WSODet: A Weakly Supervised Oriented Detector for Aerial Object Detection.
IEEE Trans. Geosci. Remote. Sens.(2023)
摘要
In contrast to natural objects, aerial targets are usually non-axis aligned with arbitrary orientations. However, mainstream weakly supervised object detection (WSOD) methods can only predict horizontal bounding boxes (HBBs) from existing proposals generated by offline algorithms. To predict oriented bounding boxes (OBBs) for aerial targets while testing images end-to-end without proposals, WSODet is designed leveraging on layerwise relevance propagation (LRP) and point set representation (RepPoints). To be specific, based on the mainstream WSOD framework, LRP on multiple instance learning branch (MIL-LRP) is conducted to decrease the uncertainty and ambiguity of feature map. Then, a pseudo oriented label generation algorithm is designed to obtain OBB pseudolabels, which serve as supervision to train an oriented RepPoint Net under the guidance of improved oriented loss function (IOLF). During the test, input images are sent to oriented RepPoint branch (ORB) to obtain OBB predictions without proposals. Extensive experiments on the detection in optical remote sensing images (DIOR), Northwestern Polytechnical University (NWPU) VHR-10.v2, and HRSC2016 datasets demonstrate the effectiveness of our method to predict precise oriented aerial objects, achieving 22.2%, 46.5%, and 43.3% mAP, respectively. Moreover, training jointly with ORB boosts the results of the original WSOD framework compared with the existing WSOD methods even if there is no specific design for the original structure.
更多查看译文
关键词
Proposals,Object detection,Uncertainty,Detectors,Prediction algorithms,Feature extraction,Convolutional neural networks,Deep learning,layerwise relevance propagation (LRP),oriented object detection,point set representation (RepPoints),weakly supervised object detection (WSOD)
AI 理解论文
溯源树
样例
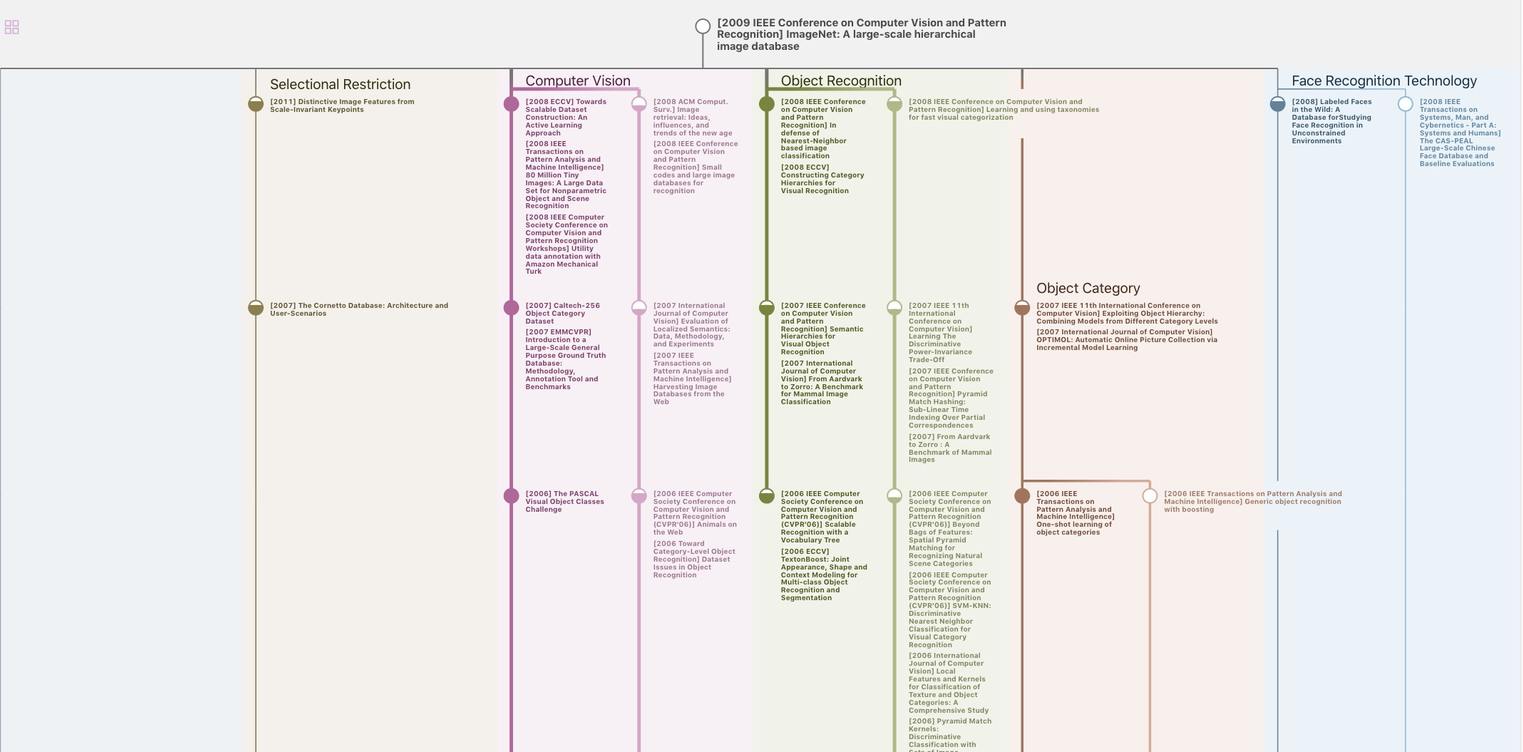
生成溯源树,研究论文发展脉络
Chat Paper
正在生成论文摘要