Automated Tumor Segmentation and Brain Tissue Extraction from Multiparametric MRI of Pediatric Brain Tumors: A Multi-Institutional Study.
medRxiv : the preprint server for health sciences(2023)
摘要
The current response assessment in pediatric brain tumors (PBTs) is currently based on bidirectional or 2D measurements, which underestimate the size of non-spherical and complex PBTs in children compared to volumetric or 3D methods. There is a need for development of automated methods to reduce manual burden and intra- and inter-rater variability to segment tumor subregions and assess volumetric changes. Most currently available automated segmentation tools are developed on adult brain tumors, and therefore, do not generalize well to PBTs that have different radiological appearances. To address this, we propose a deep learning (DL) auto-segmentation method that shows promising results in PBTs, collected from a publicly available large-scale imaging dataset (Children's Brain Tumor Network; CBTN) that comprises multi-parametric MRI scans of multiple PBT types acquired across multiple institutions on different scanners and protocols. As a complementary to tumor segmentation, we propose an automated DL model for brain tissue extraction.
更多查看译文
关键词
deep learning,magnetic resonance imaging,pediatric brain tumor,skull-stripping,tumor segmentation
AI 理解论文
溯源树
样例
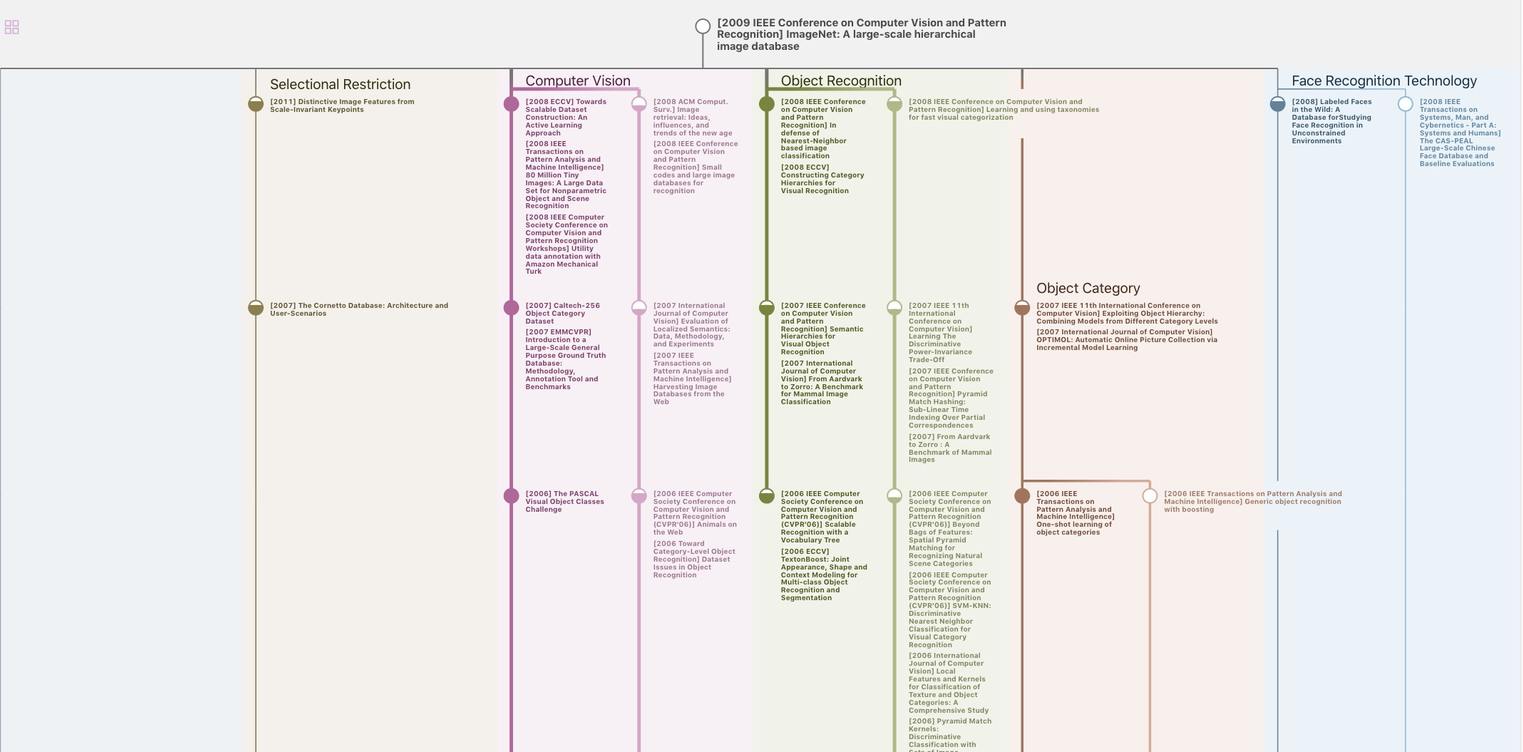
生成溯源树,研究论文发展脉络
Chat Paper
正在生成论文摘要