Reinforcement learning for energy efficiency improvement in UAV-BS access networks: A knowledge transfer scheme.
Eng. Appl. Artif. Intell.(2023)
摘要
Recently the possibility of forming unmanned aerial vehicle base station (UAV-BS) network systems with energy harvesting capabilities to support persistent wireless access services for pedestrian users has been validated. Due to the need of sustaining wireless access services of the UAV-BSs, we investigate an optimal policy to maximize the overall energy utilization efficiency (renewable energy) of the UAV-BSs during their active in-flight network access operations. Since the natural sources of renewable energy (e.g., solar energy or wind energy harvesting) have stochastic properties with respect to the arrival rate of the dynamics of the unknown environment, we exploit an actor–critic reinforcement learning framework, which considers the continuous-valued states and action space for learning the best policy during interaction with the environment. To enhance and expedite the learning process, a transfer asynchronous advantage actor–critic (TA3C) algorithm is proposed, which enables UAV-BSs to transfer (i.e., share) knowledge gained in historical periods, during parallel task asynchronous executions on multiple instances of the environment. Numerical results reveal that the proposed TA3C algorithm surpasses the classic A3C and A2C algorithms in terms of throughput and optimal energy utilization efficiency.
更多查看译文
关键词
Algorithm,Energy harvesting,NP-hard,Resource management,Reinforcement learning,UAV
AI 理解论文
溯源树
样例
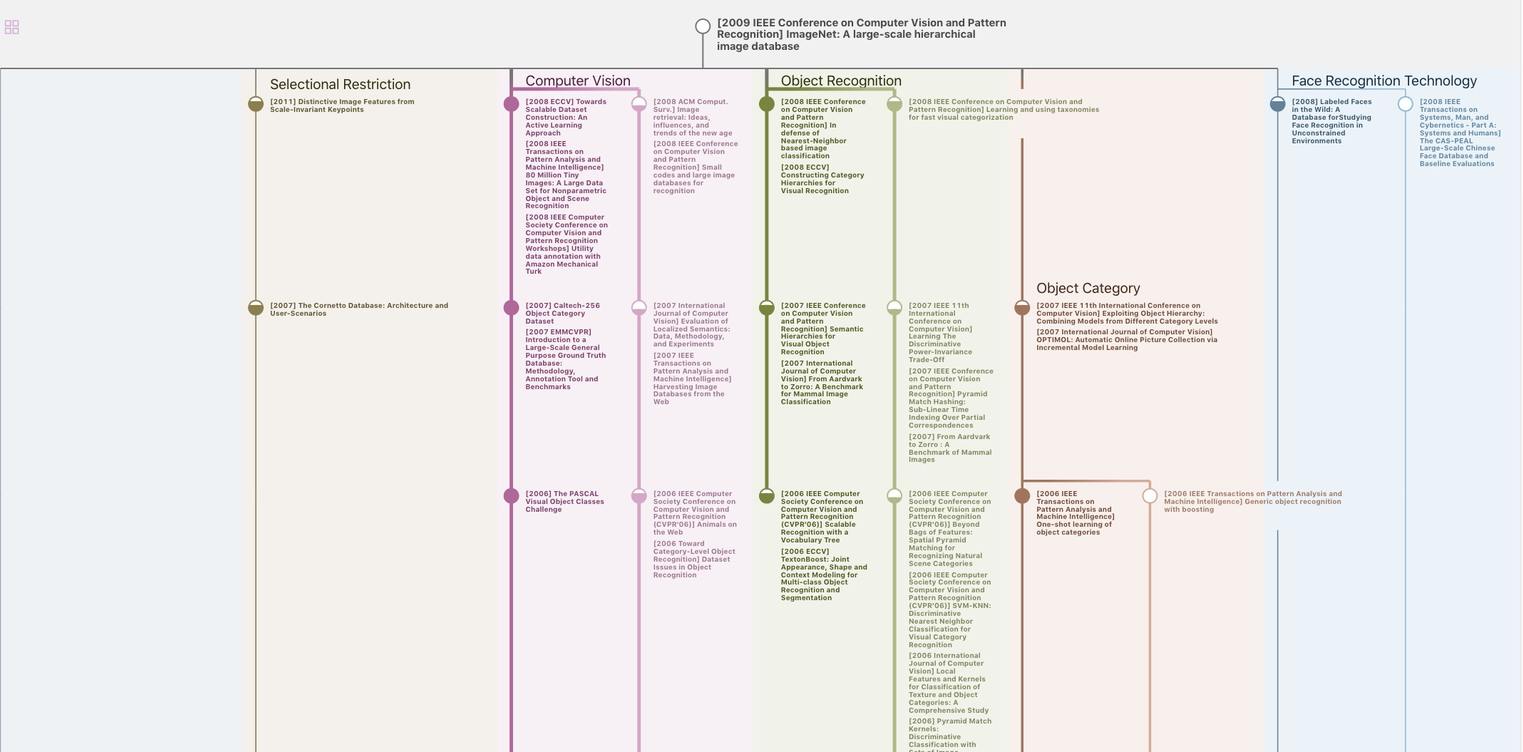
生成溯源树,研究论文发展脉络
Chat Paper
正在生成论文摘要