A deep semi-dense compression network for reinforcement learning based on information theory.
Neurocomputing(2023)
摘要
Although reinforcement learning (RL) can solve complex tasks after training, it’s difficult to extend trained agents to target environments with perturbations. This lack of generalization ability has caused the dilemma of large-scale application of RL. Rich neural networks have brought huge improvements to deep learning, but these aren’t adapted to RL and even bring negative effects. Therefore, RL algorithms have few choices of neural network, which greatly limits the representation and generalization ability of RL. To overcome these limitations, we propose a deep semi-dense compression network (DSCN) to improve RL generalization ability. First, we perform a structural extension on a general network model for RL. Then based on the information theory, we propose a semi-dense connection to enhance the neural information flow (NIF) of initial features. Finally, drawing on the ideas of course learning, we propose a channel compression approach to filter the redundant information of the deep network. In addition, we innovatively extend the experimental environment and evaluation metrics of the existing platform, which can fully evaluate the performance of DSCN. The experimental results show that our model achieves stable and significant generalization performance improvement.
更多查看译文
关键词
Reinforcement learning,Generalization,Deep semi-dense compression network,Information theory,Channel compression
AI 理解论文
溯源树
样例
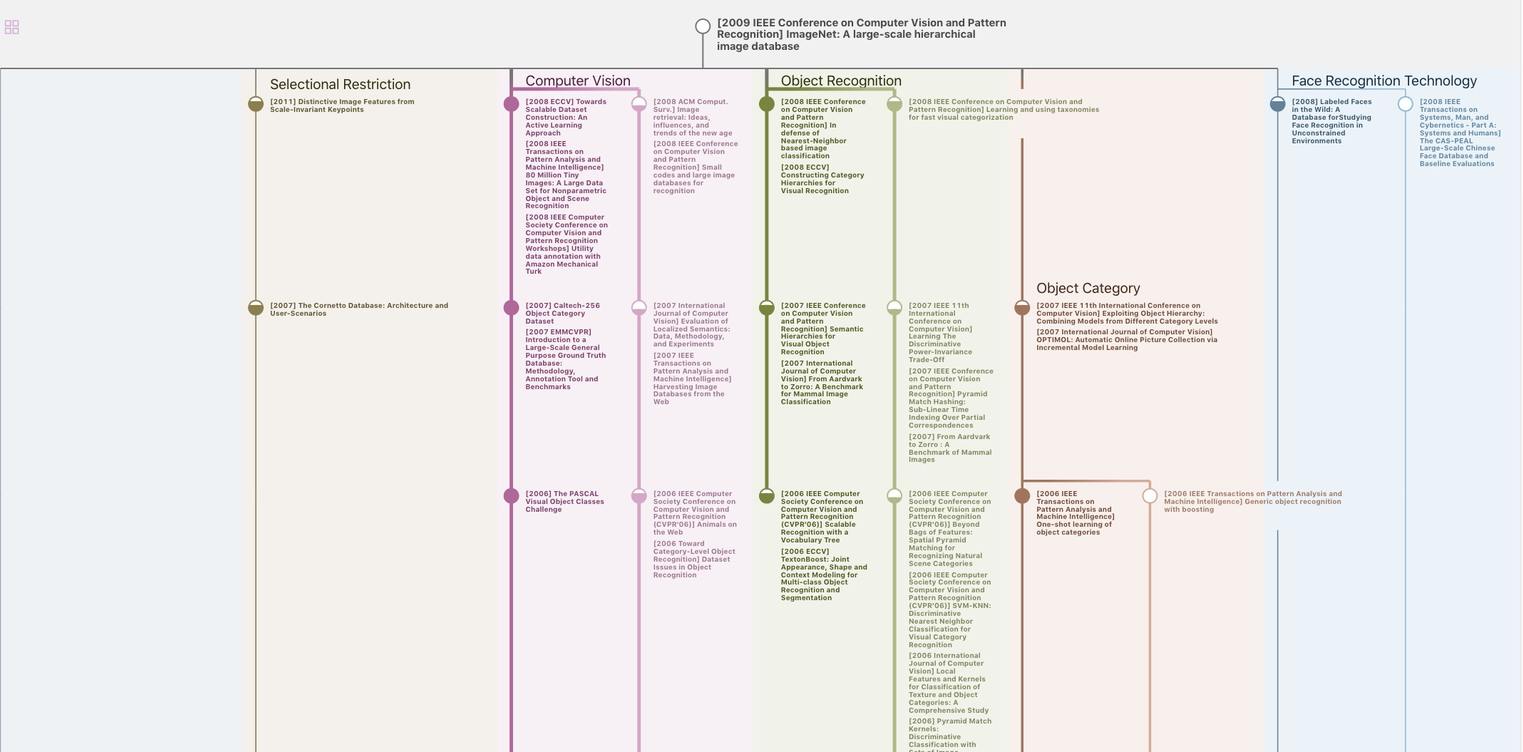
生成溯源树,研究论文发展脉络
Chat Paper
正在生成论文摘要