Transfer learning based on dynamic time warping algorithms to improve qualitative analysis and quantitative prediction of rocks over multiple LIBS instruments
JOURNAL OF ANALYTICAL ATOMIC SPECTROMETRY(2023)
摘要
Laser-induced breakdown spectroscopy (LIBS) instruments have gradually become an attractive technical tool in the field of rock chemical composition analysis due to their advantages of simplicity, rapid detection and simultaneous multi-element analysis. However, the analysis performance of LIBS instruments is prone to being affected by factors such as the changes in the measurement environment and differences between instruments. It is difficult to share the prediction models even among the same type of instruments. Thus, the generalization capability of LIBS analysis instruments is limited. Herein, we propose a transfer learning method based on the dynamic time warping (DTW) algorithm for constructing a transfer model that can correct the secondary instrumental spectrum. The method was tested by using 49 national standard samples and compared with the piecewise direct standardization (PDS) algorithm. The transfer learning method based on DTW was superior to the PDS algorithm in both the classification prediction of lithology and the quantitative prediction of elements. However, it was found that transfer learning could not fully correct the spectra in the quantitative predictions. Hence, the Pearson coefficient was also introduced in this work to select features. Eventually, the average prediction coefficient of determination of the model improved from 0.6844 to 0.9263, and the average root mean square error of prediction was reduced from 10.5621 to 2.3831. The result shows that the transfer learning method, combined with the feature selection and DTW, can not only enhance the potential of the model for a wide range of applications, but also simplify the model and improve the performance of the prediction.
更多查看译文
关键词
transfer learning,dynamic time,quantitative prediction
AI 理解论文
溯源树
样例
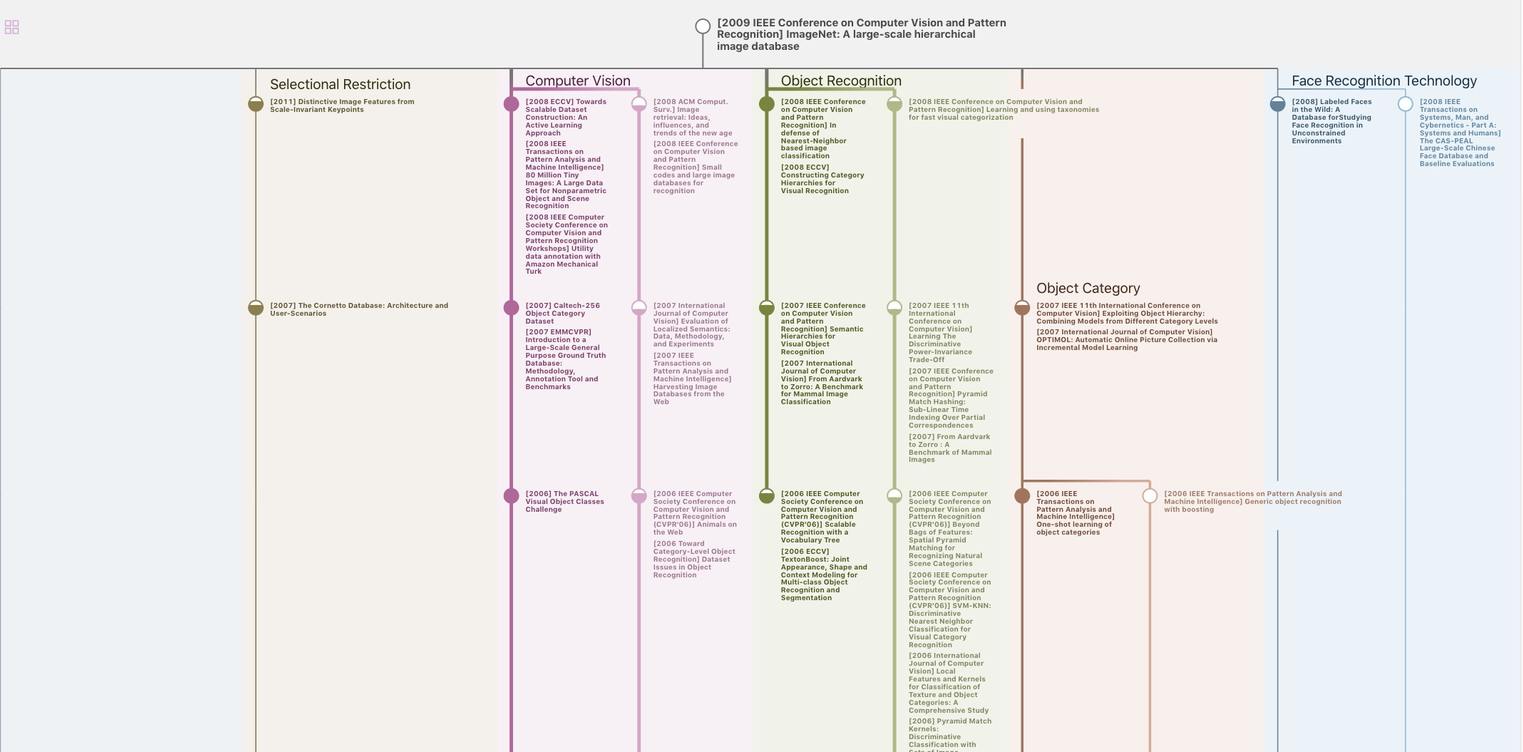
生成溯源树,研究论文发展脉络
Chat Paper
正在生成论文摘要