Meditative State Classification Using Neuronal Multi-IMF Band Power and Complexity Features.
NCC(2023)
摘要
The incredible complexity of physiological signals, along with the awareness that physiological time series contains hidden information, has sparked a surge in the interest to conduct neuroscientific research. The human brain is one of the most significant instances of intricate physiologic oscillations as recorded through Electroencephalogram (EEG). In this article, we propose a methodology for assessing the EEG chaotic levels by decomposing EEG signals into Intrinsic Mode Functions (IMFs) and extracting features like Sample Entropy (SE) and Hurst Exponent (HE). We then demonstrate how various mediation styles affect the EEG chaotic levels and also provide a framework for classifying meditative states. We conduct our research on two different types of meditation - Himalayan Yoga (HT) and Hare Krishna mantra meditation (HKT). HKT is an in-house study of a group of 16 experienced meditators who participated in a two-week mantra meditation practice. Corresponding to HT, there is a publicly accessible online dataset of 16 experienced meditators. We observed that when compared to the control group (CTR), HKT meditation demonstrated a significant increase in SE and a significantly higher drop in HE for the alpha and gamma bands, indicating enhanced human brain complexity and decreased predictability, causing the brain to be more active. We tested multiple ML classifiers with HE, SE, and Band-power (BP) features and discovered that by utilizing BP features, we could reach 100% accuracy in classifying meditative and non meditative states in both traditions ((HT) and (HKT)).
更多查看译文
关键词
Meditation,EEG,Empirical Mode Decomposition (EMD),Intrinsic Mode Functions (IMFs),Feature Extraction,Sample Entropy (SE),Hurst Exponent (HE),Band Power (BP) Classification,Machine Learning (ML)
AI 理解论文
溯源树
样例
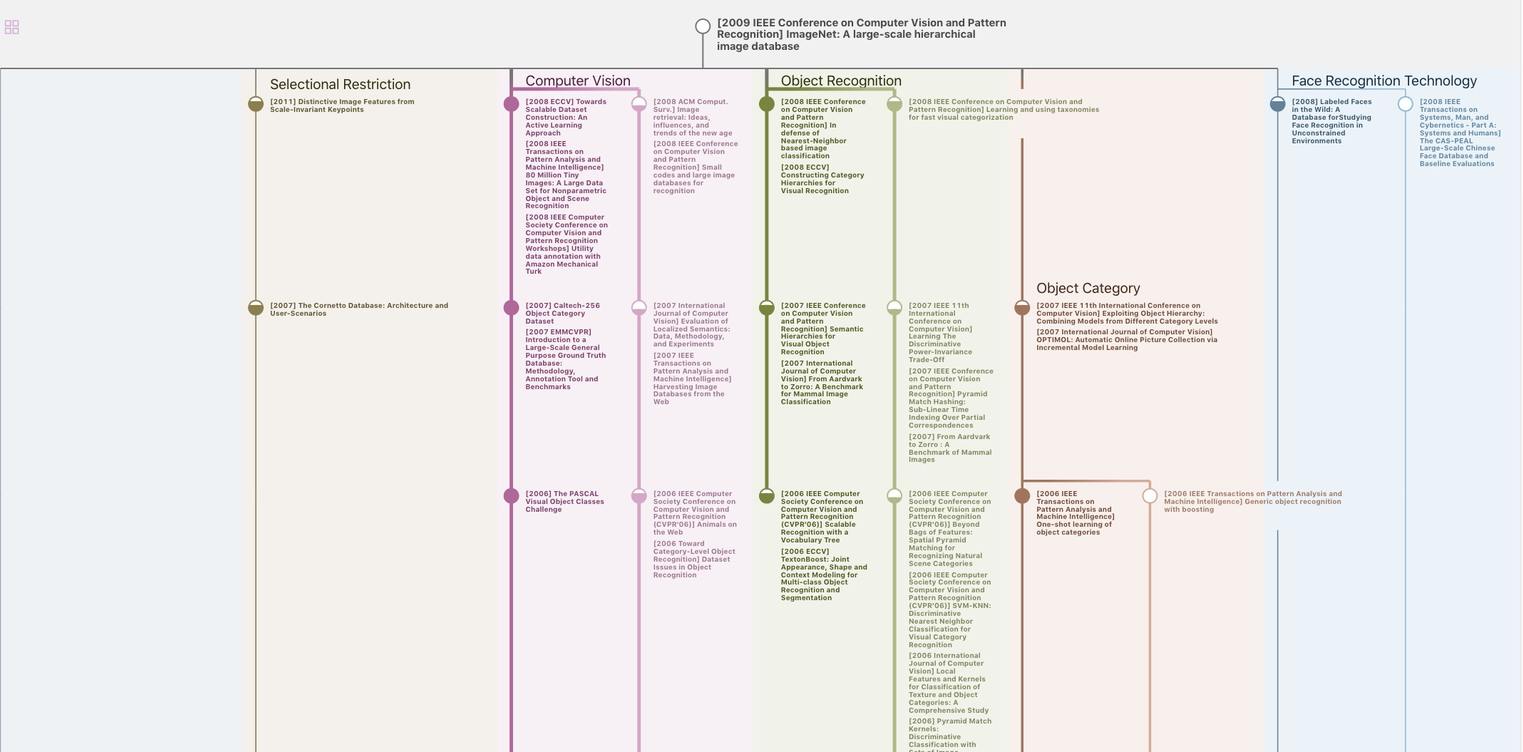
生成溯源树,研究论文发展脉络
Chat Paper
正在生成论文摘要