A Bayesian Deep Learning Approach With Convolutional Feature Engineering to Discriminate Cyber-Physical Intrusions in Smart Grid Systems.
IEEE Access(2023)
摘要
The emergence of cyber-physical smart grid (CPSG) systems has revolutionized the traditional power grid by enabling the bidirectional energy flow between consumers and utilities. However, due to escalated information exchange between the end-users, it has posed a greater challenge to the cyber security mechanisms for the communication networks at the cyber and physical planes. To address these challenges, we propose a Bayesian approach integrated with deep convolutional neural networks (CNN-Bayesian). While, the Bayesian component is used to discriminate cyber-physical intrusions from the normal events in the binary and multi-class events. CNN layers are utilized to handle the high-dimensional feature space prior to the intrusions classification task. The proposed method is validated using real-time Industrial control systems (ICS) dataset against the standard deep learning-based classification methods such as recurrent neural networks (RNN) and long-short term memory (LSTM). From the experimental results, it can be inferred that the proposed CNN-Bayesian method outperforms the existing benchmark classification methods to discriminate intrusions in CPSG systems using evaluation metrics such as accuracy, precision, recall, and F 1-score.
更多查看译文
关键词
Feature extraction,Convolutional neural networks,Bayes methods,Uncertainty,Probabilistic logic,Neural networks,Classification algorithms,Deep learning,Intrusion detection,Smart grids,SCADA systems,Bayesian inference,cybersecurity,deep learning,intrusion-detection systems,SCADA,smart grid
AI 理解论文
溯源树
样例
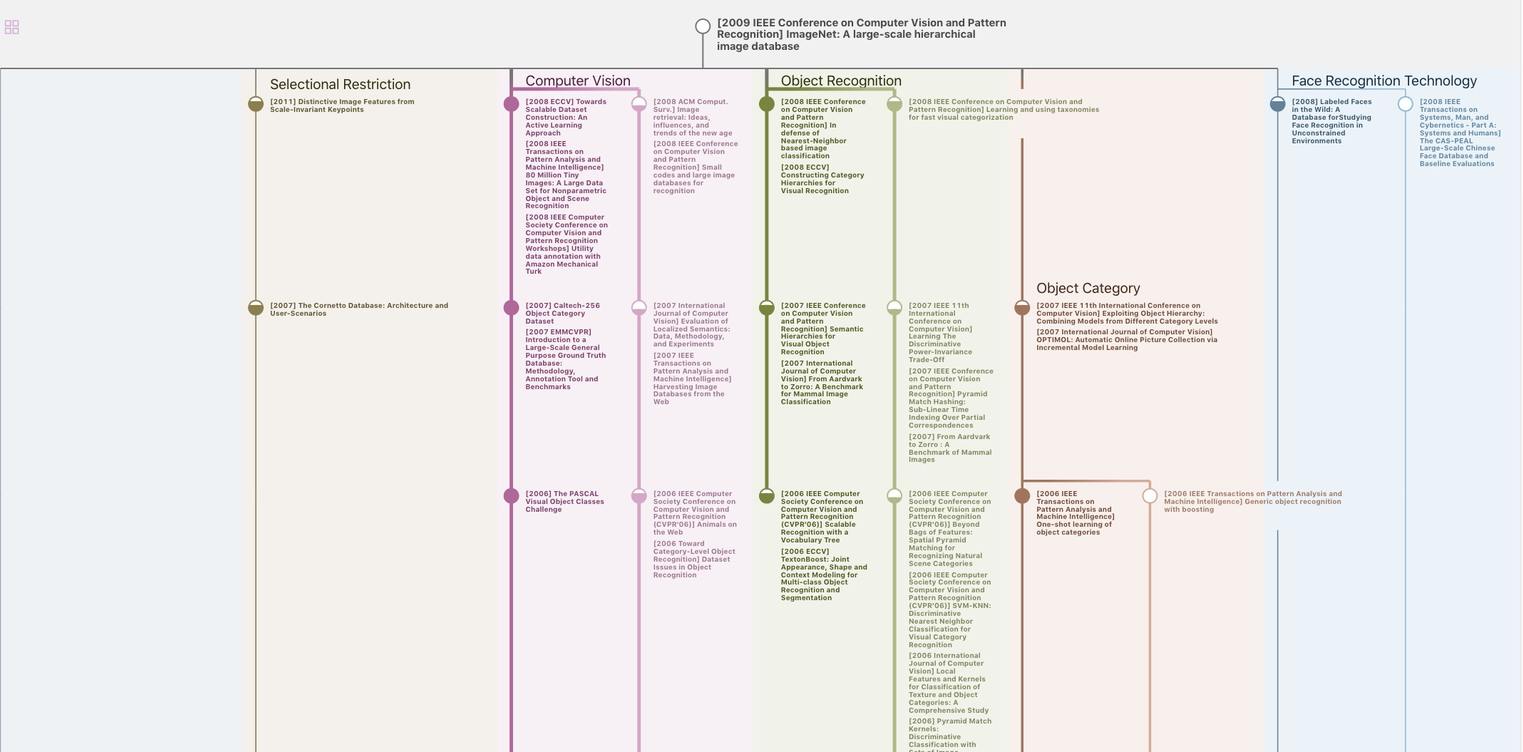
生成溯源树,研究论文发展脉络
Chat Paper
正在生成论文摘要