RPTQ: Reorder-based Post-training Quantization for Large Language Models
CoRR(2023)
摘要
Large-scale language models (LLMs) have demonstrated outstanding performance on various tasks, but their deployment poses challenges due to their enormous model size. In this paper, we identify that the main challenge in quantizing LLMs stems from the different activation ranges between the channels, rather than just the issue of outliers.We propose a novel reorder-based quantization approach, RPTQ, that addresses the issue of quantizing the activations of LLMs. RPTQ rearranges the channels in the activations and then quantizing them in clusters, thereby reducing the impact of range difference of channels. In addition, we reduce the storage and computation overhead by avoiding explicit reordering. By implementing this approach, we achieved a significant breakthrough by pushing LLM models to 3 bit activation for the first time.
更多查看译文
关键词
language,models,reorder-based,post-training
AI 理解论文
溯源树
样例
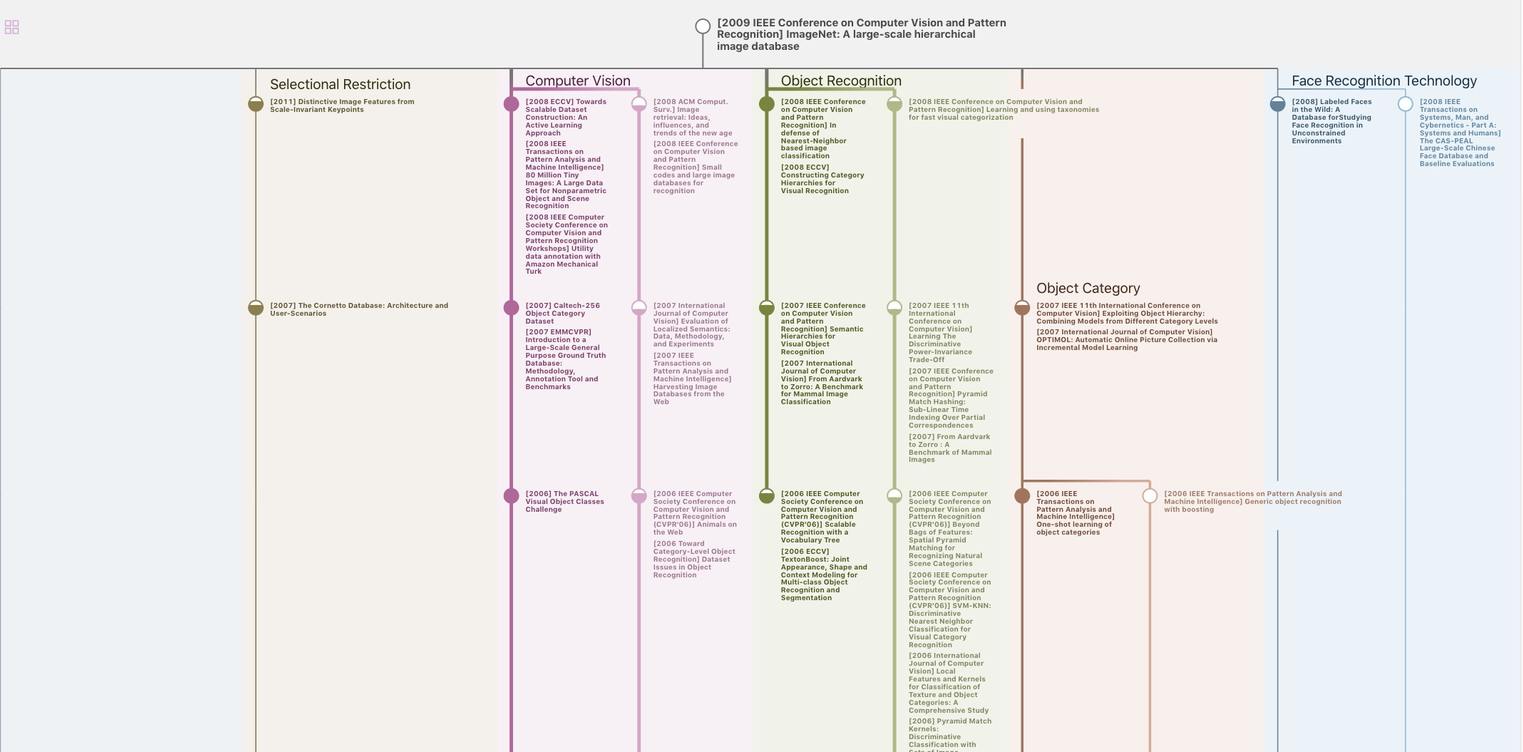
生成溯源树,研究论文发展脉络
Chat Paper
正在生成论文摘要