CMG: A Class-Mixed Generation Approach to Out-of-Distribution Detection.
ECML/PKDD (4)(2022)
摘要
Recently, contrastive learning with data and class augmentations has been shown to produce markedly better results for outof-distribution (OOD) detection than previous approaches. However, a major shortcoming of this approach is that it is extremely slow due to the significant increase in data size and in the number of classes and the quadratic pairwise similarity computation. This paper shows that this heavy machinery is unnecessary. A novel approach, called CMG (ClassMixed Generation), is proposed, which generates pseudo-OOD data by mixing class embeddings as abnormal conditions to CVAE (conditional variational Auto-Encoder) and then uses the data to fine-tune a classifier built using the given in-distribution (IND) data. To our surprise, the obvious approach of using the IND data and the pseudo-OOD data to directly train an OOD model is a very poor choice. The fine-tuning based approach turns out to be markedly better. Empirical evaluation shows that CMG not only produces new state-of-the-art results but also is much more efficient than contrastive learning, at least 10 times faster (Code is available at: https://github.com/shaoyijia/CMG).
更多查看译文
关键词
Out-of-distribution detection, Data generation
AI 理解论文
溯源树
样例
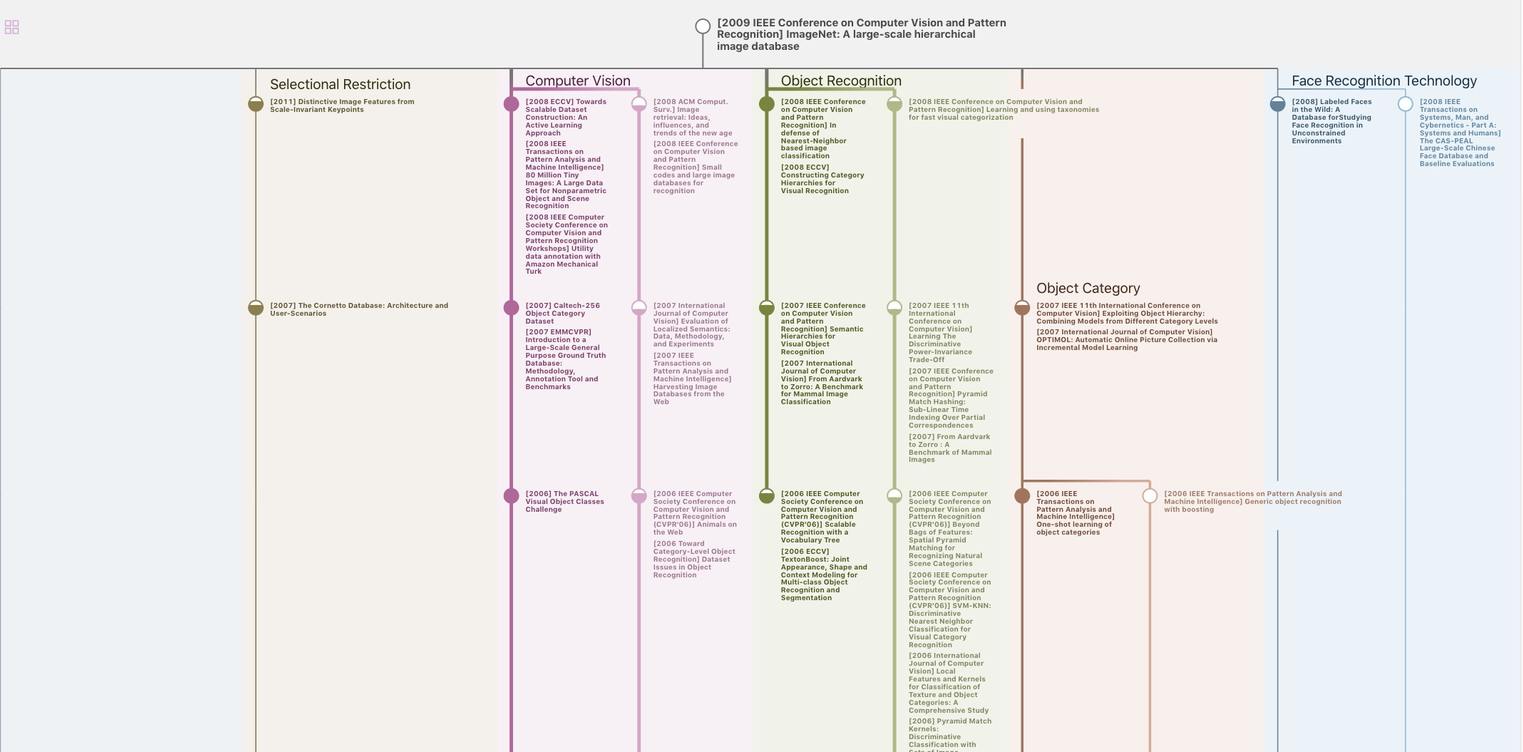
生成溯源树,研究论文发展脉络
Chat Paper
正在生成论文摘要