Comparative Analysis of Statistical and Machine Learning Techniques for Rice Yield Forecasting for Chhattisgarh, India
SUSTAINABILITY(2023)
摘要
Crop yield forecasting before harvesting is critical for the creation, implementation, and optimization of policies related to food safety as well as for agro-product storage and marketing. Crop growth and development are influenced by the weather. Therefore, models using weather variables can provide reliable predictions of crop yields. It can be tough to select the best crop production forecasting model. Therefore, in this study, five alternative models, viz., stepwise multiple linear regression (SMLR), an artificial neural network (ANN), the least absolute shrinkage and selection operator (LASSO), an elastic net (ELNET), and ridge regression, were compared in order to discover the best model for rice yield prediction. The outputs from individual models were used to build ensemble models using the generalized linear model (GLM), random forest (RF), cubist and ELNET methods. For the previous 21 years, historical rice yield statistics and meteorological data were collected for three districts under three separate agro-climatic zones of Chhattisgarh, viz., Raipur in the Chhattisgarh plains, Surguja in the northern hills, and Bastar in the southern plateau. The models were calibrated using 80% of these datasets, and the remaining 20% was used for the validation of models. The present study concluded that for rice crop yield forecasting, the performance of the ANN was good for the Raipur (R-cal(2) = 1, Rv(val)(2 ) = 1 and RMSEcal = 0.002, RMSEval = 0.003) and Surguja (R-cal(2) = 1, R-val(2 )= 0.99 and RMSE(ca)l = 0.004, RMSEval = 0.214) districts as compared to the other models, whereas for Bastar, ELNET (R-cal(2) = 90, R-val(2 ) = 0.48) and LASSO (R-cal(2) = 93, R-val(2 )= 0.568) performed better. The performance of the ensemble model was better compared to the individual models. For Raipur and Surguja, the performance of all the ensemble methods was comparable, whereas for Bastar, random forest (RF) performed better, with R-2 = 0.85 and 0.81 for calibration and validation, respectively, as compared to the GLM, cubist, and ELNET approach.
更多查看译文
关键词
yield forecasting,SMLR,ANN,LASSO,ELNET,ridge regression
AI 理解论文
溯源树
样例
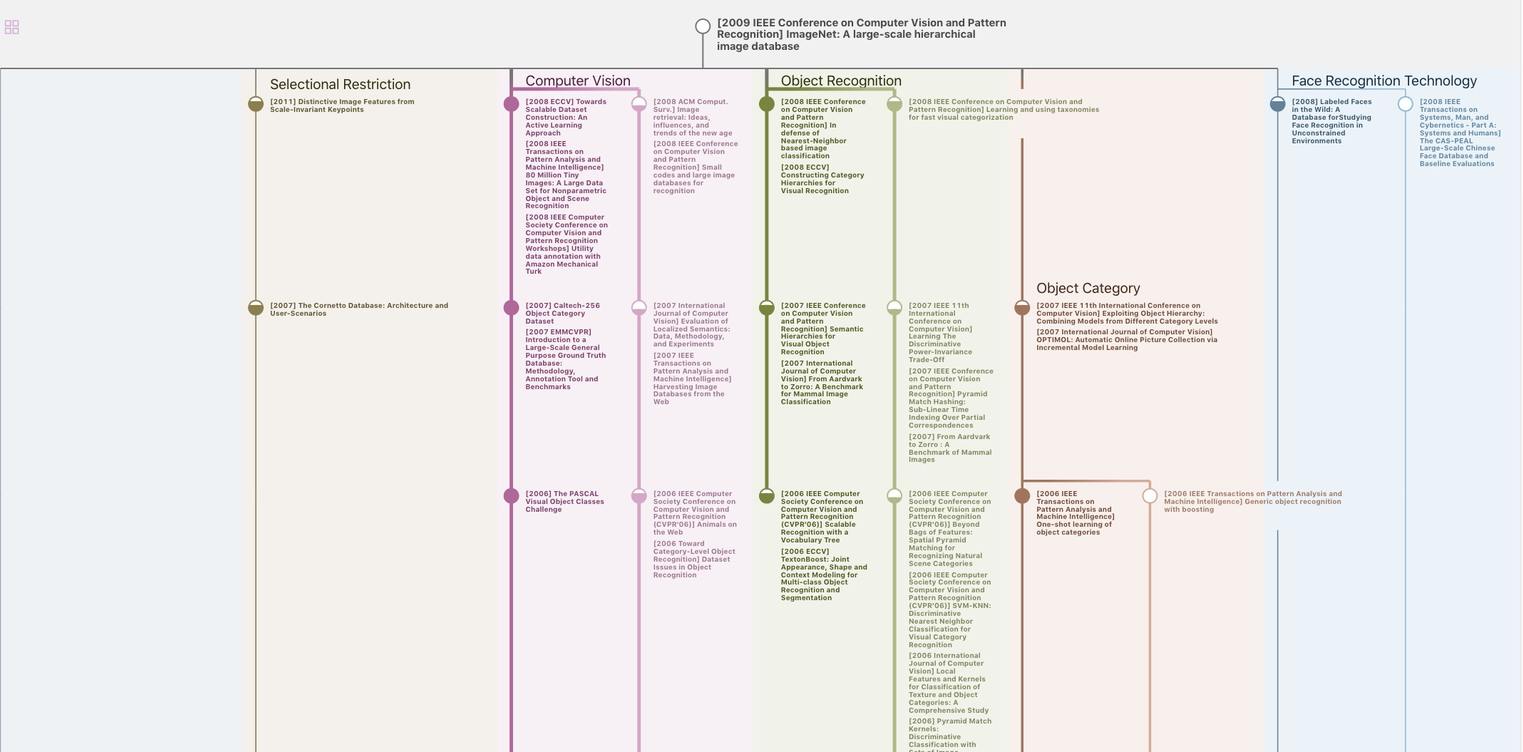
生成溯源树,研究论文发展脉络
Chat Paper
正在生成论文摘要