Novel models and algorithms for location assignment for outbound containers in container terminals.
Eur. J. Oper. Res.(2023)
摘要
In this paper, we investigate the stacking problem of outbound containers. It focuses on developing an optimal stacking strategy to allocate the arriving containers in a preassigned yard bay, with the aim of reducing extra rehandles during later retrieval. Some studies employ dynamic programming models to tackle the problem, which can offer exact solutions. Following this line of research, we propose an alter-native dynamic programming model by redefining the bay representation and adjusting the formulations accordingly. The results show that the new model strikes a good balance between stacking quality and computational time. Considering that the dynamic programming model encounters difficulty in solving large-scale instances, we design two neural network algorithms that adopt a self-attention mechanism to enhance the fitting ability of the network. The neural network algorithms learn the decision patterns from the optimal results for small-scale instances obtained by dynamic programming and then are applied to solve large-scale instances. In addition, we propose a data-driven rollout algorithm by integrating the branching strategy and the aforementioned neural network algorithms to prevent the myopic drawback of using solely the neural network. To the best of our knowledge, this is the first paper that integrates the optimization method and machine learning techniques to tackle the container stacking problem. Finally, extensive computational experiments are performed to verify the effectiveness of the proposed models and algorithms.(c) 2022 Elsevier B.V. All rights reserved.
更多查看译文
关键词
Logistics,Container stacking,Dynamic programming,Self-attention-based neural network,Rollout algorithm
AI 理解论文
溯源树
样例
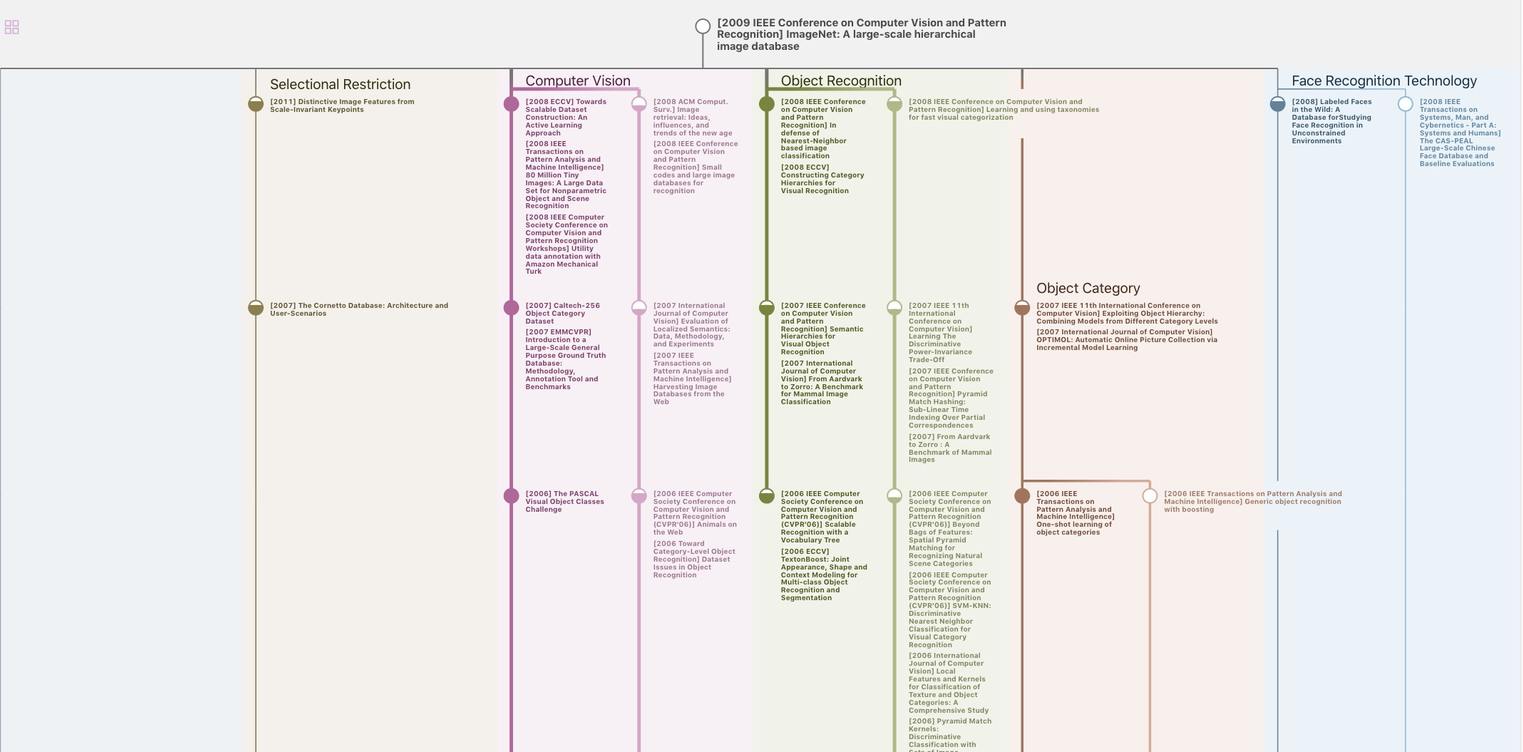
生成溯源树,研究论文发展脉络
Chat Paper
正在生成论文摘要