PVF-DectNet: Multi-modal 3D detection network based on Perspective-Voxel fusion.
Eng. Appl. Artif. Intell.(2023)
摘要
The detection of small objects such as pedestrians still poses challenges to the LiDAR-based 3D object detection due to the sparseness and disorder of point clouds. Conversely, images from cameras can provide rich semantic information, which makes these small-sized objects easy to be detected. To take use of the advantages of both devices to achieve better 3D object detection, research on the fusion of LiDAR and camera information is now being conducted. The existing fusion methods between point clouds and image are normally weighed more on the point clouds. Hence the semantic information of images is not fully utilized. We propose a new fusion method named PVFusion to try to fuse more image features. We first divide each point into a separate perspective voxel and project the voxel onto the image feature maps. Then the semantic feature of the perspective voxel is fused with the geometric feature of the point. A 3D object detection model (PVF-DectNet) is designed using PVFusion. During training we employ the ground truth paste (GT-Paste) data augmentation and solve the occlusion problem caused by newly added object. The KITTI validation set is used to validate the PVF-DectNet, which shows 3.6% AP improvement over the other feature fusion methods in pedestrian detection. On the KITTI test set, the PVF-DectNet outperforms the other multi-modal SOTA methods by 2.2% AP in pedestrian detection. And PVFusion shows better detection performance for sparse point clouds than PointFusion in both car and pedestrian categories. As for 32 beams LiDAR scene, there are 4.2% AP increment in moderate difficulty car category and 5.2% mAP improvement in pedestrian category.
更多查看译文
关键词
3D object detection,Sensor fusion,Deep learning,Small objects,LiDAR-camera-based detector
AI 理解论文
溯源树
样例
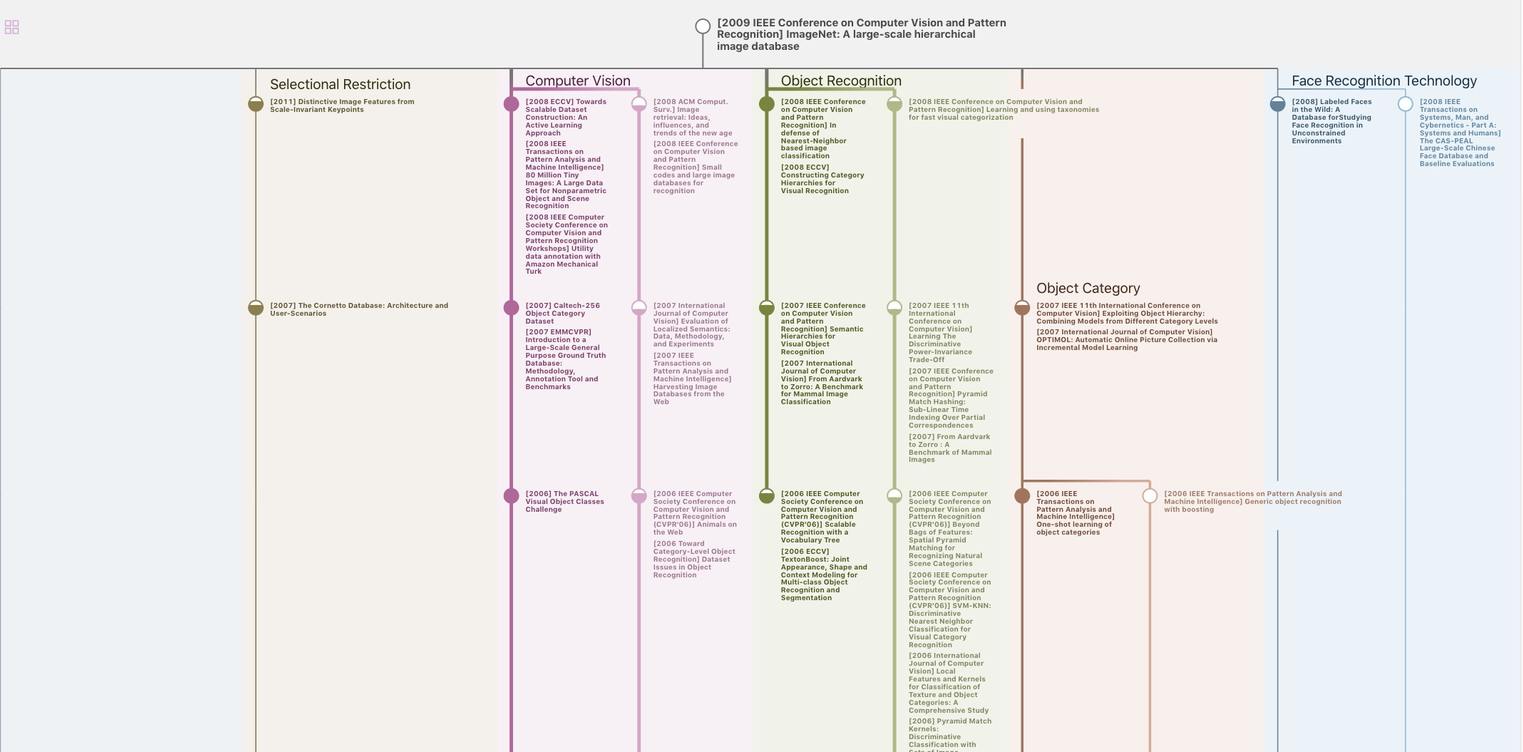
生成溯源树,研究论文发展脉络
Chat Paper
正在生成论文摘要