Classification of Leftovers from the Stock Cutting Process.
OL2A(2022)
摘要
The one-dimensional cutting stock problem considers only one dimension in the cutting process and consists of cutting a set of objects available in stock to produce the desired items in specified quantities and sizes. Therefore, the cutting process can generate leftovers - which can be reused in a new demand - or losses, which are discarded. In this context, the objective of this work is to present a methodology for generating a numerical data set, considering items demand data and cut objects, for the problem of classifying leftovers or losses from the cutting stock process. The paper presents an algorithm to generate such a set and its evaluation using Machine Learning methods, as Logistic Regression, Naive Bayes, Decision Trees and Random Forests. These methods are trained and validated using statistical measures. The provided dataset is available to be used in supervised training algorithms for classification tasks. Results show the performance of the Machine Learning methods, which are evaluated using stratified k-fold cross validation and specific statistical measures. Since the analysis indicate good performance, we can also conclude that the generated data set is robust and it can be used in other classification tasks.
更多查看译文
关键词
Cutting stock problem, Machine learning, Statistical measures, Classification task
AI 理解论文
溯源树
样例
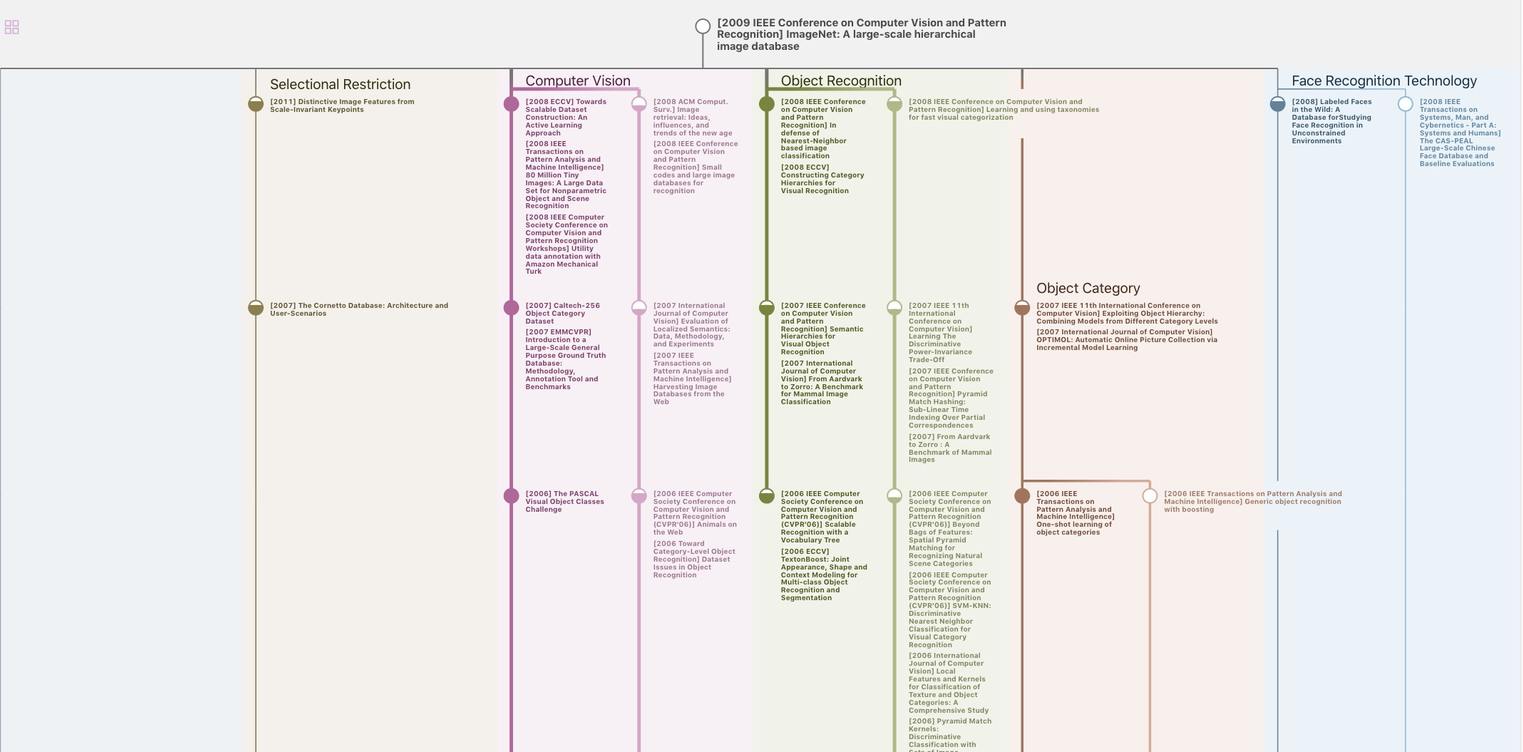
生成溯源树,研究论文发展脉络
Chat Paper
正在生成论文摘要