An EffcientNet-encoder U-Net Joint Residual Refinement Module with Tversky-Kahneman Baroni-Urbani-Buser loss for biomedical image Segmentation.
Biomed. Signal Process. Control.(2023)
摘要
Quantitative analysis on biomedical images has been on increasing demand nowadays and for modern computer vision approaches. While recently advanced procedures have been enforced, there is still necessity in optimizing network architecture and loss functions. Inspired by the pretrained EfficientNet-B4 and the refinement module in boundary-aware problems, we propose a new two-stage network which is called EffcientNet-encoder U-Net Joint Residual Refinement Module and we create a novel loss function called the Tversky-Kahneman Baroni-Urbani-Buser loss function. The loss function is built on the basement of the Baroni-Urbani-Buser coefficient and the Jaccard-Tanimoto coefficient and reformulated in the Tversky- Kahneman probability-weighting function. We have evaluated our algorithm on the four popular datasets: the 2018 Data Science Bowl Cell Nucleus Segmentation dataset, the Brain Tumor LGG Segmentation dataset, the Skin Lesion ISIC 2018 dataset and the MRI cardiac ACDC dataset. Several comparisons have proved that our proposed approach is noticeably promising and some of the segmentation results provide new state-of-the-art results. The code is available at https://github.com/tswizzle141/An-EffcientNet-encoder-U-Net-Joint-Residual-Refinement-Module-with-TK-BUB-Loss.
更多查看译文
关键词
Biomedical Image Segmentation,EfficientNet,Residual Refinement Module,Baroni-Urbani-Buser coefficient,Tversky-Kahneman probability-weighting ,function
AI 理解论文
溯源树
样例
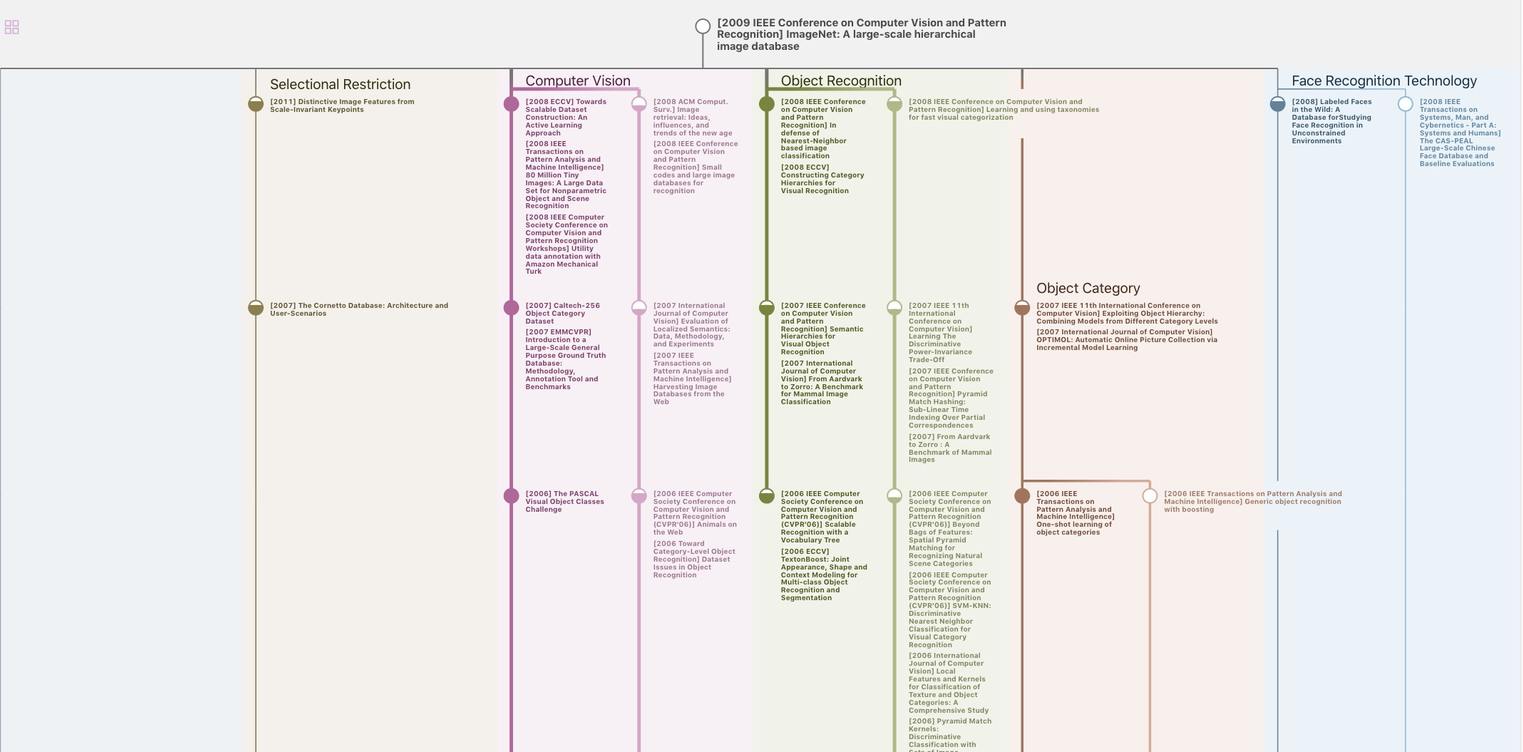
生成溯源树,研究论文发展脉络
Chat Paper
正在生成论文摘要