Ensemble machine learning for modeling greenhouse gas emissions at different time scales from irrigated paddy fields
FIELD CROPS RESEARCH(2023)
摘要
Quantifying greenhouse gas (GHG) emissions from irrigated paddy fields is of great significance for addressing climate change. Machine learning (ML) provides an alternative to empirical models and biogeochemical models, but it has not been used to simulate GHG emissions from paddy fields. In this study, a three-year field experiment dataset of paddy fields under controlled irrigation (CI, a kind of water-saving irrigation (WSI) technology) and flood irrigation (FI) in Kunshan, China, was collected, as well as a global dataset on WSI and FI. Then, the stacking ensemble model was developed based on three basic ML models, random forest (RF), K-Nearest Neighbor regression (KNN), gradient boosting regression (GBR), and a meta-learner, linear Regression (LR). Those models were used for the first time to simulate CH4 and N2O emissions on different time scales from paddy fields under WSI and FI. The results showed that the cumulative CH4 and N2O emissions from the WSI paddy fields decreased by 61.14% and increased by 19.52%, respectively, compared with FI. The ML algorithm can be applied to simulate daily, growth stages, and cumulative GHG emissions from paddy fields, but the performance of the linear regression model was worse than other ML models. Compared with the basic models, the stacking model improved the accuracy, improving the R2 by 0.37-13.36% and reducing the RMSE by 3.23-42.78%. Soil redox, temperature, and moisture are necessary for accurate modeling. Meanwhile, training the model using data from WSI and FI separately is beneficial to improve the accuracy of the model. The availability of the stacking model in other stations was verified based on literature data, with R2 varying between 0.7634 and 0.9985. Therefore, the stacking model is recommended to predict GHG emissions at different time scales from paddy fields, and this method can be extended to estimate GHG from paddy fields at various stations around the world.
更多查看译文
关键词
Machine learning,Stacking,Greenhouse gas,Water -saving irrigation,Paddy
AI 理解论文
溯源树
样例
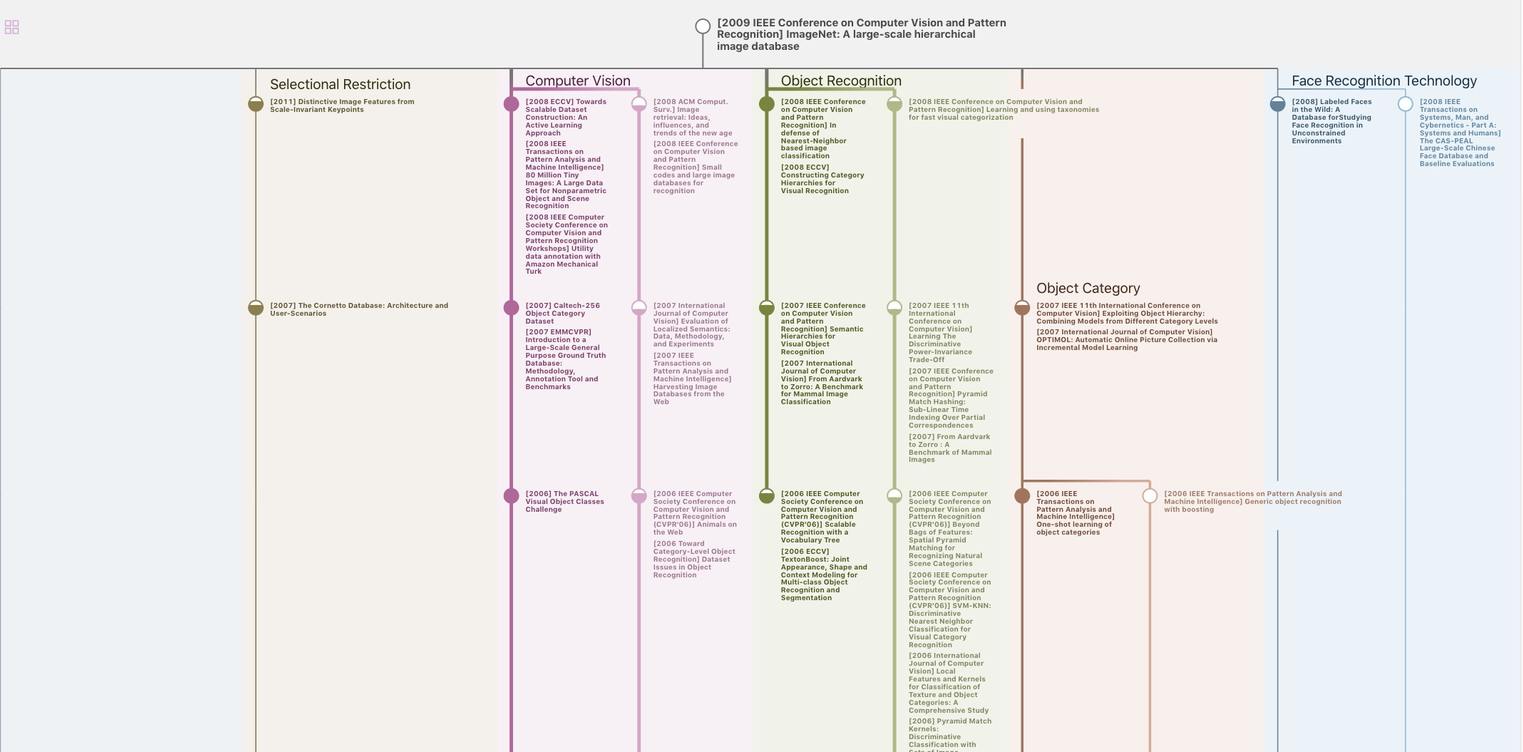
生成溯源树,研究论文发展脉络
Chat Paper
正在生成论文摘要