A Novel Transmission Line Defect Detection Method Based on Adaptive Federated Learning.
IEEE Trans. Instrum. Meas.(2023)
摘要
The existing transmission line detection technologies based on cloud computing are faced with the problems such as low response speed, high communication cost, and difficulty in obtaining scattered data. This article proposes a transmission line defect detection method based on adaptive federated learning (FL), which can adaptively change the data volume of the next training round according to the training energy consumption of each round. As a result, the proposed method can achieve the optimal number of communication between local training cost and global communication efficiency, which greatly improves the training speed and reduces the communication cost. Furthermore, a lightweight Mask region-convolutional neural network (R-CNN) that adopts the MobileNet architecture to replace the original network is designed for the edge, which reduces the required number of parameters and improves the detection speed. The experimental results show that the model training efficiency of the proposed adaptive FL is 70% higher than the centralized cloud computing, and the computing cost is saved by about 40%. Compared with the original Mask R-CNN, the proposed lightweight Mask R-CNN model can achieve 1.1% higher precision, 10% shorter training time, and 40% faster detection speed.
更多查看译文
关键词
Computational modeling,Image edge detection,Adaptation models,Training,Performance evaluation,Edge computing,Data models,Adaptive algorithm,deep learning,defect detection,edge computing,federated learning (FL)
AI 理解论文
溯源树
样例
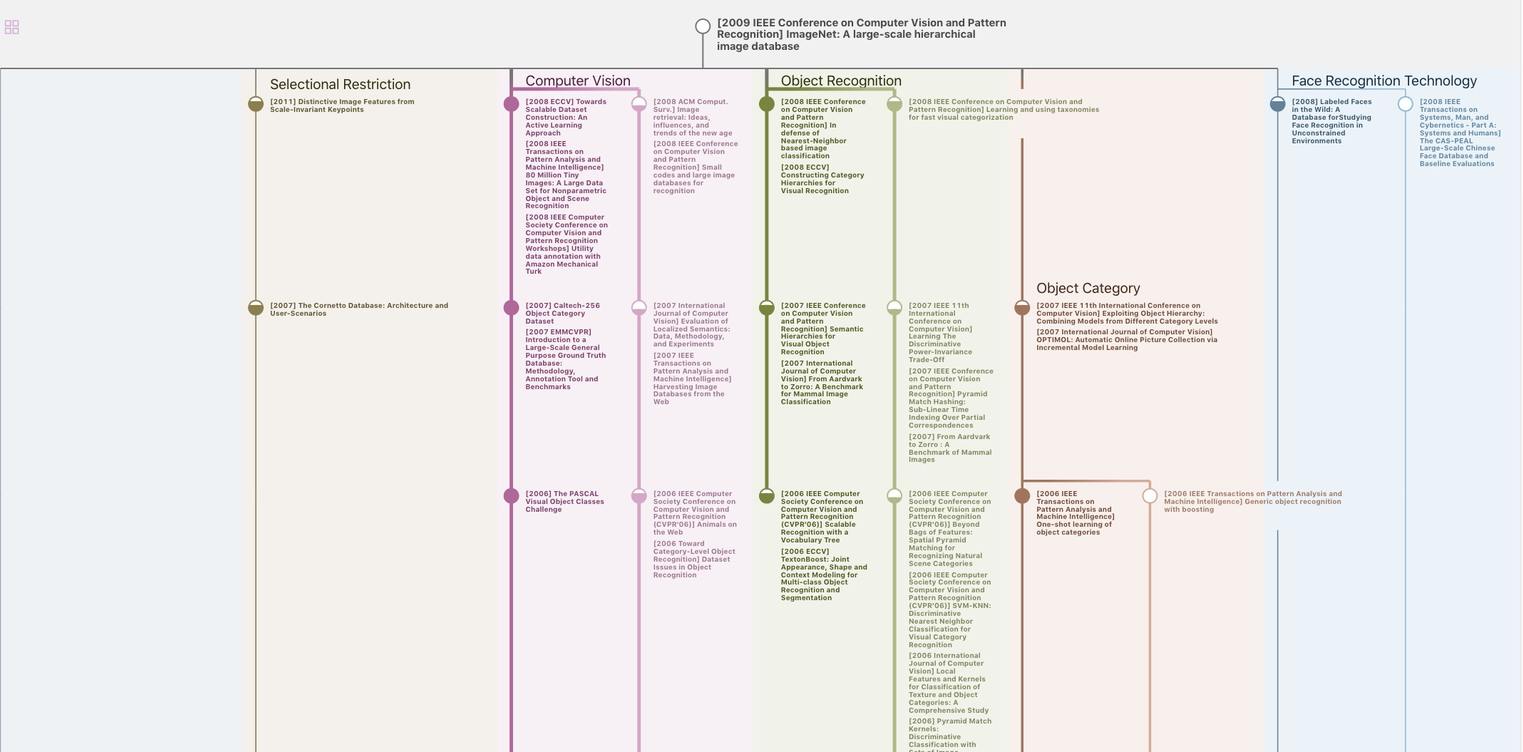
生成溯源树,研究论文发展脉络
Chat Paper
正在生成论文摘要