Emerging robust and data-driven control methods for uncertain learning systems
INTERNATIONAL JOURNAL OF ROBUST AND NONLINEAR CONTROL(2023)
摘要
Learning systems represent a particularly important class of practical data-driven systems that adapt to their environment based on the environment's response to the system's action. In some settings traditional robust and adaptive control techniques have been verified to be effective when plants are large scale and/or have very complex dynamics (e.g., industrial processes, power grid networks, and transportation systems). However, often in these plants the problems of parameter mismatch and unmodeled dynamics are encountered, and thus the techniques of robust or adaptive control methods in the framework of modern control may no longer be sufficient. As an alternative to robust or adaptive methods, various learning paradigms have been established, for example, reinforcement learning, deep learning, artificial neural networks, and iterative learning, among others. These learning paradigms construct controllers or control signals directly with the data collected and stored when the system operates, typically without the need of identifying system models. However, even such “model-independent” control systems must make assumptions about the system dynamics. One such common assumption is that the system dynamics are linear. Another is that they are time-invariant (autonomous). When the dynamics do not meet these assumptions, traditional learning paradigms may also fail. Despite the success of learning-based methods, finding suitable control frameworks for learning systems when there is uncertainty in the assumptions related to the system dynamics is still an open problem. The aim of this special issue is to collect recent research results that address issues involved in data-driven control methods and related algorithms for learning-based systems that operate under uncertainty. The special issue consists of fourteen papers covering several key areas of activity in which progress has been achieved. Many of the papers also include results of simulation or experimental tests of the presented control methods. Next we give a brief description of each of the fourteen papers. We have organized the papers thematically into four categories: (1) iterative learning control (ILC), (2) neural networks and machine learning, (3) identification and control, and (4) applications. The first five papers describe research on the control method of ILC, which is a process developed especially for applications where the same operation needs to be repeated over a limited period of time. In the first of these papers, Ding and Li give results on an adaptive ILC algorithm for nonlinear continuous nonparameterized systems. This adaptive ILC algorithm, which can adjust the adaptive parameters in both the iteration domain and the time domain, is proposed to track different reference trajectories repetitively over a finite time interval, and also to provide a unified adaptive control strategy for nonlinear continuous nonparameterized systems that have asymmetric control gain matrices for trajectory tracking in different domains. In the next paper, Chen and Chu address the fact that in multiagent collaborative tracking tasks, the system often encounters constraints in practice that cause challenging difficulties in the case of handling large-scale systems. They propose a novel constrained ILC design and further develop a decentralized implementation of the resulting ILC algorithm using the alternating direction method of multipliers, allowing the design to scale up to handle large-scale and varying system dynamics. The next three papers consider the special problem of ILC when there are quantization and intersample behavior effects. In the third paper, Ohnishi et al. develop a framework for a state-tracking ILC that mitigates the oscillatory intersample behavior that is often encountered in output-tracking ILC. The paper addresses stability in the iteration domain, introducing the idea of a robustness filter, designed in the frequency domain. The last two ILC papers both consider quantization effects. Zhang et al. simultaneously tackle the problems of predictive compensation, unknown nonlinearity, and nonaffine structure found in a quantized ILC analysis and design under a data-driven framework. Using the concept of a virtual iterative linear data model, the authors develop control laws that are completely data driven and model free. The established results are also generalized to a class of multi-input multi-output, nonlinear, nonaffine, discrete-time systems. Finally, Huo et al. investigate a quantized ILC method with an encoding–decoding mechanism for networked control systems subject to constrained transmission bandwidths and random data dropouts on both the measurement and the actuator side. Their approach is to utilize an intermittent update principle to develop a control algorithm that can demonstrate enhanced tracking performance. The next three papers use neural network and machine learning (ML) approaches to deal with uncertain systems. First, with the aid of neural networks, Xin et al. develop a neural network-based learning design for a class of high-order nonlinear systems involving full-state constraints in the form of output feedback control. Then, Chen et al. consider non-repetitive time-varying systems. They propose an ML-based nominal model updating mechanism that uses linear regression techniques to update the nominal model in each ILC trial, with only the current trial information, which improves the performance of the ILC. This paper provides information on how ML/ILC parameters can be tuned to achieve desired performance. The ideas are illustrated through experiments on a real gantry robot test platform. Another ML-based problem considers robust approximate optimal control of an air-breathing hypersonic vehicle in the paper by Han et al. The learning-based control framework can guarantee practical finite-time convergence of the tracking error, without chattering. Further, the proposed controller adopts a critic-only network design instead of an actor-critic structure, which simplifies the implementation procedure. The next three papers consider the issue of data-driven identification and control. With the aid of subspace methods and an average consensus algorithm, Cheng et al. develop a data-driven design for distributed fault detection of dynamic systems using measurements in a complex sensor network. Due to advantages such as high reliability and low communication loads, each sensor node has the ability to execute a fault detection scheme using the average consensus algorithm. The correctness and effectiveness of the proposed scheme are demonstrated in a three-phase flow facility. Next, Sun et al. describe a technique whereby a dynamic parameterization is employed to produce linear, time-varying, parameterized models that equivalently describe a general class of nonlinear systems. The parameters in these models can be identified using an iterative learning least squares technique. The third and final paper in this group, by Lan et al., presents a data-driven policy learning strategy. The authors show how to design controllers for automated vehicles based on vehicle-to-vehicle communications. The learned control policy can be implemented using a prescribed vehicle-to-vehicle communication topology, which can establish a safe, robust, and stable mixed platoon. The final three papers in the special issue consider applications of learning systems. Yang et al. propose neural learning-based adaptive impedance control for a lower limb rehabilitation exoskeleton with flexible joints. In order to improve the control performance and enhance the system robustness, periodic dynamics are considered according to the repetitive motion of the rehabilitation process and are handled by a repetitive learning algorithm. The method studied is used for a full model consisting of both the rigid link and the flexible joints. Next, the authors He and Shen investigate the distributed transient control problem for heating ventilation and air condition systems such that each unit of the large-scale building can consistently maintain the desired temperature. For this problem, a distributed ILC algorithm with a decreasing gain is proposed and analyzed. To accelerate the convergence speed, an adaptation mechanism is introduced to generate an adaptive learning gain sequence. The final paper in the special issue, by Li et al., investigates the robust lateral tracking control problem for autonomous vehicles subject to unmodeled system uncertainties, external disturbances, and input constraints that commonly exist in vehicle dynamics. A robust adaptive learning control approach is proposed based on a parametric vehicle model, where an input-dependent auxiliary system is leveraged to compensate for the influence of the input constraints. This approach is proven to be able to achieve impressive path tracking performances under perturbed and constrained scenarios. Furthermore, the proposed robust adaptive learning technique for tackling the under-actuated dynamics is novel and can also be applied to deal with other generic nonsquare systems.
更多查看译文
关键词
emerging robust,control methods,learning
AI 理解论文
溯源树
样例
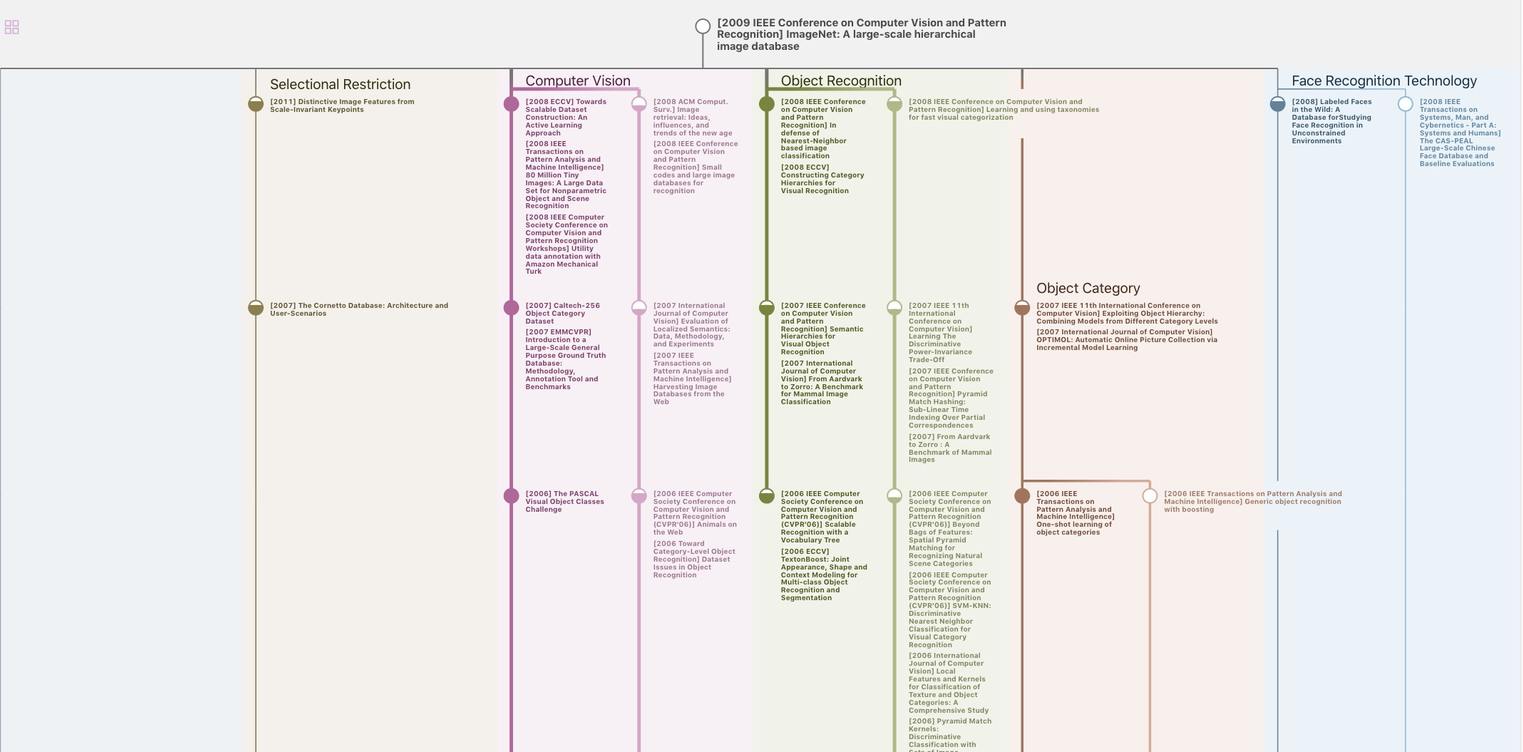
生成溯源树,研究论文发展脉络
Chat Paper
正在生成论文摘要