Multi-Modal Multi-Stage Underwater Side-Scan Sonar Target Recognition Based on Synthetic Images.
Remote. Sens.(2023)
摘要
Due to the small sample size of underwater acoustic data and the strong noise interference caused by seabed reverberation, recognizing underwater targets in Side-Scan Sonar (SSS) images is challenging. Using a transfer-learning-based recognition method to train the backbone network on a large optical dataset (ImageNet) and fine-tuning the head network with a small SSS image dataset can improve the classification of sonar images. However, optical and sonar images have different statistical characteristics, directly affecting transfer-learning-based target recognition. In order to improve the accuracy of underwater sonar image classification, a style transformation method between optical and SSS images is proposed in this study. In the proposed method, objects with the SSS style were synthesized through content image feature extraction and image style transfer to reduce the variability of different data sources. A staged optimization strategy using multi-modal data effectively captures the anti-noise features of sonar images, providing a new learning method for transfer learning. The results of the classification experiment showed that the approach is more stable when using synthetic data and other multi-modal datasets, with an overall accuracy of 100%.
更多查看译文
关键词
side-scan sonar image classification,whitening and style transfer,multi-modal transfer learning,staged optimization,feature representation
AI 理解论文
溯源树
样例
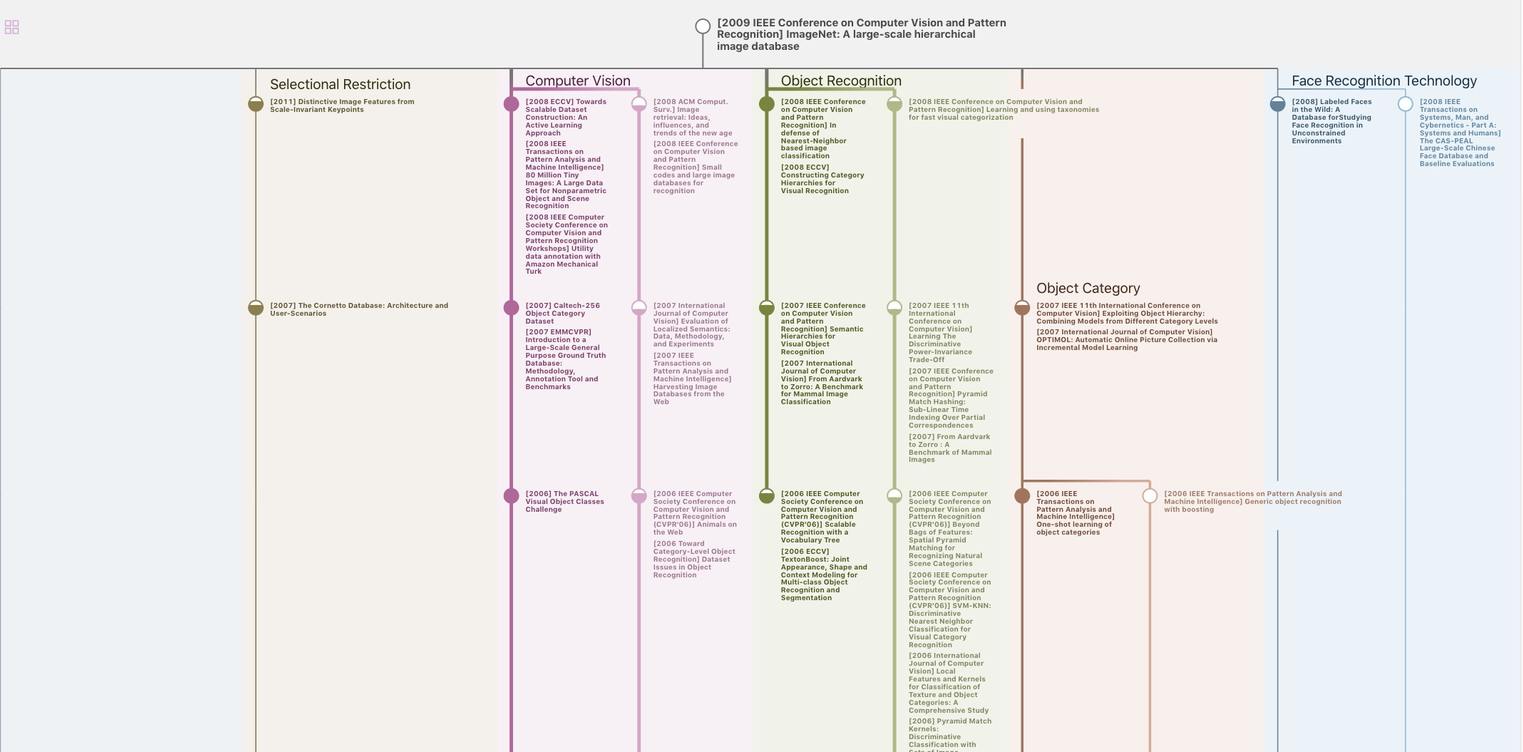
生成溯源树,研究论文发展脉络
Chat Paper
正在生成论文摘要