A Wrapped Approach Using Unlabeled Data for Diabetic Retinopathy Diagnosis
APPLIED SCIENCES-BASEL(2023)
摘要
Large-scale datasets, which have sufficient and identical quantities of data in each class, are the main factor in the success of deep-learning-based classification models for vision tasks. A shortage of sufficient data and interclass imbalanced data distribution, which often arise in the medical domain, cause modern deep neural networks to suffer greatly from imbalanced learning and overfitting. A diagnostic model of diabetic retinopathy (DR) that is trained from such a dataset using supervised learning is severely biased toward the majority class. To enhance the efficiency of imbalanced learning, the proposal of this study is to leverage retinal fundus images without human annotations by self-supervised or semi-supervised learning. The proposed approach to DR detection is to add an auxiliary procedure to the target task that identifies DR using supervised learning. The added process uses unlabeled data to pre-train the model that first learns features from data using self-supervised or semi-supervised learning, and then the pre-trained model is transferred with the learned parameter to the target model. This wrapper algorithm of learning from unlabeled data can help the model gain more information from samples in the minority class, thereby improving imbalanced learning to some extent. Comprehensive experiments demonstrate that the model trained with the proposed method outperformed the one trained with only the supervised learning baseline utilizing the same data, with an accuracy improvement of 4 similar to 5%. To further examine the method proposed in this study, a comparison is conducted, and our results show that the proposed method also performs much better than some state-of-the-art methods. In the case of EyePaCS, for example, the proposed method outperforms the customized CNN model by 9%. Through experiments, we further find that the models trained with a smaller but balanced dataset are not worse than those trained with a larger but imbalanced dataset. Therefore, our study reveals that utilizing unlabeled data can avoid the expensive cost of collecting and labeling large-scale medical datasets.
更多查看译文
关键词
deep learning,pre-trained model,self-supervised learning,semi-supervised learning,diabetic retinopathy,imbalanced data distribution
AI 理解论文
溯源树
样例
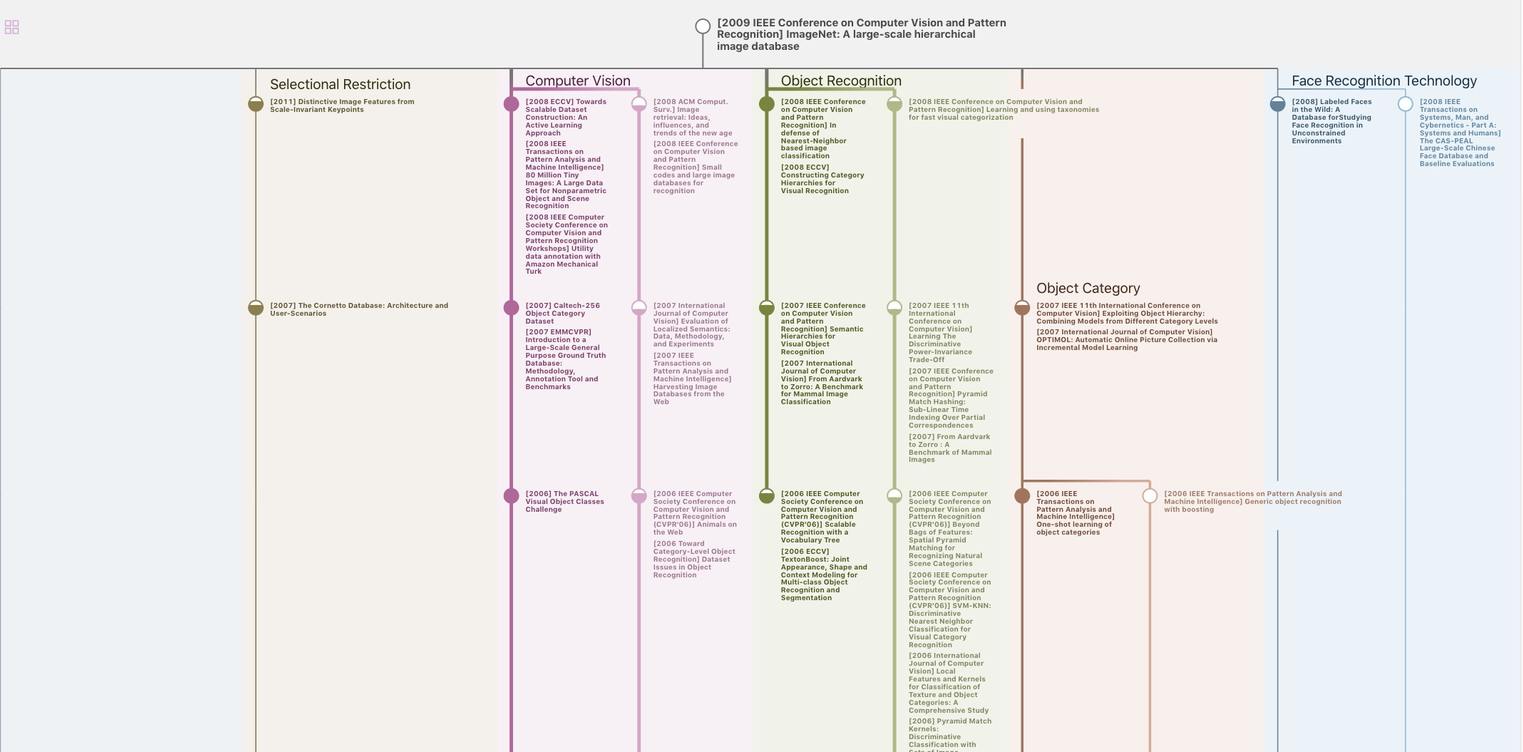
生成溯源树,研究论文发展脉络
Chat Paper
正在生成论文摘要