Injecting Temporal-Aware Knowledge in Historical Named Entity Recognition.
ECIR (1)(2023)
摘要
In this paper, we address the detection of named entities in multilingual historical collections. We argue that, besides the multiple challenges that depend on the quality of digitization (e.g., misspellings and linguistic errors), historical documents can pose another challenge due to the fact that such collections are distributed over a long enough period of time to be affected by changes and evolution of natural language. Thus, we consider that detecting entities in historical collections is time-sensitive, and explore the inclusion of temporality in the named entity recognition (NER) task by exploiting temporal knowledge graphs. More precisely, we retrieve semantically-relevant additional contexts by exploring the time information provided by historical data collections and include them as mean-pooled representations in a Transformer-based NER model. We experiment with two recent multilingual historical collections in English, French, and German, consisting of historical newspapers (19C-20C) and classical commentaries (19C). The results are promising and show the effectiveness of injecting temporal-aware knowledge into the different datasets, languages, and diverse entity types.
更多查看译文
关键词
Named entity recognition, Temporal information extraction, Digital humanities
AI 理解论文
溯源树
样例
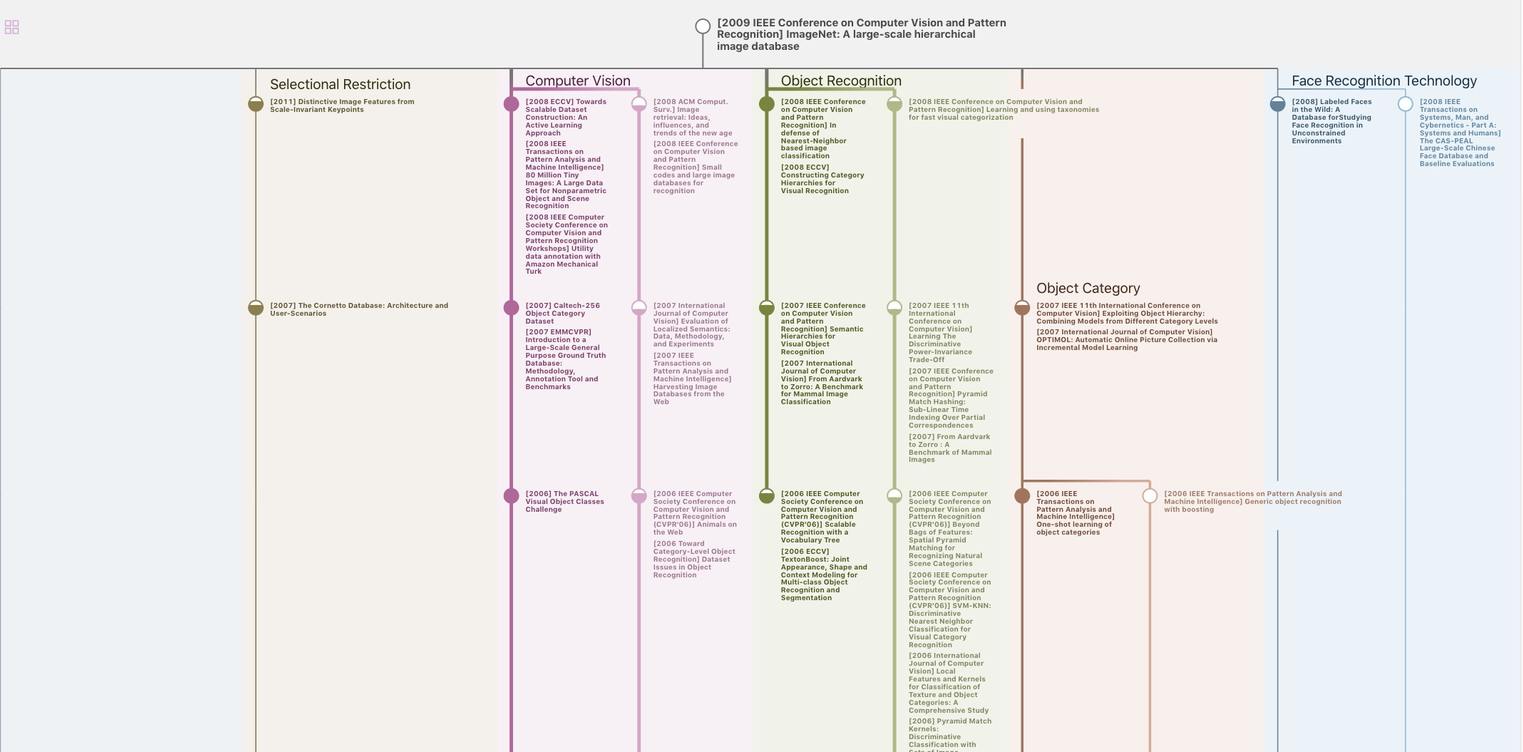
生成溯源树,研究论文发展脉络
Chat Paper
正在生成论文摘要