Physics-aware Roughness Optimization for Diffractive Optical Neural Networks
CoRR(2023)
摘要
As a representative next-generation device/circuit technology beyond CMOS, diffractive optical neural networks (DONNs) have shown promising advantages over conventional deep neural networks due to extreme fast computation speed (light speed) and low energy consumption. However, there is a mismatch, i.e., significant prediction accuracy loss, between the DONN numerical modelling and physical optical device deployment, because of the interpixel interaction within the diffractive layers. In this work, we propose a physics-aware diffractive optical neural network training framework to reduce the performance difference between numerical modeling and practical deployment. Specifically, we propose the roughness modeling regularization in the training process and integrate the physics-aware sparsification method to introduce sparsity to the phase masks to reduce sharp phase changes between adjacent pixels in diffractive layers. We further develop 2π periodic optimization to reduce the roughness of the phase masks to preserve the performance of DONN. Experiment results demonstrate that, compared to state-of-the-arts, our physics-aware optimization can provide 35.7%, 34.2%, 28.1%, and 27.3% reduction in roughness with only accuracy loss on MNIST, FMNIST, KMNIST, and EMNIST, respectively.
更多查看译文
关键词
Diffractive optical neural network, Weight Sparsification, Roughness Modeling
AI 理解论文
溯源树
样例
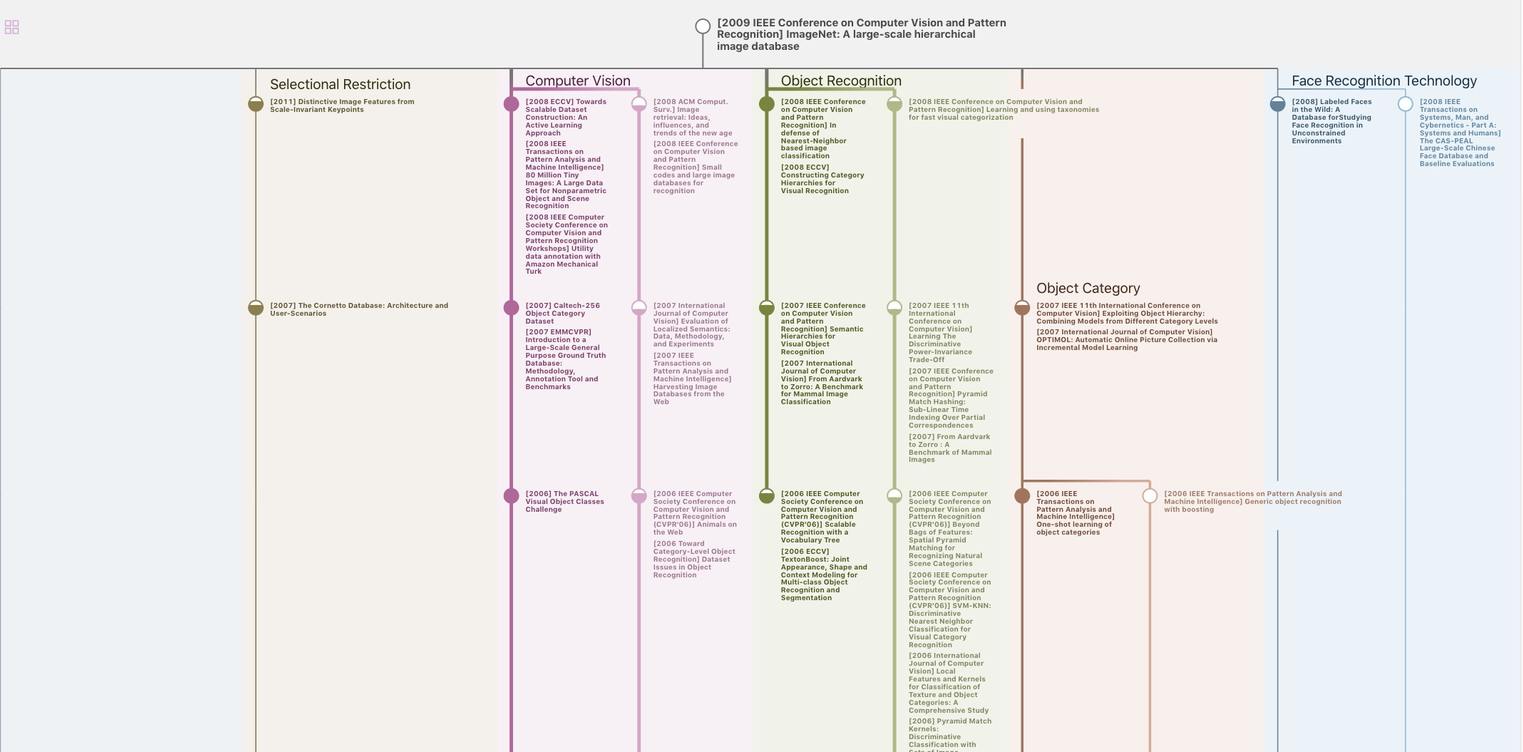
生成溯源树,研究论文发展脉络
Chat Paper
正在生成论文摘要