A GNN-based proactive caching strategy in NDN networks
PEER-TO-PEER NETWORKING AND APPLICATIONS(2023)
摘要
As people spend more time watching and sharing videos online, it is critical to provide users with a satisfactory quality of experience (QoE). Leveraging the in-network caching and named-based routing features in Named Data Networks (NDNs), our paper aims to improve user experience through caching. We propose a graph neural network-gain maximization (GNN-GM) cache placement algorithm. First, we use a GNN model to predict users’ ratings of unviewed videos. Second, we consider the total predicted rating of a video as the gain of the cached video. Third, we propose a cache placement algorithm to maximize the caching gain and actively cache videos. Cache replacement is implemented based on the cache gain ranking of videos, with higher cache gain videos replacing lower cache gain videos. We compare GNN-GM with two state-of-the-art caching strategies, namely the NMF-based caching strategy and GNN-CPP. GNN-GM is also compared with two traditional caching strategies, LCE and LRU, LCE and FIFO. We evaluate the five caching strategies using real-world datasets in a tree network topology, a real-world network topology GEANT, and various random topologies. The experimental results show that our caching policy significantly improves cache hit ratio, latency and server load. Notably, GNN-GM achieves a 25% higher cache hit rate, 5% lower latency and 7% lower server load than GNN-CPP in GEANT.
更多查看译文
关键词
Named data networking,Deep learning,Predict rating,Caching decision,Content placement,Proactive caching
AI 理解论文
溯源树
样例
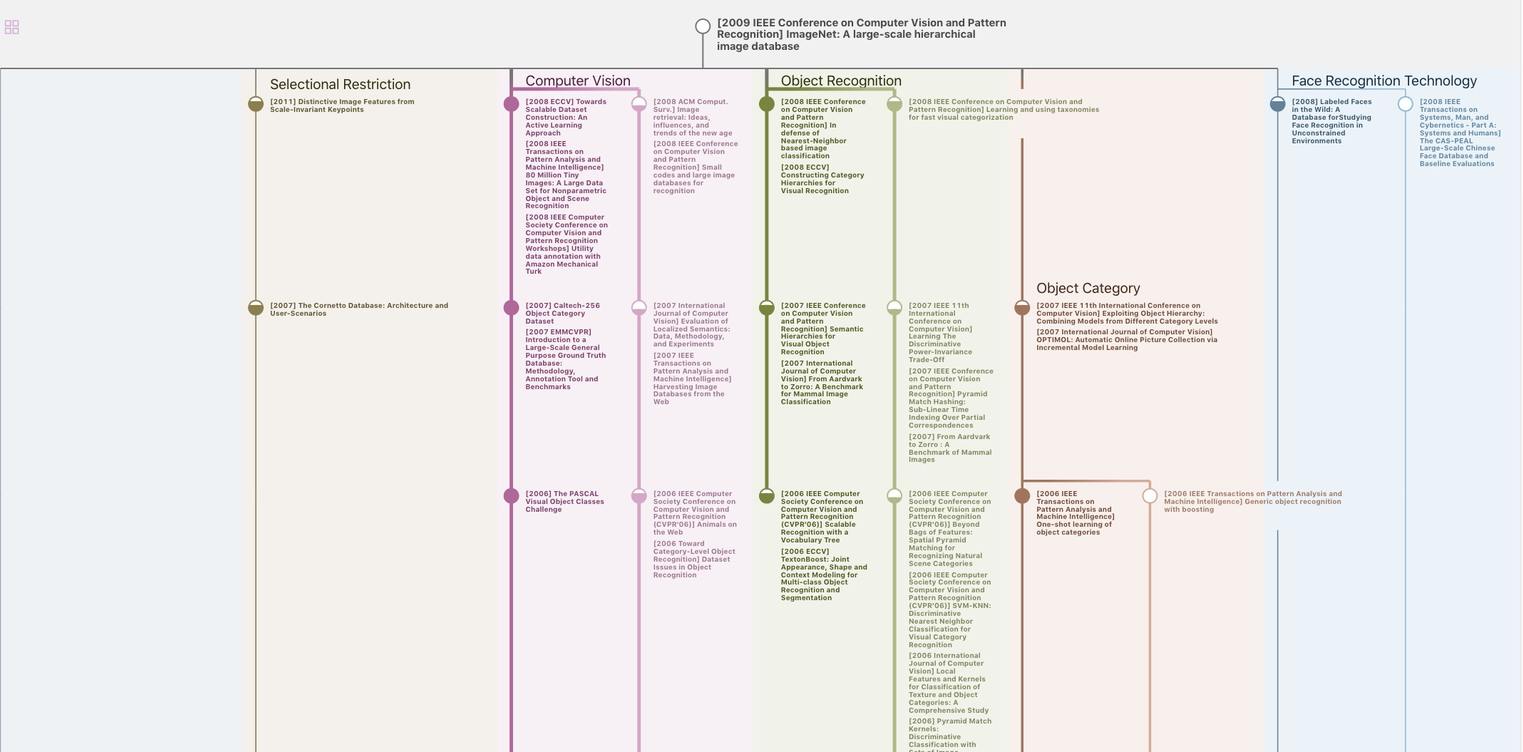
生成溯源树,研究论文发展脉络
Chat Paper
正在生成论文摘要