A heterogeneous stacking ensemble-based security framework for detecting phishing attacks.
NCC(2023)
摘要
This paper proposes a heterogeneous stacking ensemble-based security framework comprising three different base-level classifiers, and a single meta-level classifier for detecting phishing attacks. The proposed framework initially extracts 24 different URL based and 20 distinct web-page based feature from a given URL corpus. These features are then used to create feature vectors for training the base-level classifiers of the proposed framework. The trained base-level classifiers are then evaluated on the unseen feature vectors from the test set. The output vectors produced by the trained base-level classifiers are then combined into a dataframe object, and used for training and evaluating a fully connected neural network(FCNN) based meta-level classifier. The trained meta-level classifier makes the final prediction about whether the web-pages corresponding to given URLs are benign or malicious(phishing). Experimental results on two benchmark datasets namely, PhishTank & UNB datasets show that the proposed framework achieves an accuracy of 99% on the binary class PhishTank & UNB datasets, while it achieves an accuracy of 98% on multiclass UNB dataset. The proposed framework is also shown to outperform other similar phishing attack detection frameworks proposed in the literature.
更多查看译文
关键词
Phishing attacks,stacking ensemble-based classifier,PhishTank & UNB datasets
AI 理解论文
溯源树
样例
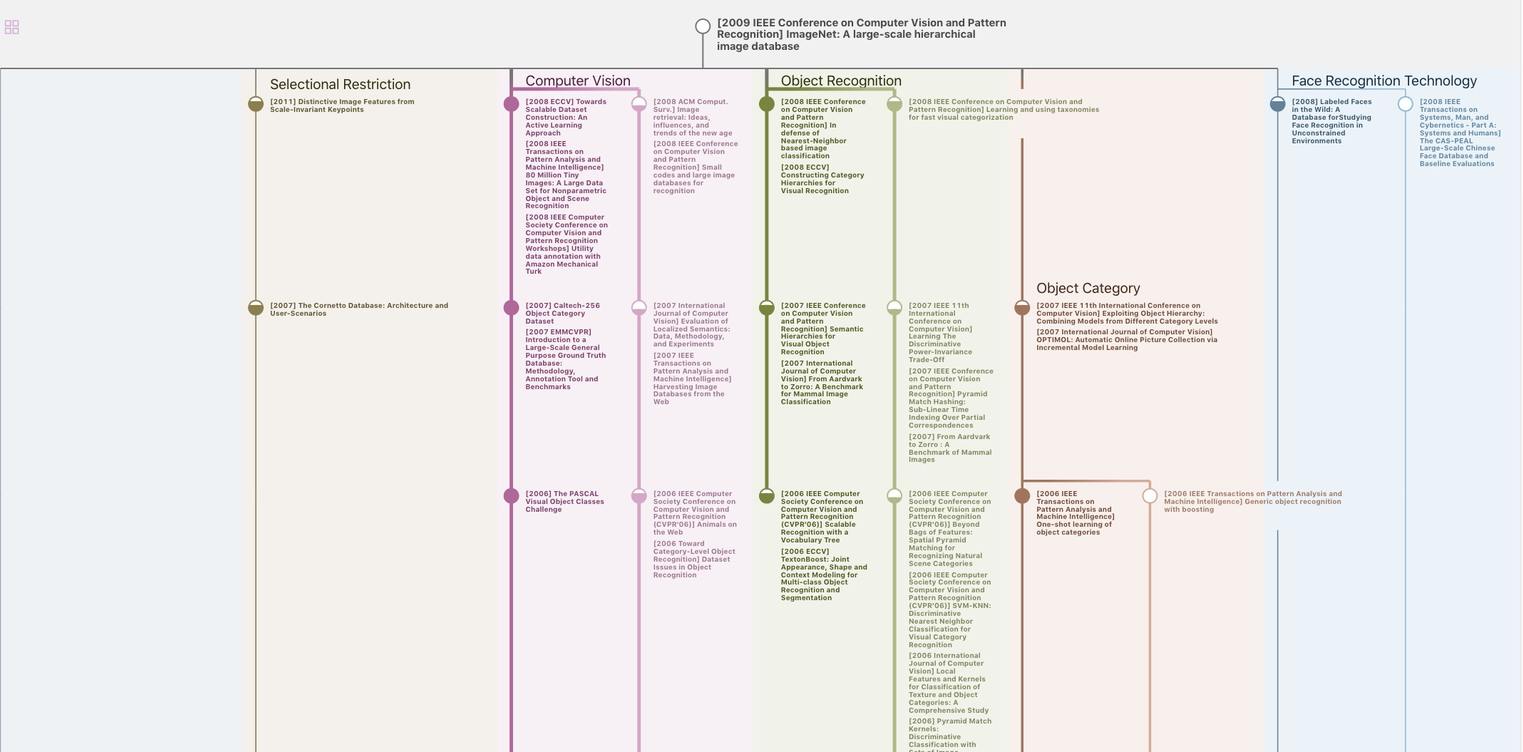
生成溯源树,研究论文发展脉络
Chat Paper
正在生成论文摘要