A Multi-Scale Traffic Object Detection Algorithm for Road Scenes Based on Improved YOLOv5
ELECTRONICS(2023)
摘要
Object detection in road scenes is a task that has recently become popular and it is also an important part of intelligent transportation systems. Due to the different locations of cameras in the road scenes, the size of the traffic objects captured varies greatly, which imposes a burden on the network optimization. In addition, in some dense traffic scenes, the size of the traffic objects captured is extremely small and it is easy to miss detection and to encounter false detection. In this paper, we propose an improved multi-scale YOLOv5s algorithm based on the YOLOv5s algorithm. In detail, we add a detection head for extremely small objects to the original YOLOv5s model, which significantly improves the accuracy in detecting extremely small traffic objects. A content-aware reassembly of features (CARAFE) module is introduced in the feature fusion part to enhance the feature fusion. A new SPD-Conv CNN Module is introduced instead of the original convolutional structure to enhance the overall computational efficiency of the model. Finally, the normalization-based attention module (NAM) is introduced, allowing the model to focus on more useful information during training and significantly improving detection accuracy. The experimental results demonstrate that compared with the original YOLOv5s algorithm, the detection accuracy of the multi-scale YOLOv5s model proposed in this paper is improved by 7.1% on the constructed diverse traffic scene datasets. The improved multi-scale YOLOv5s algorithm also maintains the highest detection accuracy among the current mainstream object detection algorithms and is superior in accomplishing the task of detecting traffic objects in complex road scenes.
更多查看译文
关键词
road scenes,object detection,YOLOv5,multi-scale,attention mechanism
AI 理解论文
溯源树
样例
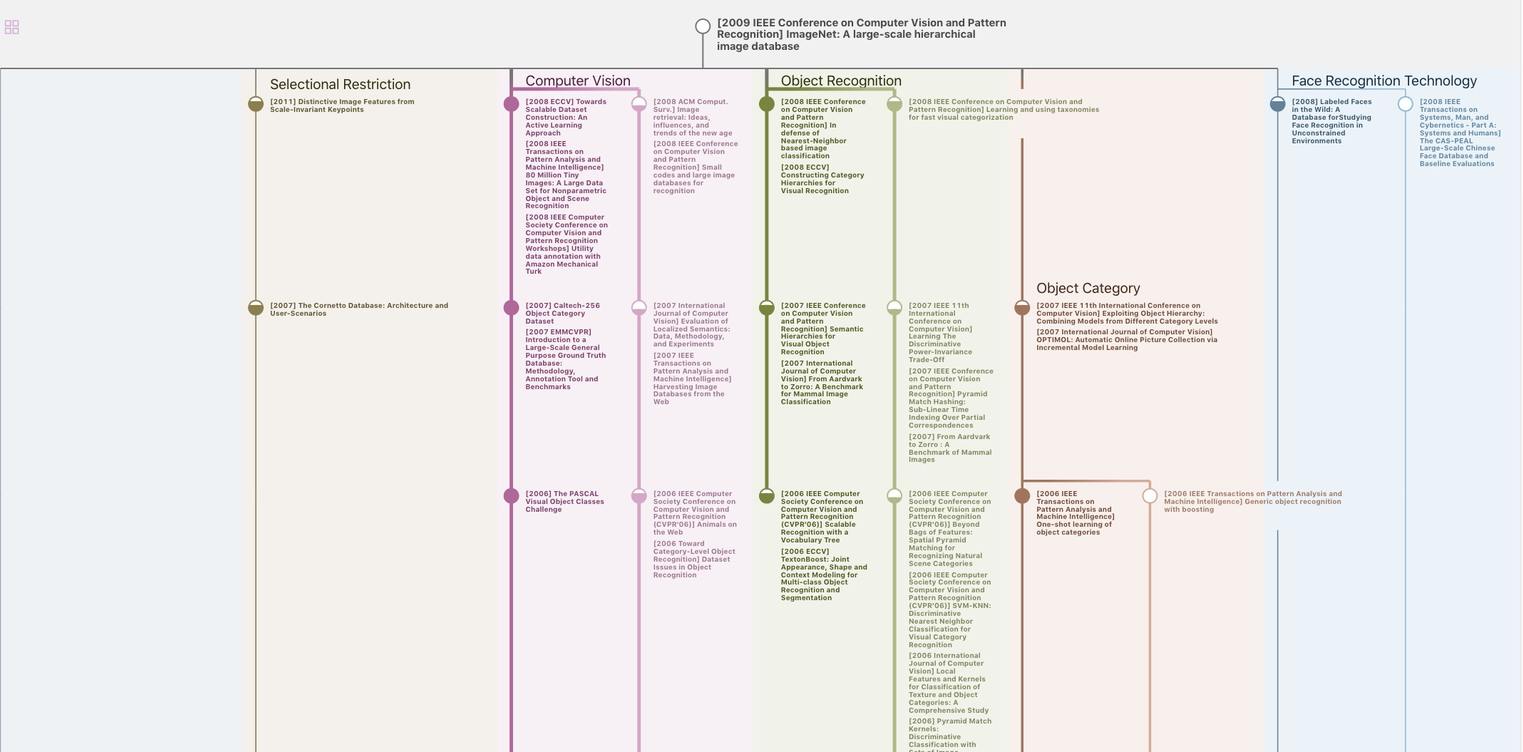
生成溯源树,研究论文发展脉络
Chat Paper
正在生成论文摘要