IK-EMOViz: An Interactive Knowledge-Based Evolutionary Multi-objective Optimization Framework.
EMO(2023)
摘要
The knowledge and intuition of experienced users for practical optimization problems are often underutilized in academic research. Such knowledge, formulated as inter-variable relationships, can assist an optimization algorithm in finding good solutions faster. User-provided information can be utilized at the beginning of the optimization, or during the optimization in an interactive fashion. In this paper, we propose IK-EMOViz, a software framework to allow discovery and use of knowledge from and to an EMO algorithm interactively. Key knowledge common to current non-dominated solutions are extracted using rule learning methods and shared with the decision-makers (DMs) through a easy-to-comprehend visualization tool. Learned knowledge are then filtered and vetted by DMs are communicated to the EMO algorithm using the same visualization tool. EMO algorithm then processes the filtered knowledge and integrates them with its search operators. Repeated such interactions have resulted in faster convergence to the final trade-off set on a large-scale engineering design problem. In addition, the effect of asynchronous and synchronous interactivity is also evaluated to make the proposed interactive optimization procedure more pragmatic.
更多查看译文
关键词
Interactive optimization, Knowledge extraction, Graphical user interface, Multi-objective optimization
AI 理解论文
溯源树
样例
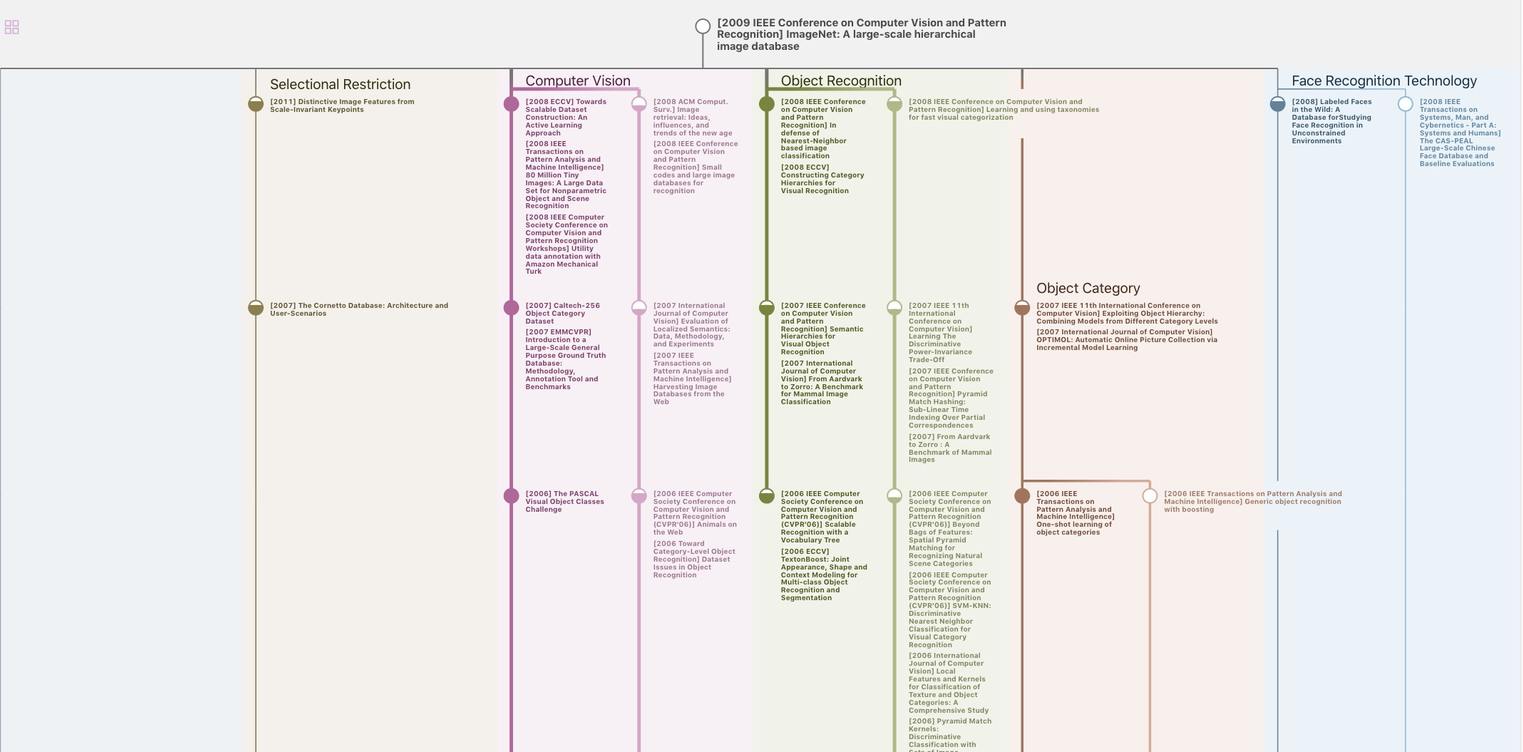
生成溯源树,研究论文发展脉络
Chat Paper
正在生成论文摘要