MS3D: Leveraging Multiple Detectors for Unsupervised Domain Adaptation in 3D Object Detection
CoRR(2023)
摘要
We introduce Multi-Source 3D (MS3D), a new self-training pipeline for unsupervised domain adaptation in 3D object detection. Despite the remarkable accuracy of 3D detectors, they often overfit to specific domain biases, leading to suboptimal performance in various sensor setups and environments. Existing methods typically focus on adapting a single detector to the target domain, overlooking the fact that different detectors possess distinct expertise on different unseen domains. MS3D leverages this by combining different pre-trained detectors from multiple source domains and incorporating temporal information to produce high-quality pseudo-labels for fine-tuning. Our proposed Kernel-Density Estimation (KDE) Box Fusion method fuses box proposals from multiple domains to obtain pseudo-labels that surpass the performance of the best source domain detectors. MS3D exhibits greater robustness to domain shifts and produces accurate pseudo-labels over greater distances, making it well-suited for high-to-low beam domain adaptation and vice versa. Our method achieved state-of-the-art performance on all evaluated datasets, and we demonstrate that the choice of pre-trained source detectors has minimal impact on the self-training result, making MS3D suitable for real-world applications.
更多查看译文
关键词
Object Detection,Domain Adaptation,Multiple Detection,3D Detection,3D Object Detection,Domain Shift,Target Domain,Source Domain,Source Dataset,Unseen Domains,Probability Density Function,Intersection Over Union,Multiple Datasets,Point Cloud,Bounding Box,Confidence Score,Detection Of Types,Target Dataset,Static Objects,High Beam,Source Detector,Single Box,Dynamic Objects,Unlabeled Target Domain,Non-maximum Suppression,Domain Gap,Scan Pattern,Prediction Box,Detection Boxes,Object Detection Approaches
AI 理解论文
溯源树
样例
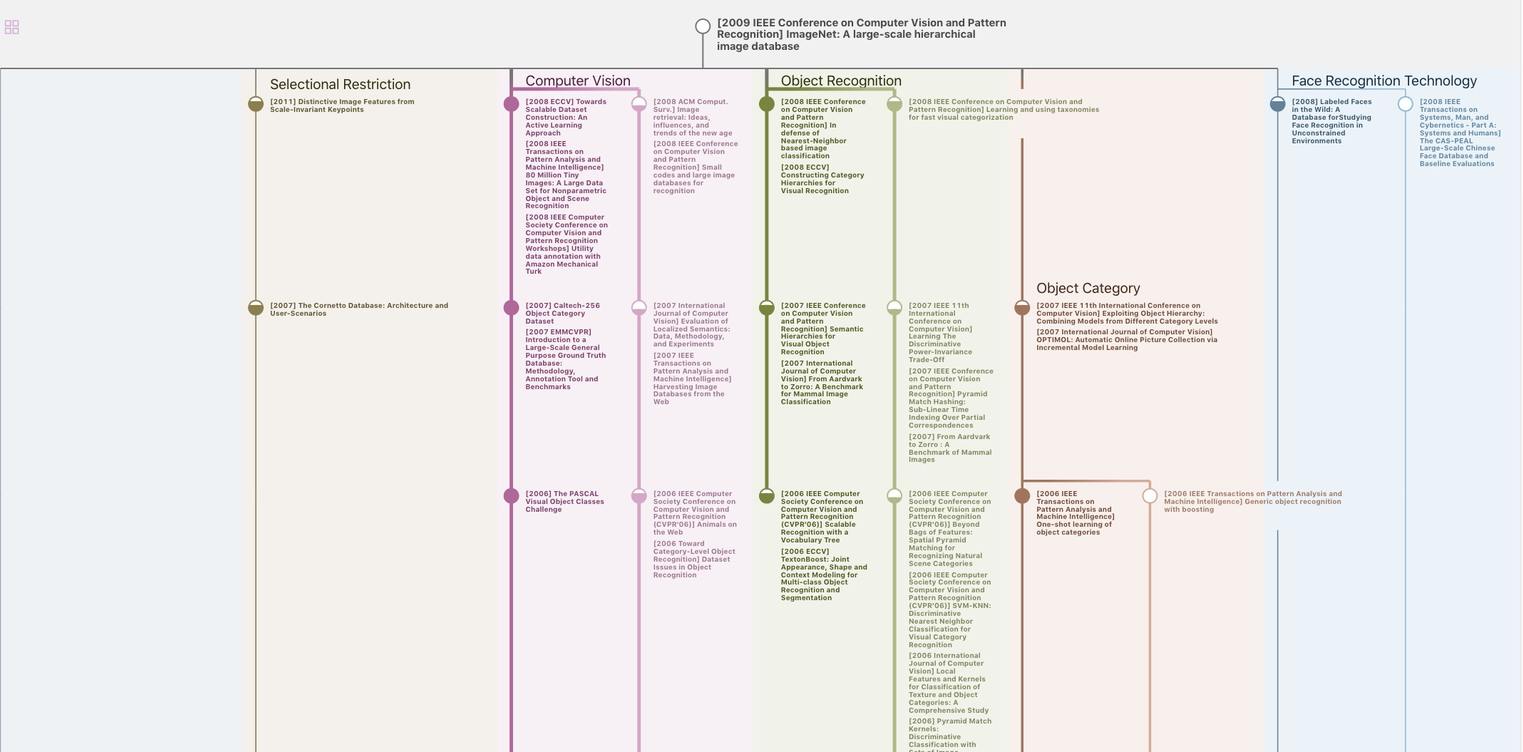
生成溯源树,研究论文发展脉络
Chat Paper
正在生成论文摘要