Conditional Cross Correlation Network for Video Question Answering.
ICSC(2023)
摘要
Video question answering (VideoQA) is the process that aims at responding to questions expressed in natural language, according to the semantic content of a given video. VideoQA is a highly challenging task and demands a comprehensive understanding of the video document, including the recognition of the various objects, actions and activities involved together with the spatial, temporal and causal relations between them. To tackle the challenge of VideoQA, most methods propose efficient techniques to fuse the representations between visual and textual modalities. In this paper, we introduce a novel framework based on a conditional cross-correlation network that learns multimodal contextualization with reduced computational and memory requirements. At the core of our approach, we consider a cross-correlation module designed to learn reciprocally constrained visual/textual features combined with a lightweight transformer that fuses the intermodal contextualization between visual and textual modalities. We test the vulnerability of the composing elements of our pipeline using black box attacks. To this purpose, we automatically generate semantic-preserving rephrased questions. The ablation study conducted confirms the importance of each module in the framework. The experimental evaluation, carried out on the MSVD-QA benchmark, validates the proposed methodology with average accuracy scores of 43.58%. When compared with state-of-the-art methods the proposed method yields gains in accuracy of more than 4%and achieves a 43.58% accuracy rate on the MSVD-QA data set.
更多查看译文
关键词
video question answering,multimodal learning,cross-correlation
AI 理解论文
溯源树
样例
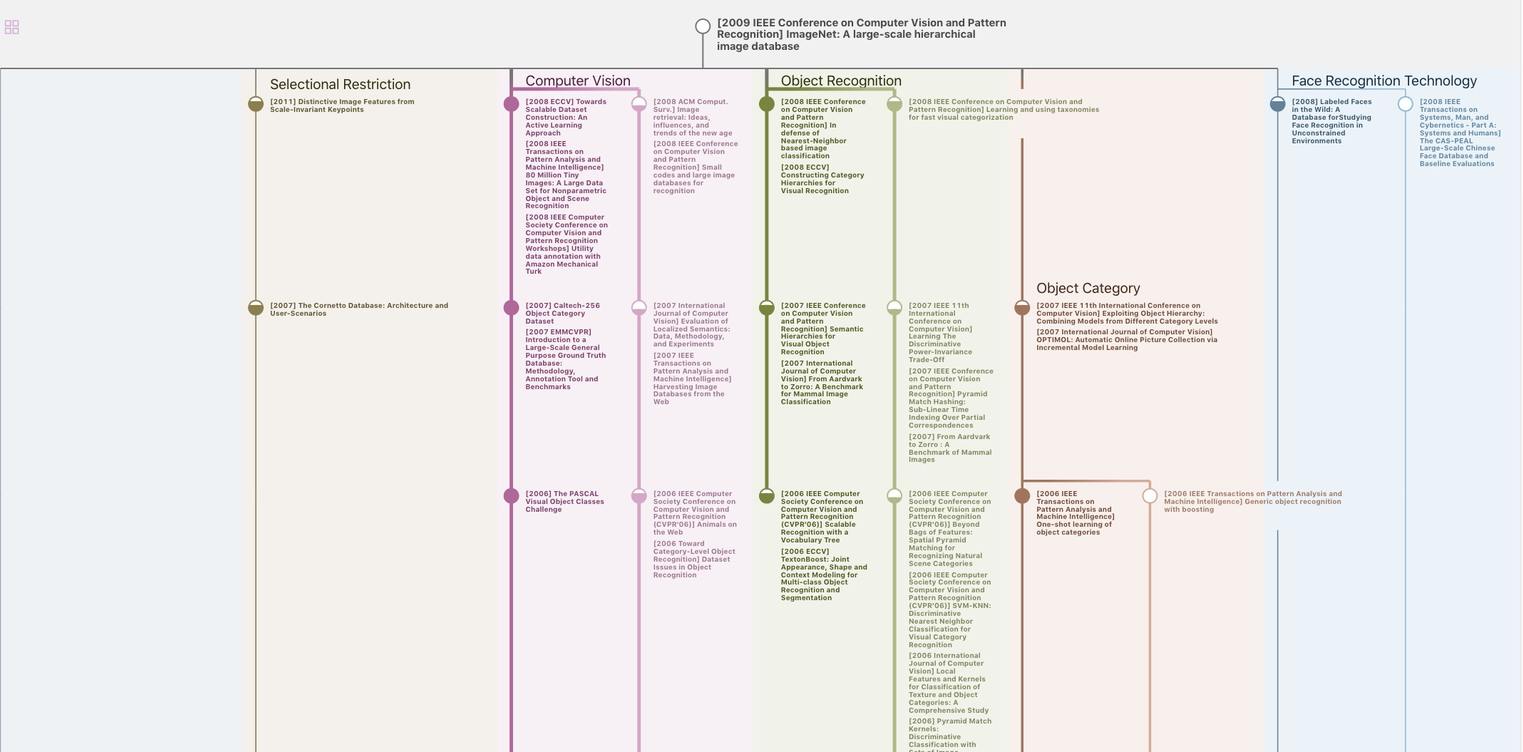
生成溯源树,研究论文发展脉络
Chat Paper
正在生成论文摘要