Region-of-interest Attentive Heteromodal Variational Encoder-Decoder for Segmentation with Missing Modalities.
ACCV (6)(2022)
摘要
The use of multimodal images generally improves segmentation. However, complete multimodal datasets are often unavailable due to clinical constraints. To address this problem, we propose a novel multimodal segmentation framework that is robust to missing modalities by using a region-of-interest (ROI) attentive modality completion. We use ROI attentive skip connection to focus on segmentation-related regions and a joint discriminator that combines tumor ROI attentive images and segmentation probability maps to learn segmentation-relevant shared latent representations. Our method is validated in the brain tumor segmentation challenge dataset of 285 cases for the three regions of the complete tumor, tumor core, and enhancing tumor. It is also validated on the ischemic stroke lesion segmentation challenge dataset with 28 cases of infarction lesions. Our method outperforms state-of-the-art methods in robust multimodal segmentation, achieving an average Dice of 84.15
$$\%$$
, 75.59
$$\%$$
, and 54.90
$$\%$$
for the three types of brain tumor regions, respectively, and 48.29
$$\%$$
for stroke lesions. Our method can improve the clinical workflow that requires multimodal images.
更多查看译文
关键词
Segmentation, Missing modalities, Multimodal learning, Adversarial learning
AI 理解论文
溯源树
样例
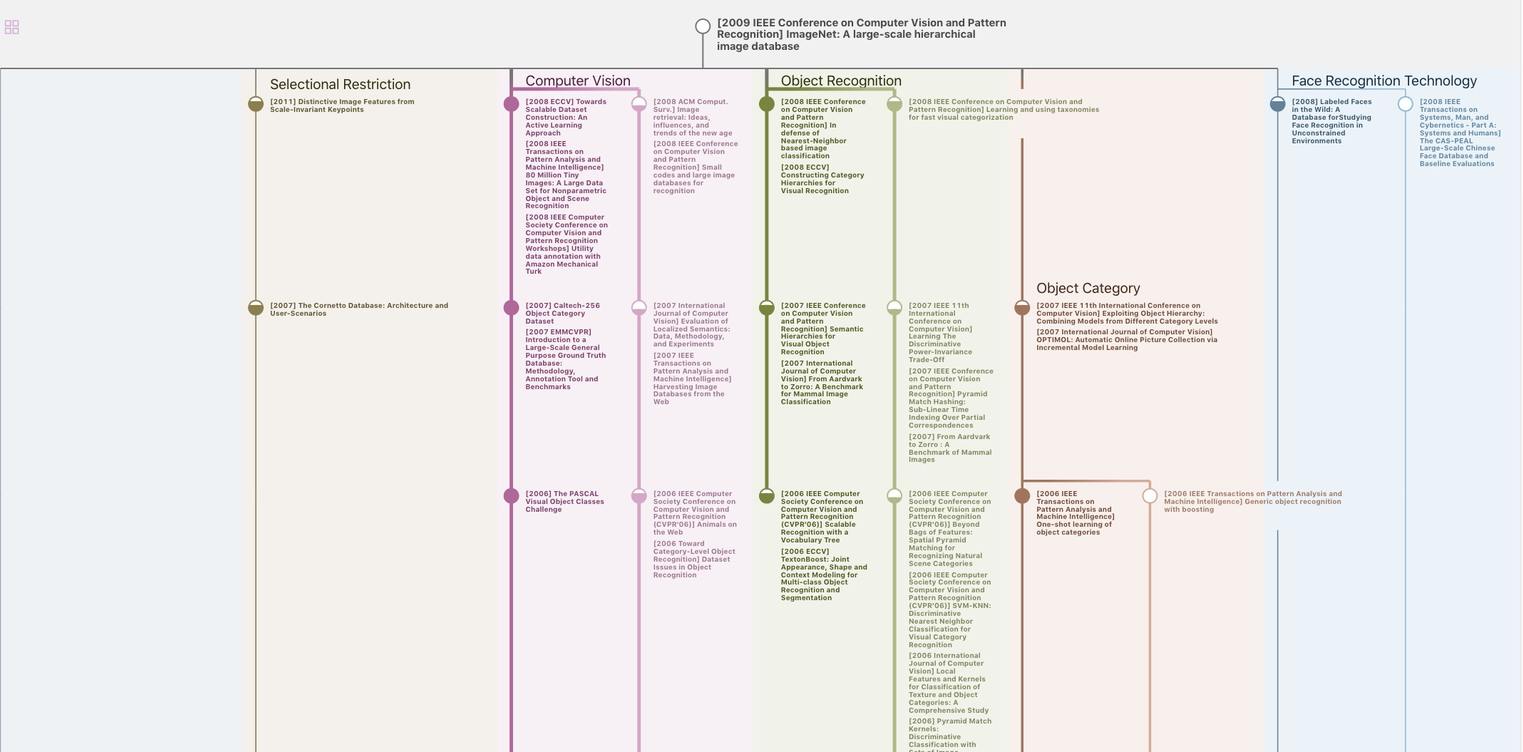
生成溯源树,研究论文发展脉络
Chat Paper
正在生成论文摘要