,cross-modal fusion,single-/joint-modality decoder
AI 理解论文
溯源树
样例
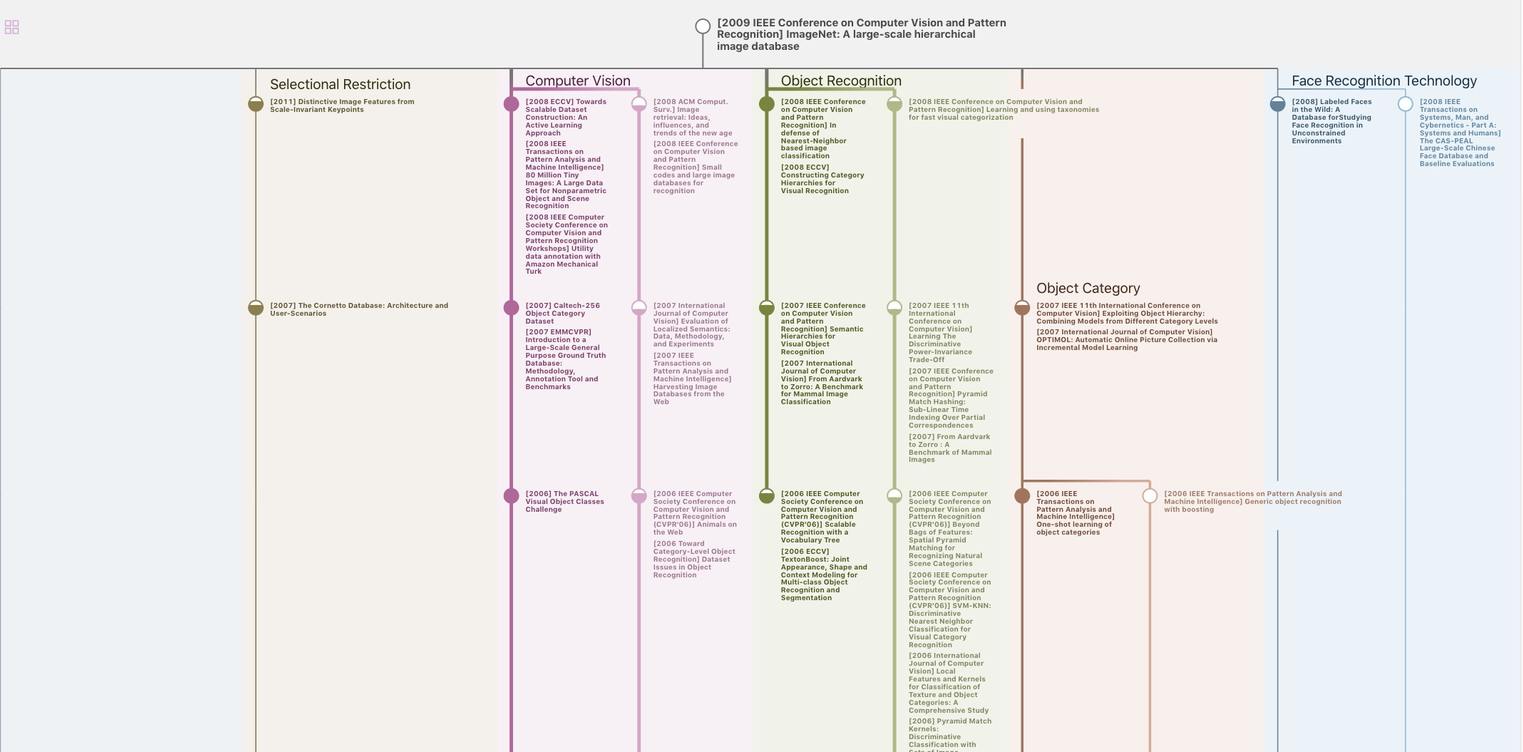
生成溯源树,研究论文发展脉络
Chat Paper
正在生成论文摘要