ChroSegNet: An Attention-Based Model for Chromosome Segmentation with Enhanced Processing
APPLIED SCIENCES-BASEL(2023)
摘要
In modern medical diagnosis, the karyotype analysis for human chromosome is clinically significant for the diagnosis and treatment of genetic diseases. In such an analysis, it is critically important to segment the banded chromosomes. Chromosome segmentation, however, is technically challenging due to the variable chromosome features, the complex background noise, and the uneven image quality of the chromosome images. Owing to these technical challenges, the existing deep-learning-based algorithms would have severe overfitting problems and are ineffective in the segmentation task. In this paper, we propose a novel chromosome segmentation model with our enhanced chromosome processing, namely ChroSegNet. First, we develop enhanced chromosome processing techniques to realize the quality and quantity enhancement of the chromosome data, leading to the chromosome segmentation dataset for our subsequent network training. Second, we propose our novel chromosome segmentation model "ChroSegNet" based on U-Net. According to the characteristics of chromosome data, we have not only improved the baseline structure but also incorporate the hybrid attention module to ChroSegNet, which can extract the key feature information and location information of chromosome. Finally, we evaluated ChroSegNet on our chromosome segmentation dataset and obtained the MPA of 93.31% and the F1-score of 92.99%. Experimental results show that ChroSegNet not only outperforms the representative segmentation models in chromosome segmentation performance but also has a lightweight model structure. We believe that our proposed ChroSegNet is highly promising in future applications of genetic measurement and diagnosis.
更多查看译文
关键词
deep learning,chromosome segmentation,U-Net,attention mechanism,enhanced processing
AI 理解论文
溯源树
样例
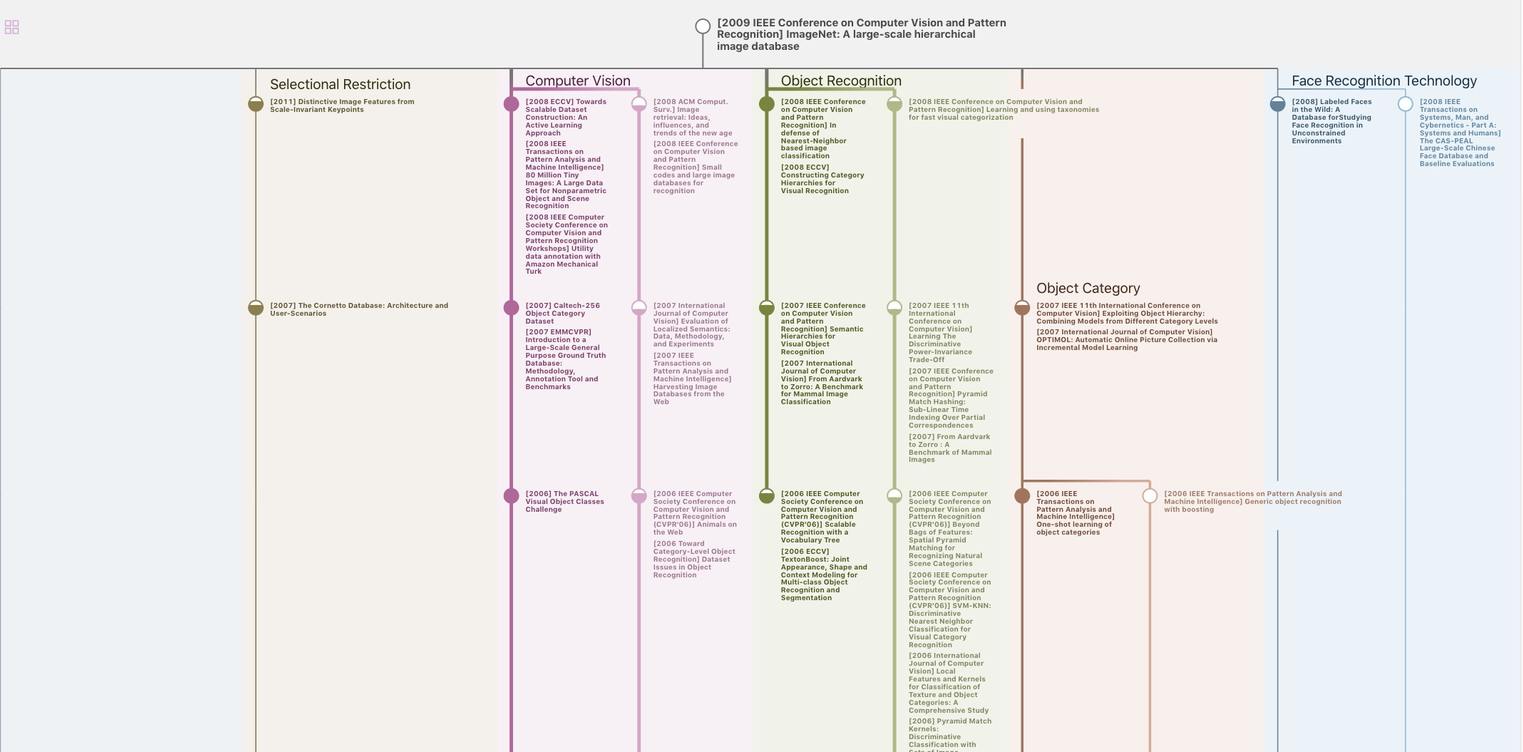
生成溯源树,研究论文发展脉络
Chat Paper
正在生成论文摘要