Short-Term Load Forecasting using Conditionally Restricted Boltzman Machine Optimized by Modified Grasshopper Optimization Algorithm.
ISGT(2023)
摘要
This paper proposes a novel hybrid model by integrating data pre-processing, feature extraction (FX), and forecasting & optimization modules to improve the accuracy and convergence rate. Where, the radial basis kernel-principal component analysis (RB-KPCA) based FX algorithm eliminates the redundant and irrelevant features to ensure the high computational efficiency. While, the modified grasshopper optimization algorithm (mGOA) optimizes the appropriate control parameters of the conditionally restricted Boltzmann machine (CRBM) to avoid the solution trapping into the local optimum and returns the results with improved accuracy. Furthermore, since the accuracy and convergence rate are two contradictory objectives, which are difficult to achieve, simultaneously. However, the the proposed FX-CRBM-mGOA forecasting model is designed in such a way to simultaneously achieve these relatively independent objectives. To evaluate the performance in terms of MAPE, accuracy and convergence rate, the proposed model is implemented on the publically available dataset of the Dayton power grid, USA. The results show that the MAPE of the proposed model is 0.4525%, the information-based artificial neural network (MIANN) is 2.4202%, long short-term memory (LSTM) is 3.231%, ANN-based accurate and fast converging (AFC-ANN) is 2.452%, and Bi-Level is 2.123%. The devised framework outperforms MI-ANN by 3.2%, Bi-level by 2.3%, and AFC-ANN by 3.1% in terms of forecast accuracy. Furthermore, the average execution time of the proposed model is 49s, the AFC-ANN model is 69s, the Bi-level model is 52s, MI-ANN model is 78s, and LSTM model is 54s.
更多查看译文
关键词
Conditionally restricted Boltzmann, Modified grasshopper optimization algorithm, Kernel principal component analysis
AI 理解论文
溯源树
样例
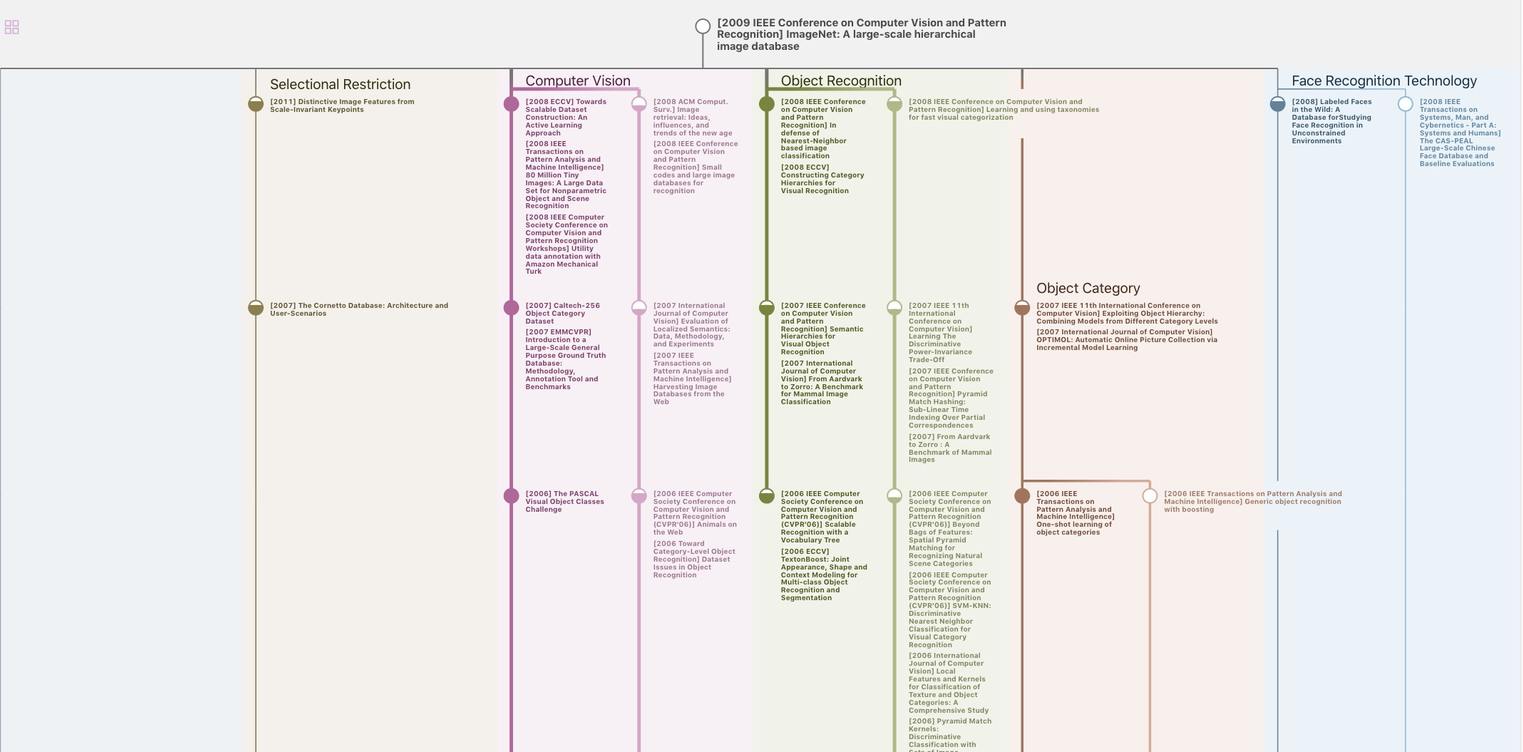
生成溯源树,研究论文发展脉络
Chat Paper
正在生成论文摘要