MMTS: Multimodal Teacher-Student learning for One-Shot Human Action Recognition
BigComp(2023)
摘要
Human action recognition (HAR) is applied to many real-world applications, such as visual surveillance, video retrieval, and autonomous driving vehicles. It can utilize various modalities such as RGB, infrared, depth, or skeleton. Among these, we selected and used a skeleton suited to real-time application because it requires less input than RGB data. Furthermore, we focused on a one-shot setting. The skeleton data tends to have a smaller dataset size than other modalities, so it is hard to expect the powerful generalization ability to make representation from unseen data (i.e. novel class). Therefore, to solve this problem, we proposed a skeleton-text multimodal learning method by borrowing a powerful pretrained text encoder that was trained using a large-scale dataset. Our method utilizes the teacher-student approach through the skeleton-text dataset and only uses the skeleton for inferences. The proposed method is more suitable for one-shot skeleton-based HAR compared to the existing multimodal learning method. Our approach outperformed the state-of-the-art methods for the one-shot action recognition protocol on the NTU RGB+D 120 dataset.
更多查看译文
关键词
human action recognition,skeleton,keypoints,one-shot,metric learning,teacher-student networks
AI 理解论文
溯源树
样例
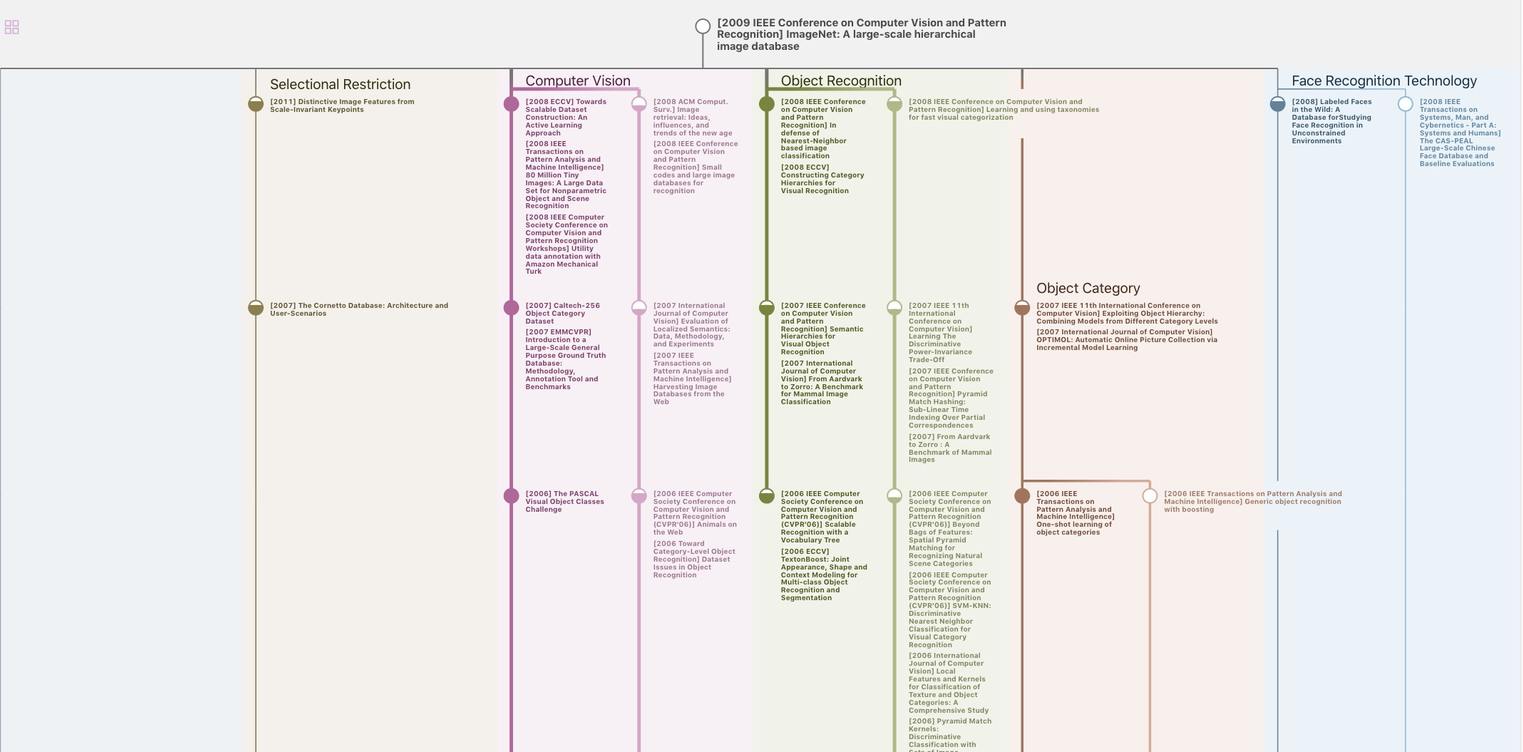
生成溯源树,研究论文发展脉络
Chat Paper
正在生成论文摘要